Parallel Deep Learning Ensembles For Human Pose Estimation
PROCEEDINGS OF THE ASME 11TH ANNUAL DYNAMIC SYSTEMS AND CONTROL CONFERENCE, 2018, VOL 1(2018)
摘要
This paper presents an efficient method to detect human pose with monocular color imagery using a parallel architecture based on deep neural network. The network presented in this approach consists of two sequentially connected stages of 13 parallel CNN ensembles, where each ensemble is trained to detect one specific kind of linkage of the human skeleton structure. After detecting all skeleton linkages, a voting score based post-processing algorithm assembles the individual linkages to form a complete human structure. This algorithm exploits human structural heuristics while assembling skeleton links and searches only for adjacent link pairs around the expected common joint area. The use of structural heuristics in the presented approach heavily simplifies the post-processing computations. Furthermore, the parallel architecture of the presented network enables mutually independent computing nodes to be efficiently deployed on parallel computing devices such as GPUs for computationally efficient training. The proposed network has been trained and tested on the COCO 2017 person-keypoints dataset and delivers pose estimation performance matching state-of-art networks. The parallel ensembles architecture improves its adaptability in applications aimed at identifying only specific body parts while saving computational resources.
更多查看译文
关键词
Pose Estimation, Convolutional Neural Networks (CNN), Linkage-based Approach, Parallel CNN Architecture.
AI 理解论文
溯源树
样例
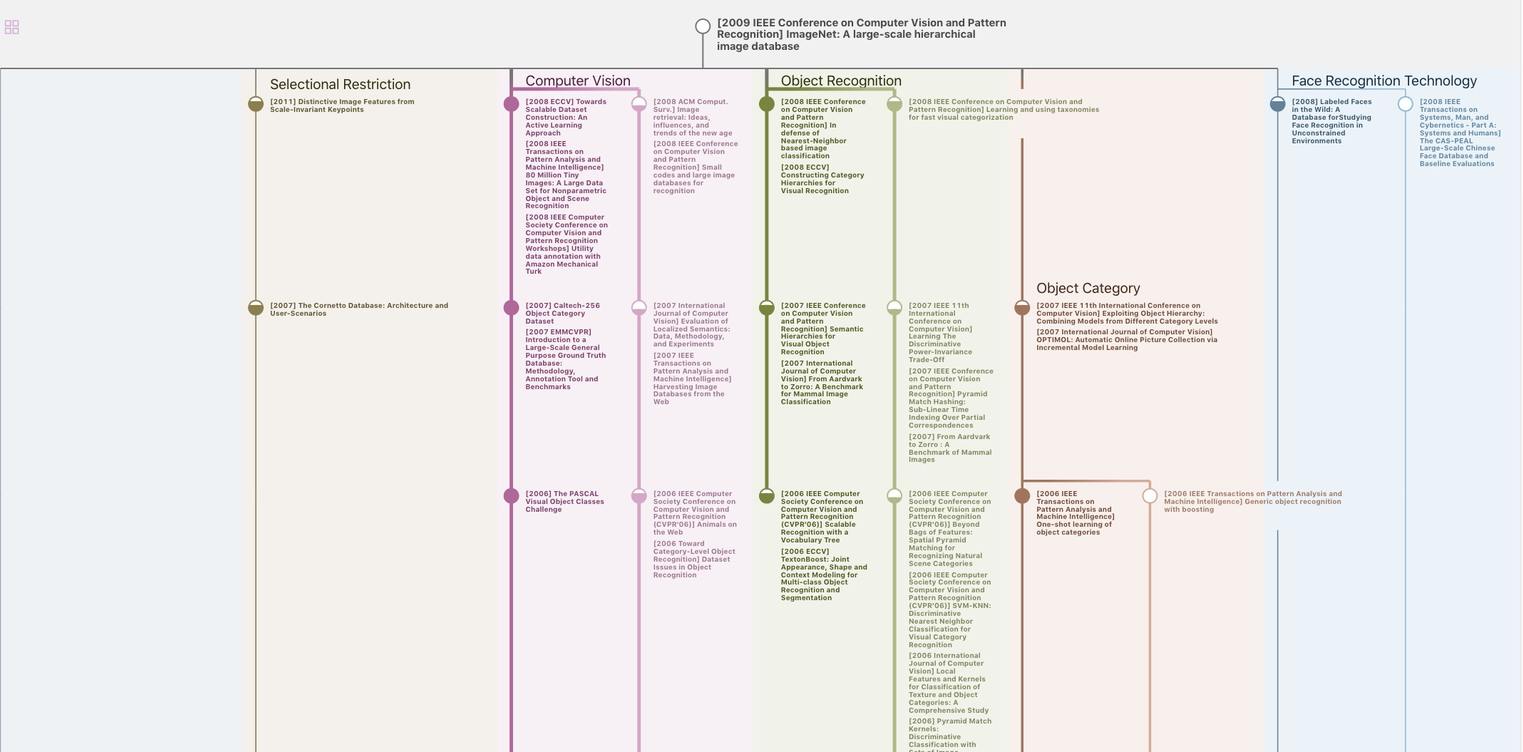
生成溯源树,研究论文发展脉络
Chat Paper
正在生成论文摘要