Novelty-Driven Recommendation By Using Integrated Matrix Factorization And Temporal-Aware Clustering Optimization
INTERNATIONAL JOURNAL OF COMMUNICATION SYSTEMS(2020)
摘要
With the rapid proliferation of information and communication technology (ICT), the vast amount of available data creates information overload. The Websites and e-commerce applications employ several information filtering methods such as personalized recommender system to manage the information overload. The recommender system assists the users in obtaining the desired list of products based on their interest. Several existing research works focus on the novelty or unexpectedness in the recommendation list while ensuring the quality to enhance the recommendation mechanism. It is essential to balance the unexpected and useful products or services to generate the satisfactory personalized recommendations with novelty. Thus, this paper proposes a novelty-driven movie suggestion using integrated matrix factorization and temporal-aware clustering optimization (NOMINATE). The proposed approach determines the personalized preferences through probabilistic matrix factorization (PMF) and contextually updates the rules and extracts the user preferences based on the inherent features of both the users and movies with temporal information. The NOMINATE approach also suggests the novelty-driven, and desired top-N movies to the users through the K-means, and particle swarm optimization (PSO)-based clustering algorithm with the help of LOD source. To identify the expert users, the NOMINATE approach applies the K-means and PSO-based clustering algorithm to enrich the personalized features of the users. Moreover, it integrates the relevant features with the preferred set of features for each user using the LOD source and decides a set of optimal preferences of the users. Finally, the NOMINATE approach generates the top-N recommendation list for the corresponding user through ranking method. The experiment results stipulate that the NOMINATE approach personalize the top-N movie recommendations with high performance regarding accuracy and novelty when compared with the existing recommendation method.
更多查看译文
关键词
features, K-means, LOD, novelty, PMF, PSO, recommender system, top-N recommendation
AI 理解论文
溯源树
样例
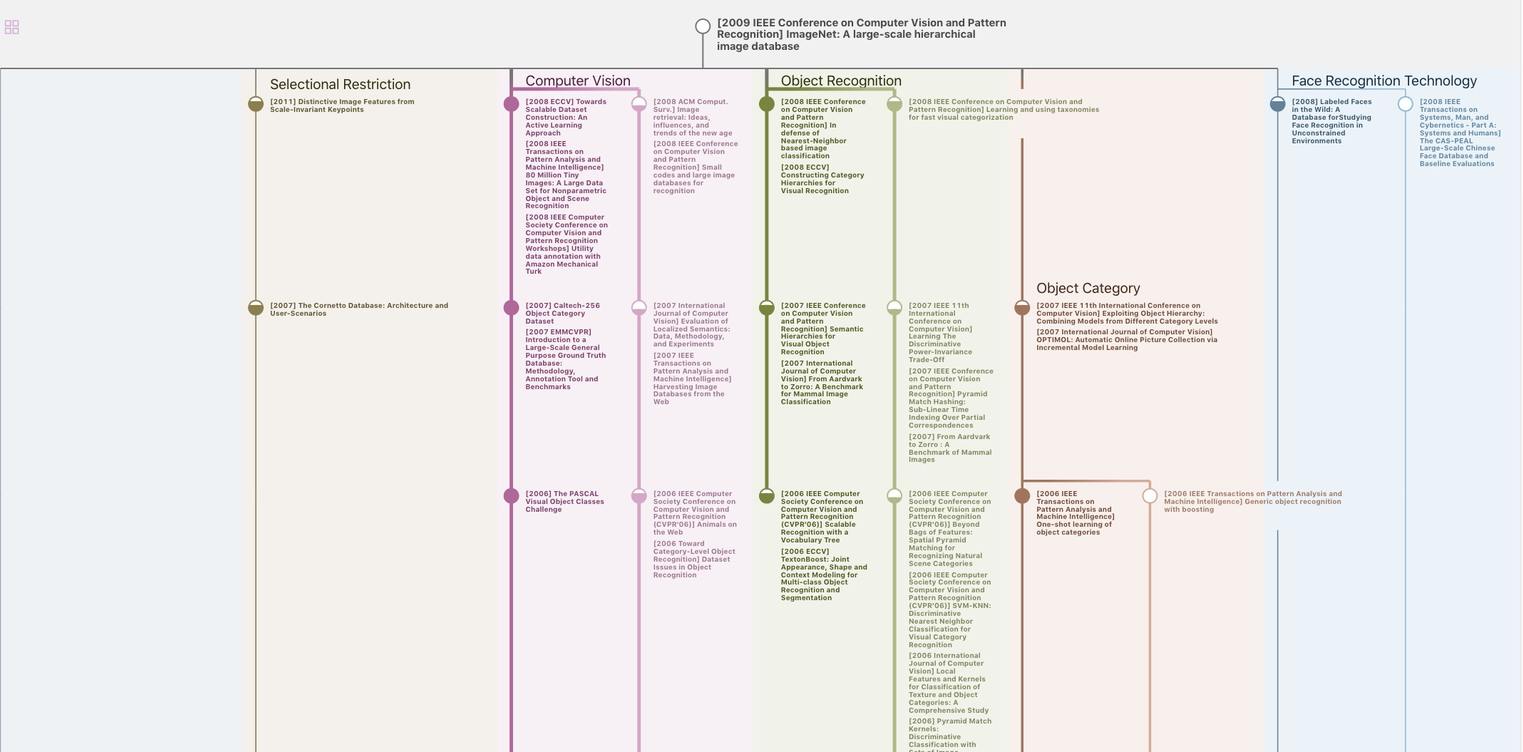
生成溯源树,研究论文发展脉络
Chat Paper
正在生成论文摘要