Tracking Cluster Transitions Using Summaries
2018 International Conference on Data Science and Engineering (ICDSE)(2018)
摘要
Clustering is an important task in data mining, for understanding the natural grouping or partitions in a dataset. It is not surprising that clustering finds application in data stream mining as well. Distribution of the data is expected to be dynamically varying in data streams. This leads to the dynamic changes in the natural partitions. Hence the number of clusters, shape and size of individual clusters etc. are subject to dynamic variations. Understanding the nature of cluster transitions is useful in many fields such as customer relationship management, fraud discovery, healthcare systems etc. It will give hint on current trends, split or merge of groups, and shifting interests in that particular domain. Cluster transition modelling is done mostly by using point-wise computations. Here we propose a model which represents the clusters by their summary and do summary-wise computations to identify the transition. Also, upcoming cluster transitions are predicted using time series prediction methods.
更多查看译文
关键词
Data streams,Clustering,Cluster transition
AI 理解论文
溯源树
样例
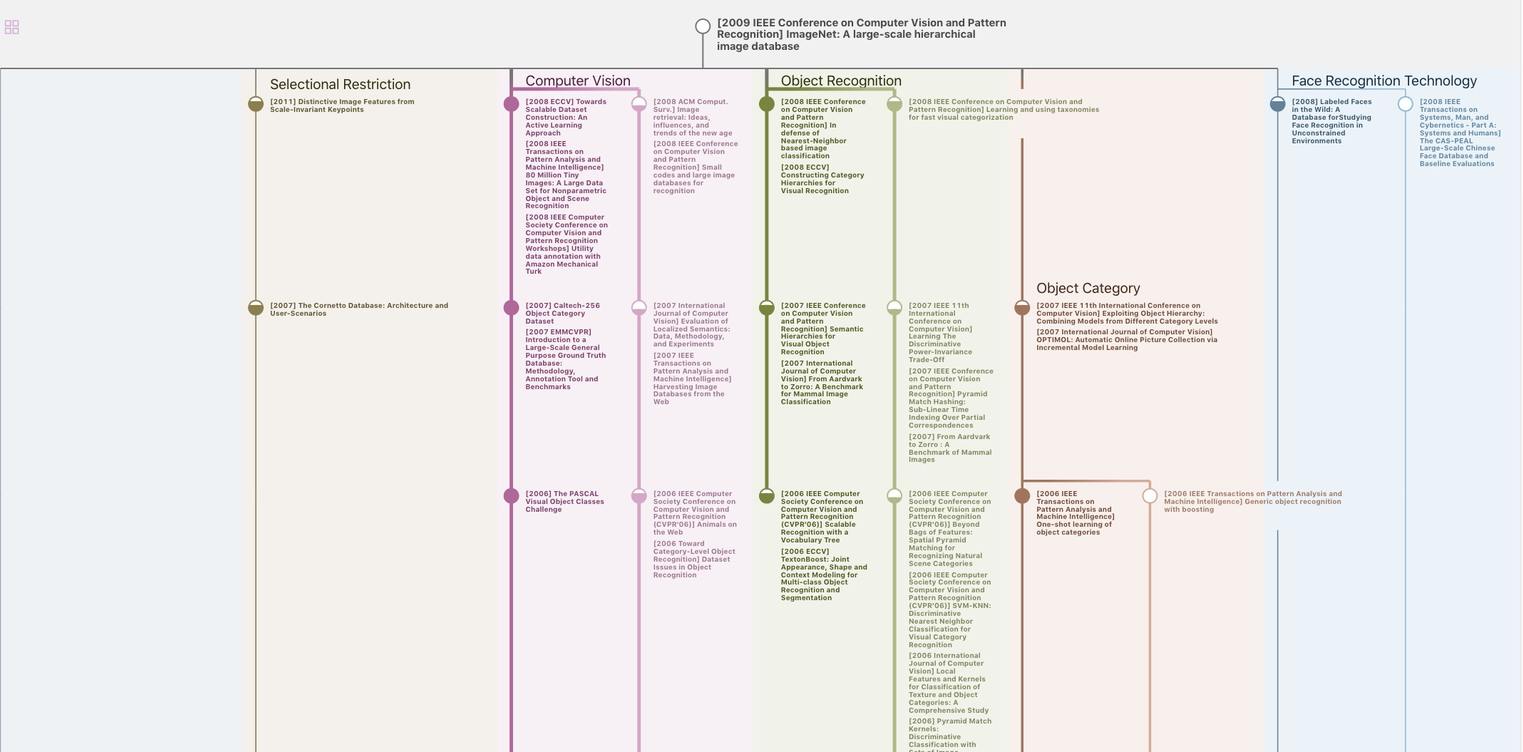
生成溯源树,研究论文发展脉络
Chat Paper
正在生成论文摘要