Characterizing Combustion Instability Using Deep Convolutional Neural Network
PROCEEDINGS OF THE ASME 11TH ANNUAL DYNAMIC SYSTEMS AND CONTROL CONFERENCE, 2018, VOL 1(2018)
摘要
Detecting the transition to an impending instability is important to initiate effective control in a combustion system. As one of the early applications of characterizing thermoacoustic instability using Deep Neural Networks, we train our proposed deep convolutional neural network (CNN) model on sequential image frames extracted from hi-speed flame videos by inducing instability in the system following a particular protocol-varying the acoustic length. We leverage the sound pressure data to define a non-dimensional instability measure used for applying an inexpensive but noisy labeling technique to train our supervised 2D CNN model. We attempt to detect the onset of instability in a transient dataset where instability is induced by a different protocol. With the continuous variation of the control parameter, we can successfully detect the critical transition to a state of high combustion instability demonstrating the robustness of our proposed detection framework, which is independent of the combustion inducing protocol.
更多查看译文
AI 理解论文
溯源树
样例
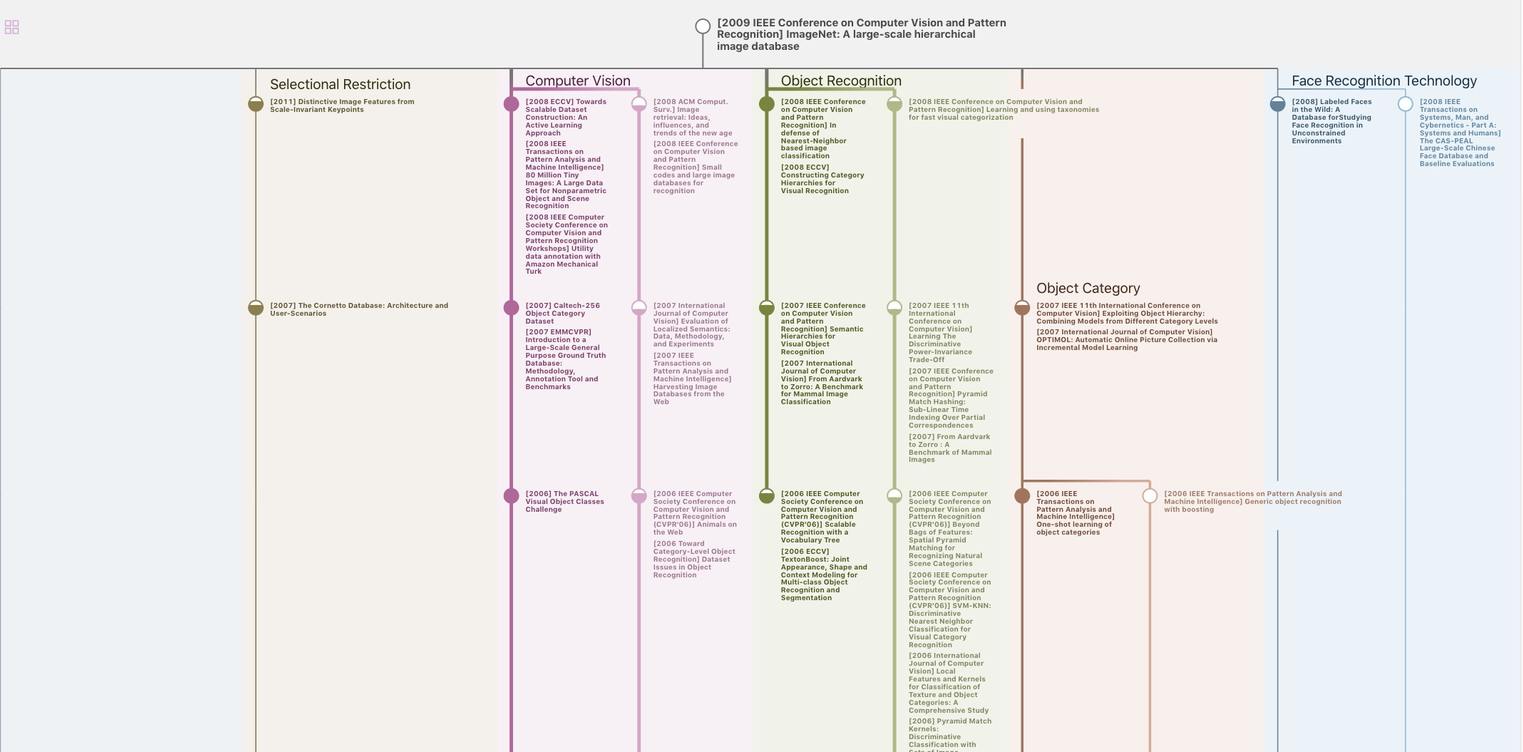
生成溯源树,研究论文发展脉络
Chat Paper
正在生成论文摘要