Missing Information Management for Massive Sparse Data
2018 IEEE 4th International Conference on Big Data Security on Cloud (BigDataSecurity), IEEE International Conference on High Performance and Smart Computing, (HPSC) and IEEE International Conference on Intelligent Data and Security (IDS)(2018)
摘要
Finding out the method of handling the missing information is essential for system efficiency and robustness in the field of the database. The sparsity of massive data in the big data environment makes the problem of missing information more prominent. The existing methods either have limited semantic expression ability or do not consider the influence of big data environment. Missing information in large-scale sparse data tends to have richer semantics, leading to more complex computational logic, as well as affecting operations such as data queries. To solve these problems, this paper proposes a novel missing information management method of logic operation definition and relational algebra expansion. Combining the practical case of big data environment, we summarize the missing information into two types: unknown value and nonexistent value, and define four-valued logic to support the logic operation. Based on the dynamic table model, we systematically extend the relational algebra to describe the data operations for massive sparse data. Our method is implemented in the self-developed big data management system Muldas. Experimental results on real large-scale sparse data set show the proposed four-valued logic and the relational algebra expansion of missing information have the good ability of semantic expression and computational efficiency.
更多查看译文
关键词
missing information,massive sparse data,big data,four valued logic,relational algebra expansion
AI 理解论文
溯源树
样例
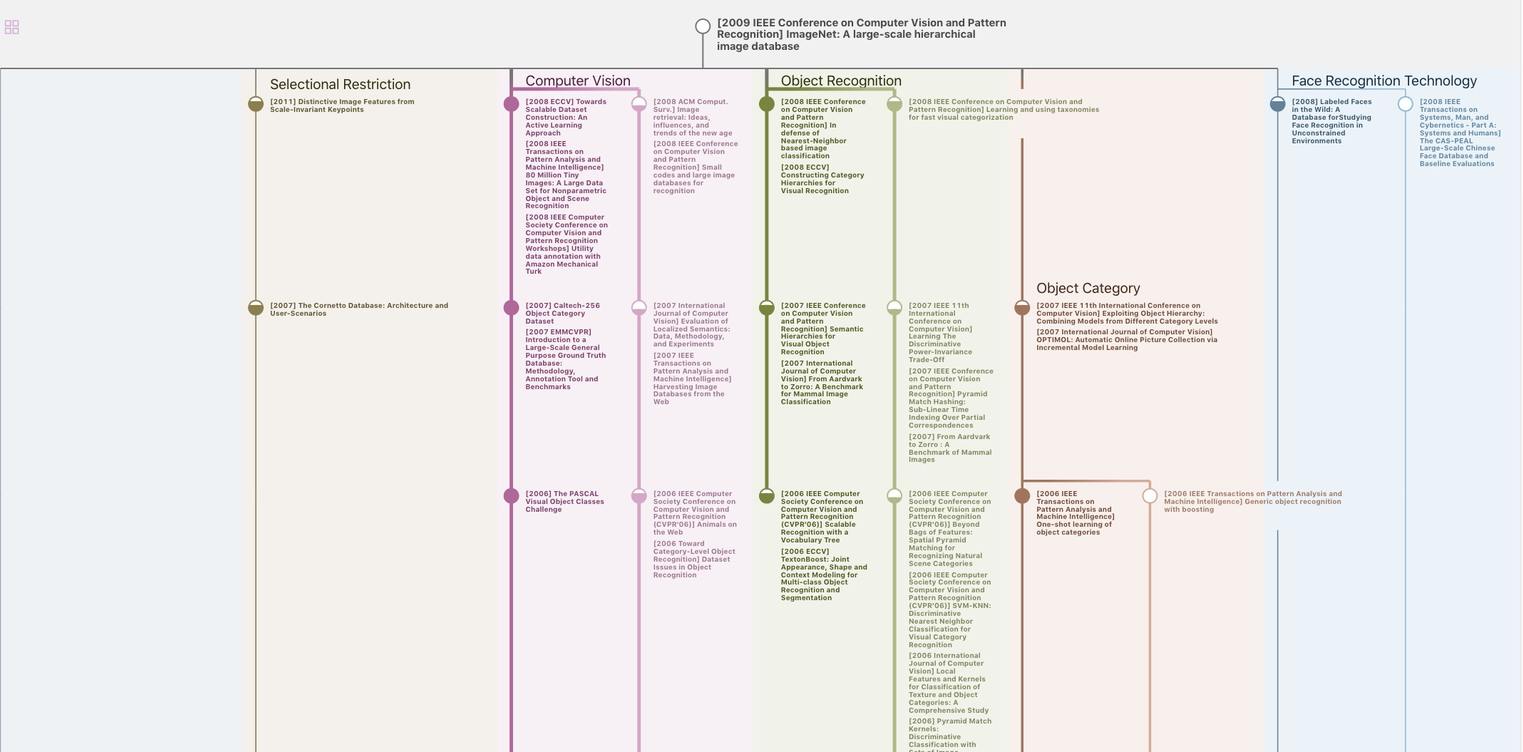
生成溯源树,研究论文发展脉络
Chat Paper
正在生成论文摘要