Using deep neural network with small dataset to predict material defects
Materials & Design(2019)
摘要
Deep neural network (DNN) exhibits state-of-the-art performance in many fields including microstructure recognition where big dataset is used in training. However, DNN trained by conventional methods with small datasets commonly shows worse performance than traditional machine learning methods, e.g. shallow neural network and support vector machine. This inherent limitation prevented the wide adoption of DNN in material study because collecting and assembling big dataset in material science is a challenge. In this study, we attempted to predict solidification defects by DNN regression with a small dataset that contains 487 data points. It is found that a pre-trained and fine-tuned DNN shows better generalization performance over shallow neural network, support vector machine, and DNN trained by conventional methods. The trained DNN transforms scattered experimental data points into a map of high accuracy in high-dimensional chemistry and processing parameters space. Though DNN with big datasets is the optimal solution, DNN with small datasets and pre-training can be a reasonable choice when big datasets are unavailable in material study.
更多查看译文
关键词
BTR,DNN,LVT,PCA,RBM,SAE,SCS,SNN,SVM,TCL
AI 理解论文
溯源树
样例
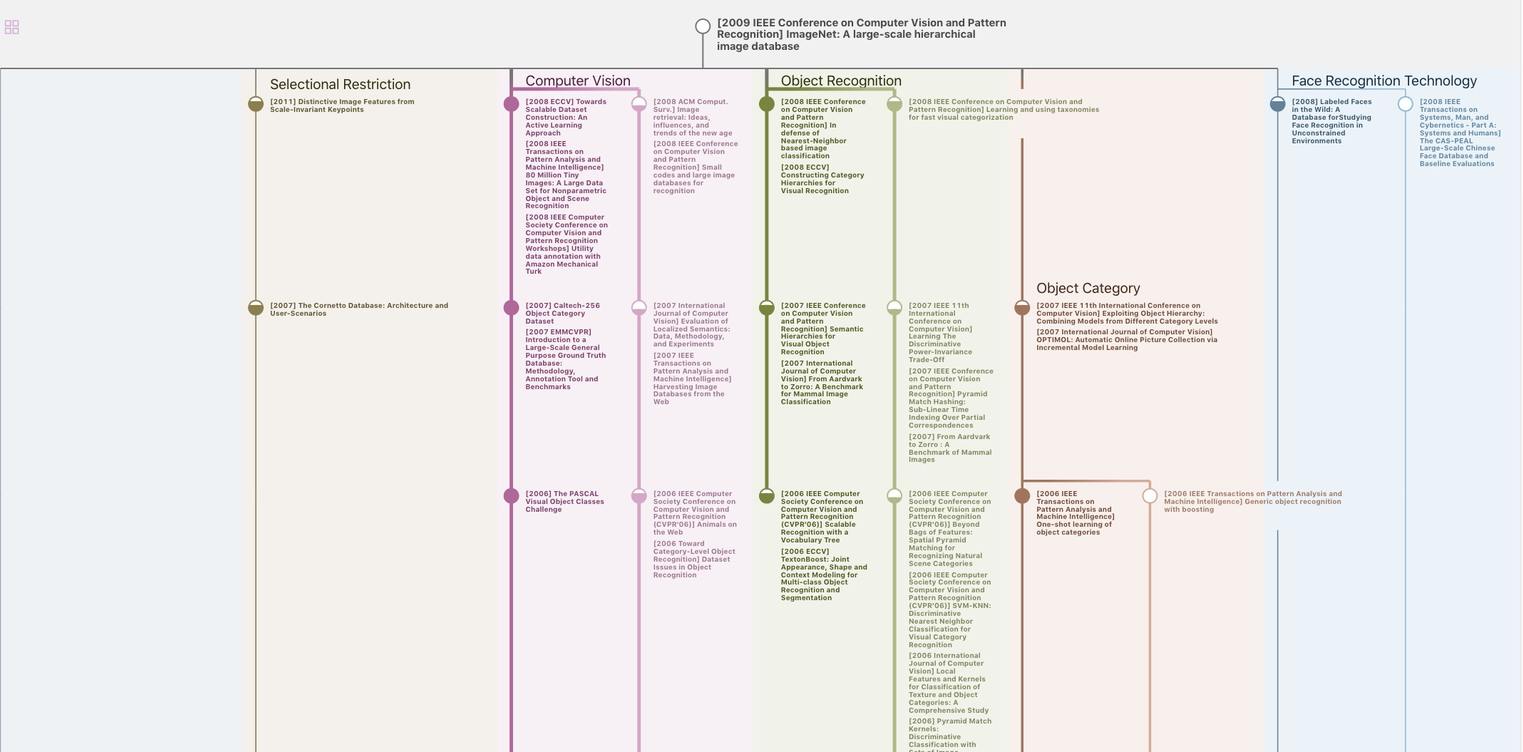
生成溯源树,研究论文发展脉络
Chat Paper
正在生成论文摘要