A Bayesian Hierarchical Modeling Framework for Correcting Reporting Bias in the U.S. Tornado Database
Weather and forecasting(2018)
摘要
The Storm Prediction Center (SPC) tornado database, generated from NCEI's Storm Data publication, is indispensable for assessing U.S. tornado risk and investigating tornado-climate connections. Maximizing the value of this database, however, requires accounting for systemically lower reported tornado counts in rural areas owing to a lack of observers. This study uses Bayesian hierarchical modeling to estimate tornado reporting rates and expected tornado counts over the central United States during 1975-2016. Our method addresses a serious solution nonuniqueness issue that may have affected previous studies. The adopted model explains 73% (>90%) of the variance in reported counts at scales of 50 km (>100 km). Population density explains more of the variance in reported tornado counts than other examined geographical covariates, including distance from nearest city, terrain ruggedness index, and road density. The model estimates that approximately 45% of tornadoes within the analysis domain were reported. The estimated tornado reporting rate decreases sharply away from population centers; for example, while >90% of tornadoes that occur within 5 km of a city with population > 100 000 are reported, this rate decreases to <70% at distances of 20-25 km. The method is directly extendable to other events subject to underreporting (e.g., severe hail and wind) and could be used to improve climate studies and tornado and other hazard models for forecasters, planners, and insurance/reinsurance companies, as well as for the development and verification of storm-scale prediction systems.
更多查看译文
关键词
Tornadoes,Climatology,Bayesian methods,Statistics
AI 理解论文
溯源树
样例
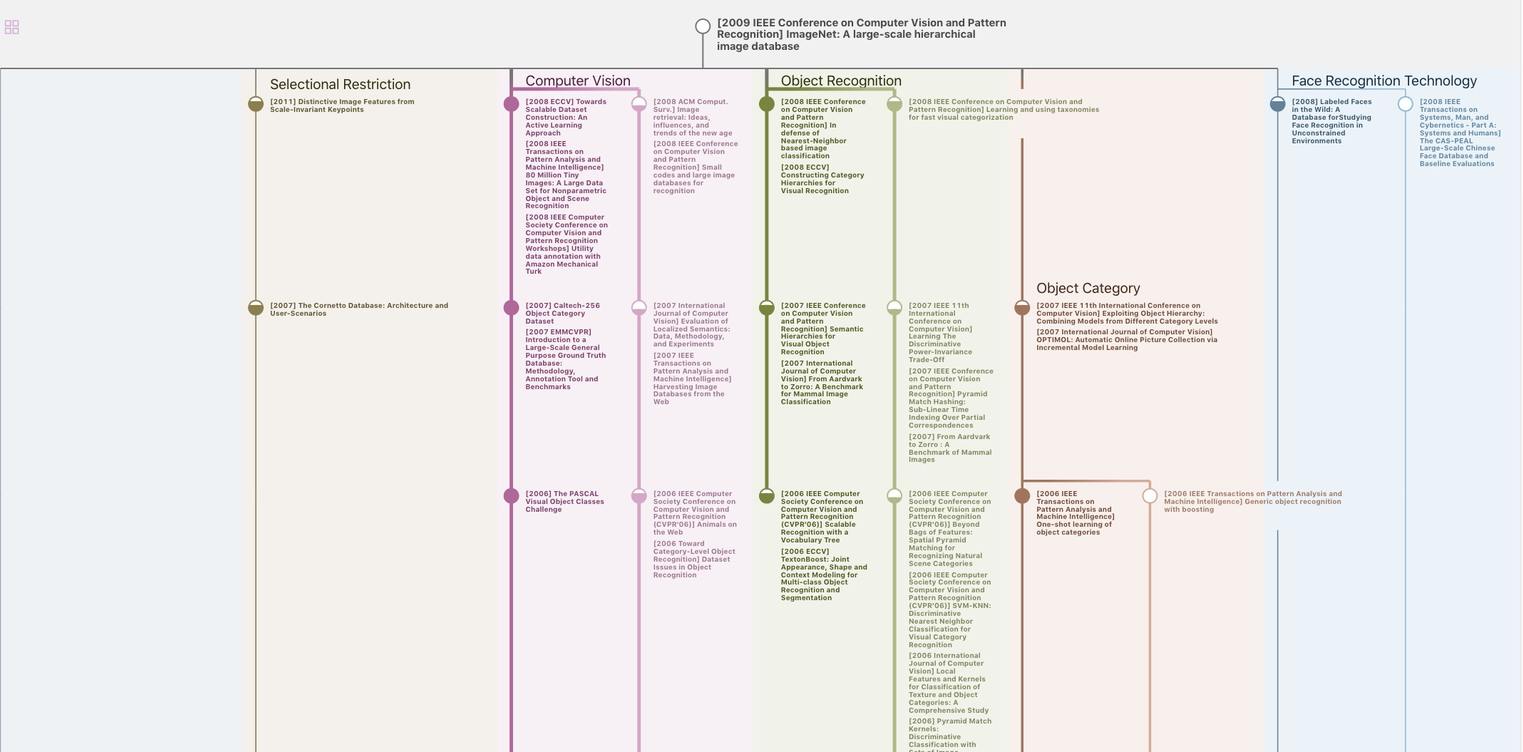
生成溯源树,研究论文发展脉络
Chat Paper
正在生成论文摘要