Low-rank Riemannian eigensolver for high-dimensional Hamiltonians
Journal of Computational Physics(2019)
摘要
Such problems as computation of spectra of spin chains and vibrational spectra of molecules can be written as high-dimensional eigenvalue problems, i.e., when the eigenvector can be naturally represented as a multidimensional tensor. Tensor methods have proven to be an efficient tool for the approximation of solutions of high-dimensional eigenvalue problems, however, their performance deteriorates quickly when the number of eigenstates to be computed increases. We address this issue by designing a new algorithm motivated by the ideas of Riemannian optimization (optimization on smooth manifolds) for the approximation of multiple eigenstates in the tensor-train format, which is also known as matrix product state representation. The proposed algorithm is implemented in TensorFlow, which allows for both CPU and GPU parallelization.
更多查看译文
关键词
Matrix product state,Tensor-Train decomposition,Riemannian optimization,Eigensolver,Vibrational spectra,Spin chains
AI 理解论文
溯源树
样例
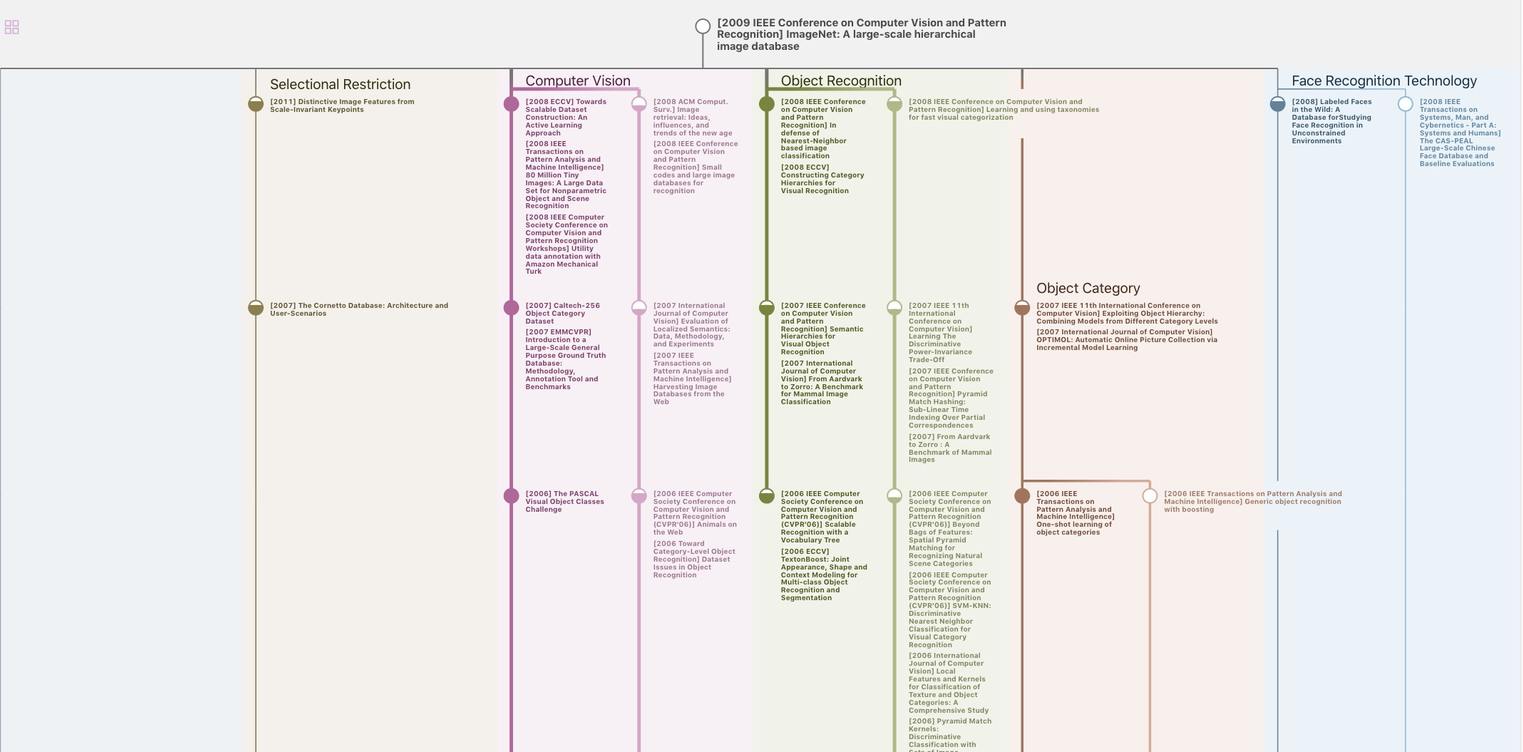
生成溯源树,研究论文发展脉络
Chat Paper
正在生成论文摘要