Machine learning-assisted discovery of GPCR bioactive ligands
arXiv: Biomolecules(2018)
摘要
While G-protein coupled receptors (GPCRs) constitute the largest class of membrane proteins, structures and endogenous ligands of a large portion of GPCRs remain unknown. Due to the involvement of GPCRs in various signaling pathways and physiological roles, the identification of endogenous ligands as well as designing novel drugs is of high interest to the research and medical communities. Along with highlighting the recent advances in structure-based ligand discovery, including docking and molecular dynamics, this article focuses on the latest advances using machine learning for discovering bioactive ligands. Machine learning, which is centered around the development and applications of algorithms that can learn from data automatically, offers immense opportunities for bioactivity prediction as well as quantitative structure-activity relationship studies. After the most recent and successful applications of machine learning for bioactive ligand discovery are reviewed, this article concludes with an outlook on deep learning methods that are capable of extracting salient information from structural data as a promising future direction for bioactive ligand discovery.
更多查看译文
AI 理解论文
溯源树
样例
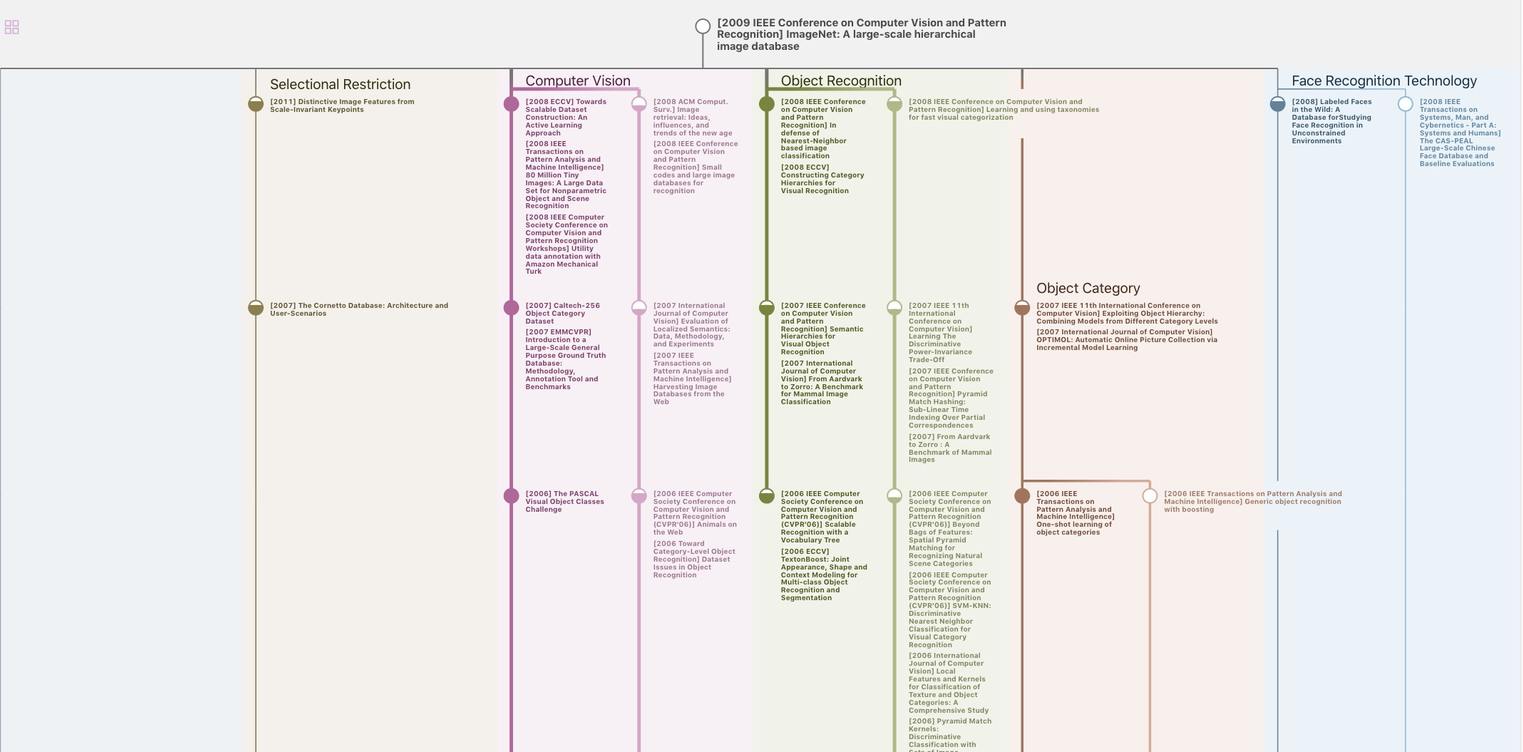
生成溯源树,研究论文发展脉络
Chat Paper
正在生成论文摘要