Using Flow Cytometry and Multistage Machine Learning to Discover Label-Free Signatures of Algal Lipid Accumulation
Physical biology(2019)
摘要
Most applications of flow cytometry or cell sorting rely on the conjugation of fluorescent dyes to specific biomarkers. However, labeled biomarkers are not always available, they can be costly, and they may disrupt natural cell behavior. Label-free quantification based upon machine learning approaches could help correct these issues, but label replacement strategies can be very difficult to discover when applied labels or other modifications in measurements inadvertently modify intrinsic cell properties. Here we demonstrate a new, but simple approach based upon feature selection and linear regression analyses to integrate statistical information collected from both labeled and unlabeled cell populations and to identify models for accurate label-free single-cell quantification. We verify the method's accuracy to predict lipid content in algal cells (Picochlorum soloecismus) during a nitrogen starvation and lipid accumulation time course. Our general approach is expected to improve label-free single-cell analysis for other organisms or pathways, where biomarkers are inconvenient, expensive, or disruptive to downstream cellular processes.
更多查看译文
关键词
machine learning,flow cytometry,single cell,label-free quantification,algal biofuels
AI 理解论文
溯源树
样例
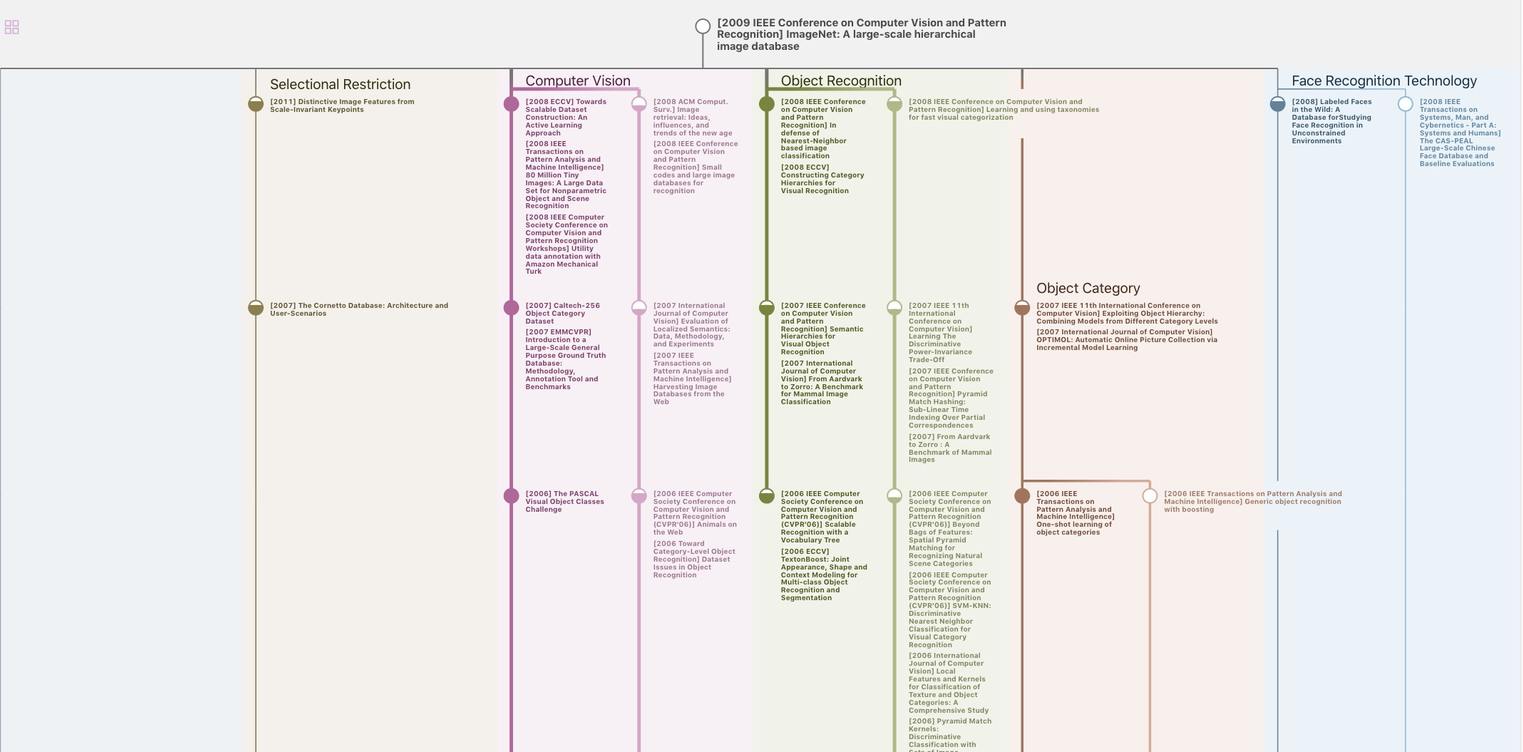
生成溯源树,研究论文发展脉络
Chat Paper
正在生成论文摘要