A Reinforcement Learning Approach to Autonomous Decision Making of Intelligent Vehicles on Highways
IEEE Transactions on Systems, Man, and Cybernetics(2020)
摘要
Autonomous decision making is a critical and difficult task for intelligent vehicles in dynamic transportation environments. In this paper, a reinforcement learning approach with value function approximation and feature learning is proposed for autonomous decision making of intelligent vehicles on highways. In the proposed approach, the sequential decision making problem for lane changing and overtaking is modeled as a Markov decision process with multiple goals, including safety, speediness, smoothness, etc. In order to learn optimized policies for autonomous decision-making, a multiobjective approximate policy iteration (MO-API) algorithm is presented. The features for value function approximation are learned in a data-driven way, where sparse kernel-based features or manifold-based features can be constructed based on data samples. Compared with previous RL algorithms such as multiobjective Q-learning, the MO-API approach uses data-driven feature representation for value and policy approximation so that better learning efficiency can be achieved. A highway simulation environment using a 14 degree-of-freedom vehicle dynamics model was established to generate training data and test the performance of different decision-making methods for intelligent vehicles on highways. The results illustrate the advantages of the proposed MO-API method under different traffic conditions. Furthermore, we also tested the learned decision policy on a real autonomous vehicle to implement overtaking decision and control under normal traffic on highways. The experimental results also demonstrate the effectiveness of the proposed method.
更多查看译文
关键词
Autonomous decision-making,intelligent driving vehicles,Markov decision processes (MDPs),multiobjective,reinforcement learning (RL),value function approximation
AI 理解论文
溯源树
样例
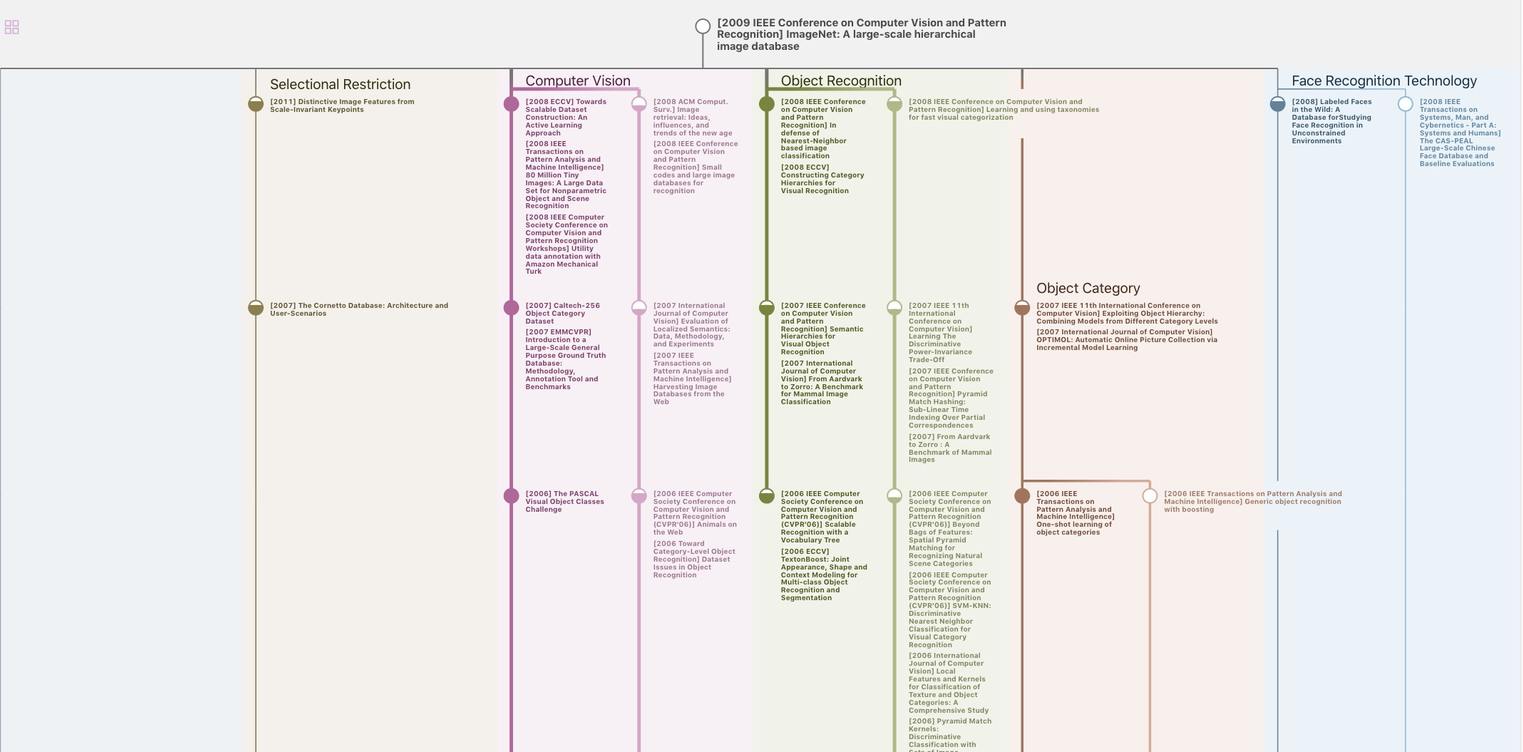
生成溯源树,研究论文发展脉络
Chat Paper
正在生成论文摘要