Scalable probabilistic matrix factorization for single-cell RNA-seq analysis
bioRxiv(2018)
摘要
Motivation: The gene expression profile of a cell dictates its function in molecular processes, and can be used to probe its health status. This represents a step forward in the deep characterization of diseases such as cancer and may lead to breakthroughs in their treatment. The technology used to measure the gene expression of isolated cells, single-cell RNA-seq (scRNA-seq), has emerged in the last decade as a key enabler of this progress. However, the use of existing methods for dimensionality reduction, clustering and differential expression is limited by the specificities of the data obtained from scRNA-seq experiments, where technical factors may confound analyses of the true biological signal and contribute to spurious results. To overcome this issue, a possible approach is designing probabilistic generative models of the data with hidden variables encoding different underlying processes. Results: We propose two novel probabilistic models for scRNA-seq data: modified probabilistic count matrix factorization (m-pCMF) and Bayesian zero-inflated negative binomial factorization (ZINBayes). These build upon previous models in the literature while leveraging scalable Bayesian inference via variational methods. We show that the proposed methods are competitive with the state-of-the-art models for robust dimensionality reduction in modern data sets, and improve upon the current best Bayesian model for small numbers of cells. The results show that building probabilistic models of latent variables which encode domain knowledge and using variational inference constitute a promising approach to analyse scRNA-seq data in a scalable way. Availability: m-pCMF and ZINBayes are publicly available as Python packages at https://github.com/pedrofale/, along with the code to reproduce all the results. Contact: susanavinga@tecnico.ulisboa.pt
更多查看译文
AI 理解论文
溯源树
样例
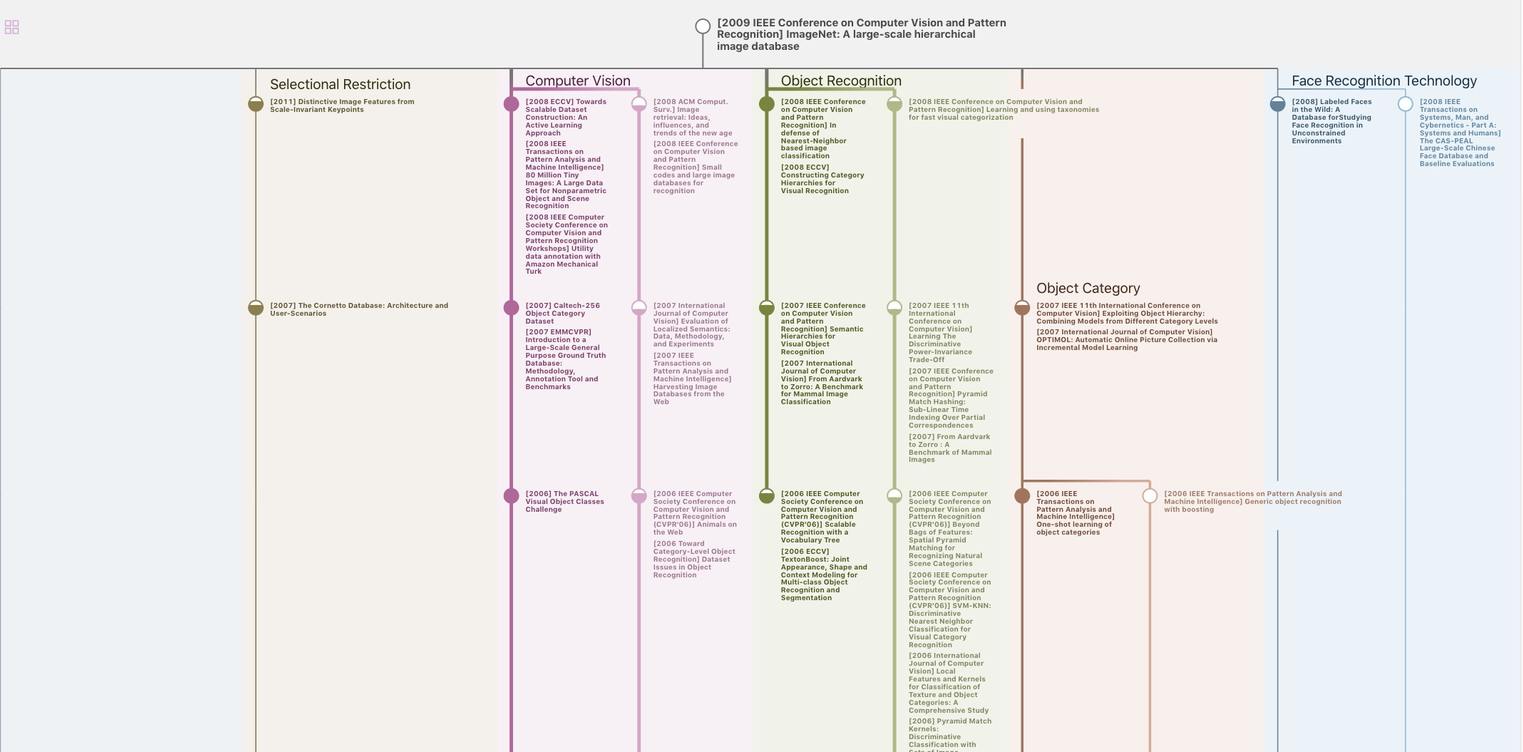
生成溯源树,研究论文发展脉络
Chat Paper
正在生成论文摘要