Data-Driven Reconstruction of Gravitationally Lensed Galaxies using Recurrent Inference Machines
ASTROPHYSICAL JOURNAL(2019)
摘要
We present a machine-learning method for the reconstruction of the undistorted images of background sources in strongly lensed systems. This method treats the source as a pixelated image and utilizes the recurrent inference machine to iteratively reconstruct the background source given a lens model. Our architecture learns to maximize the likelihood of the model parameters (source pixels) given the data using the physical forward model (ray-tracing simulations) while implicitly learning the prior of the source structure from the training data. This results in better performance compared to linear inversion methods, where the prior information is limited to the two-point covariance of the source pixels approximated with a Gaussian form, and often specified in a relatively arbitrary manner. We combine our source reconstruction network with a convolutional neural network that predicts the parameters of the mass distribution in the lensing galaxies directly from telescope images, allowing a fully automated reconstruction of the background source images and the foreground mass distribution.
更多查看译文
关键词
Convolutional neural networks,Strong gravitational lensing,Neural networks
AI 理解论文
溯源树
样例
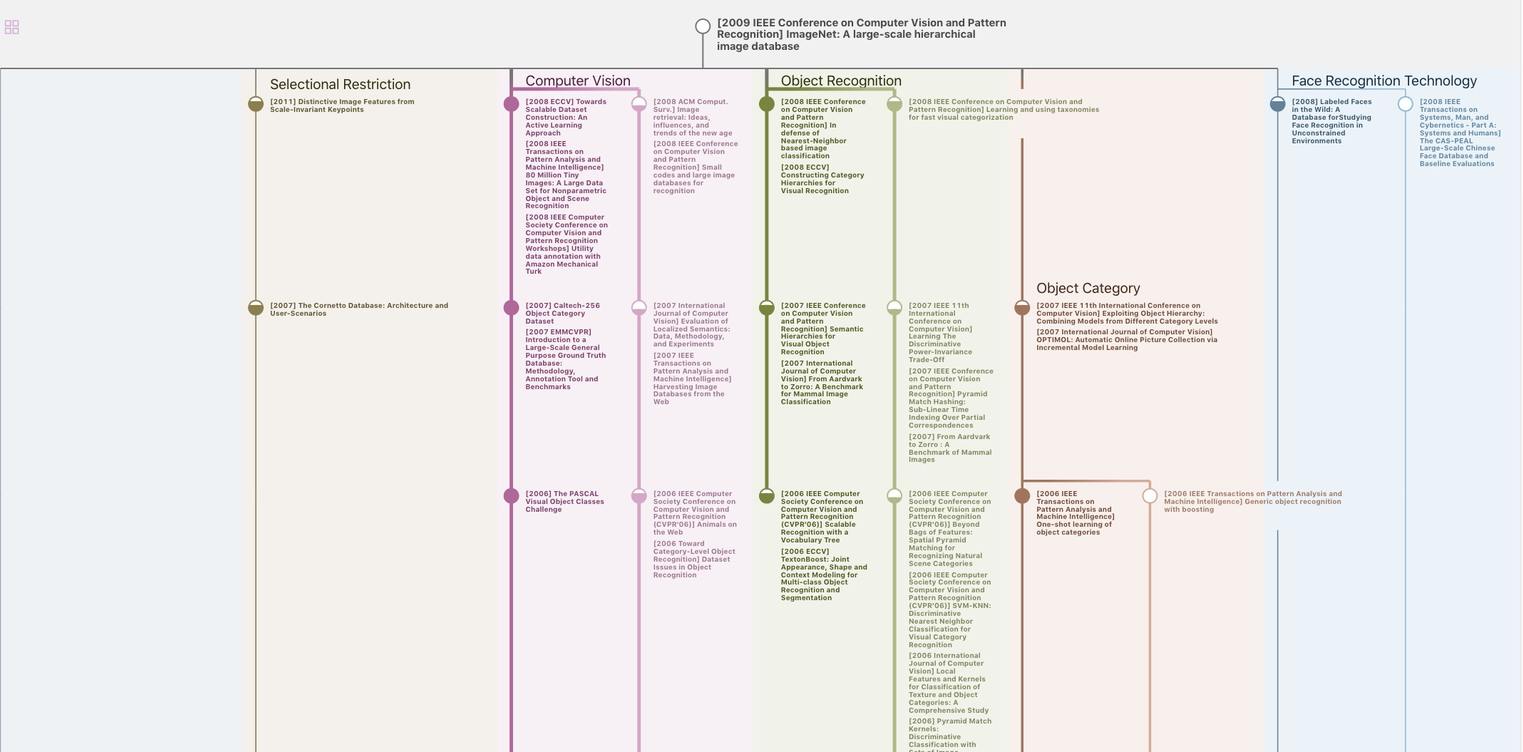
生成溯源树,研究论文发展脉络
Chat Paper
正在生成论文摘要