On the Efficiency of Random Permutation for ADMM and Coordinate Descent.
MATHEMATICS OF OPERATIONS RESEARCH(2020)
摘要
Random permutation is observed to be powerful for optimization algorithms: for multiblock ADMM (alternating direction method of multipliers), whereas the classical cyclic version diverges, the randomly permuted version converges in practice; for BCD (block coordinate descent), the randomly permuted version is typically faster than other versions. In this paper we provide strong theoretical evidence that random permutation has positive effects on ADMM and BCD, by analyzing randomly permuted ADMM (RP-ADMM) for solving linear systems of equations, and randomly permuted BCD (RP-BCD) for solving unconstrained quadratic problems. First, we prove that RP-ADMM converges in expectation for solving systems of linear equations. The key technical result is that the spectrum of the expected update matrix of RP-BCD lies in (-1/3, 1), instead of the typical range (-1, 1). Second, we establish expected convergence rates of RP-ADMM for solving linear systems and RP-BCD for solving unconstrained quadratic problems. This expected rate of RP-BCD is O(n) times better than the worst-case rate of cyclic BCD, thus establishing a gap of at least O(n) between RP-BCD and cyclic BCD. To analyze RP-BCD, we propose a conjecture of a new matrix algebraic mean-geometric mean inequality and prove a weaker version of it.
更多查看译文
关键词
random permutation,ADMM,coordinate descent,convergence,matrix AM-GM inequality,spectral radius
AI 理解论文
溯源树
样例
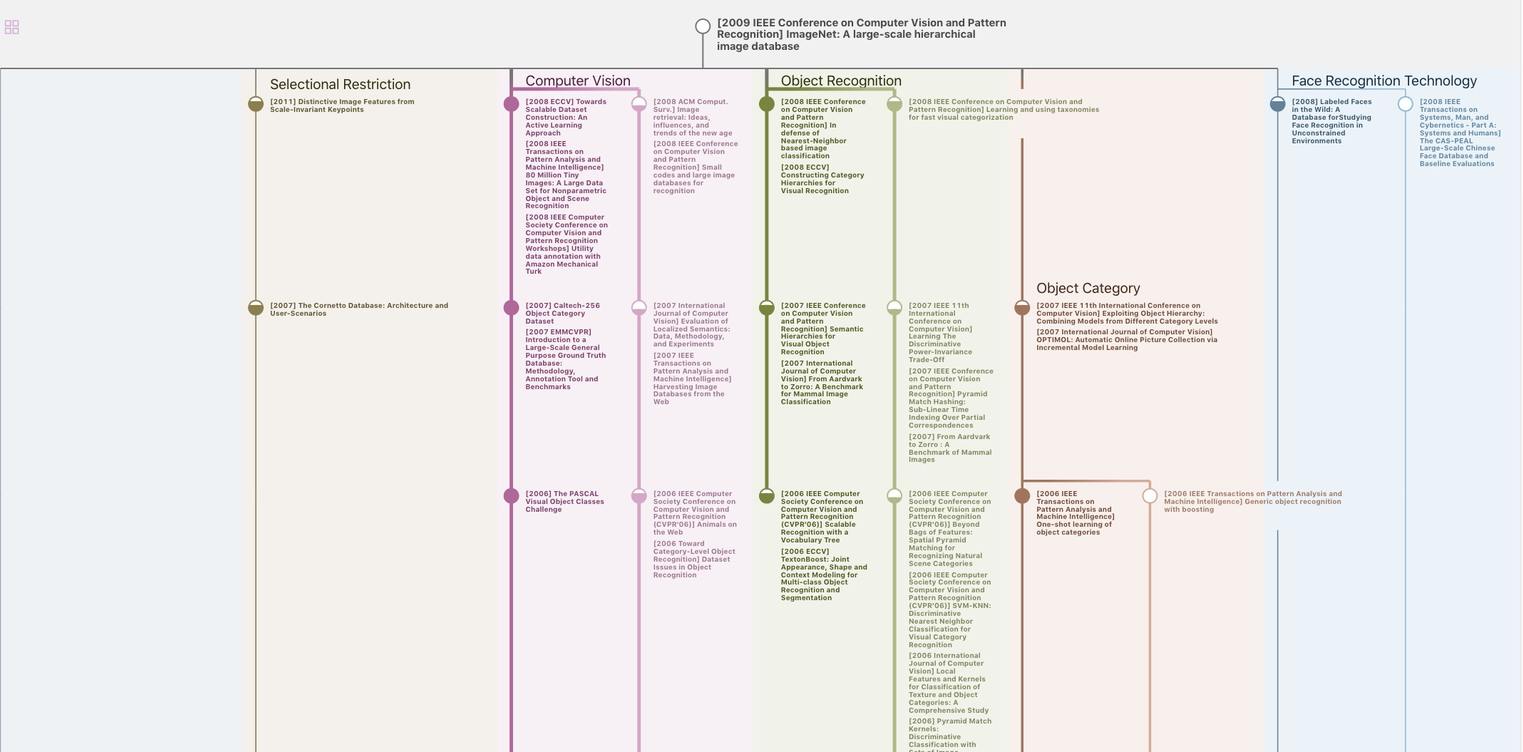
生成溯源树,研究论文发展脉络
Chat Paper
正在生成论文摘要