Investigating the Efficiency of WordNet as Background Knowledge for Document Clustering
Journal of Engineering Research and Technology(2016)
摘要
Traditional techniques of document clustering do not consider the semantic relationships between words when assigning documents to clusters. For instance, if two documents talk about the same topic but by using different words, these techniques may assign documents to different clusters. Many efforts have approached this problem by enriching the document’s representation with background knowledge from WordNet. These efforts, however, often showed conflicting results: While some researches claimed that WordNet had the potential to improve the clustering performance by its capability to capture and estimate similarities between words, other researches claimed that WordNet provided little or no enhancement to the obtained clusters. This work aims to experimentally resolve this contradiction between the two teams, and explain why WordNet could be useful in some cases while not in others, and what factors can influence the use of WordNet for document clustering. We conducted a set of experiments in which WordNet was used for document clustering with various settings including different datasets, different ways of incorporating semantics into the document’s representation and different similarity measures. Results showed that different experimental settings may yield different clusters: For example, the influence of WordNet’s semantic features varies according to the dataset being used. Results also revealed that WordNet-based similarity measures do not seem to improve clustering, and that there was no certain measure to ensure the best clustering results.
更多查看译文
关键词
wordnet,background knowledge,clustering
AI 理解论文
溯源树
样例
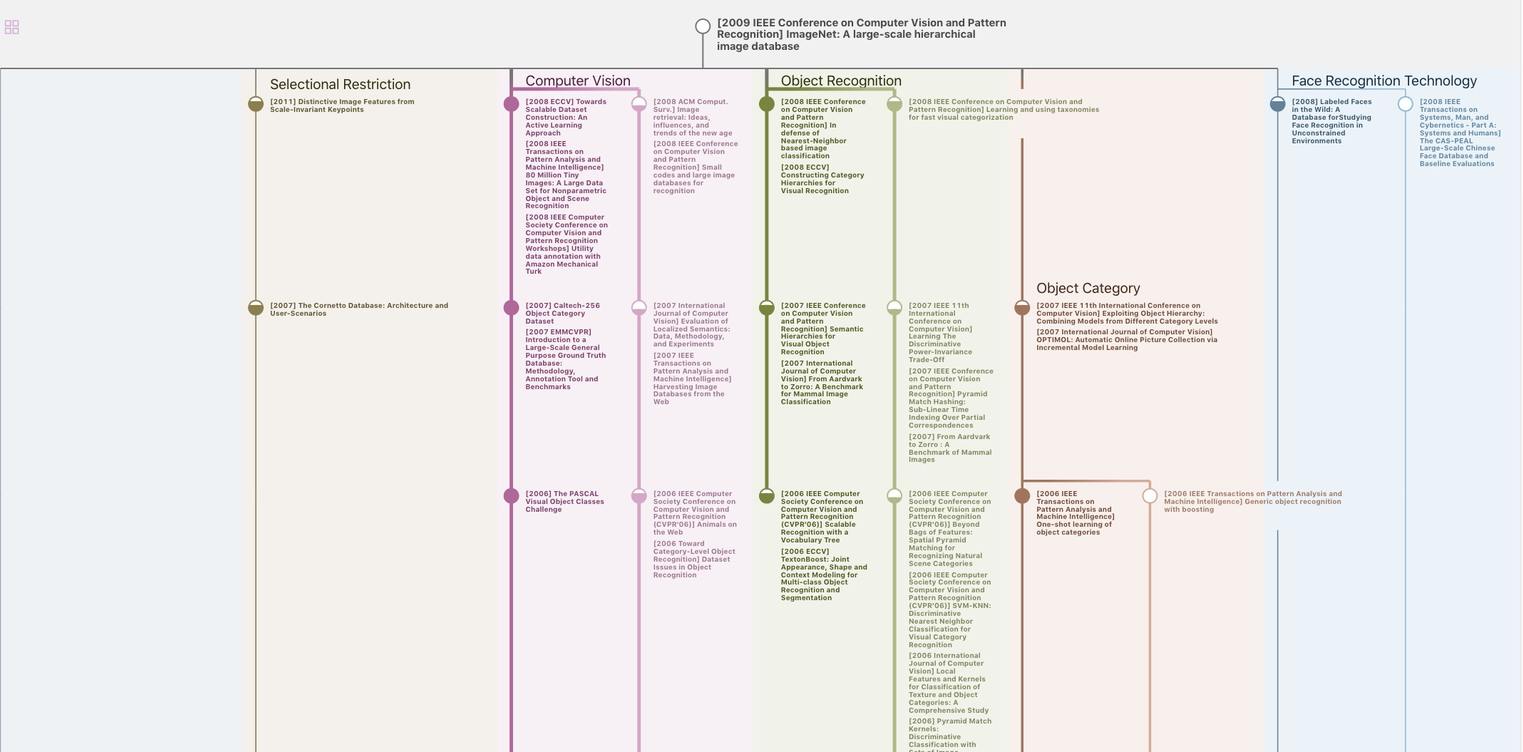
生成溯源树,研究论文发展脉络
Chat Paper
正在生成论文摘要