An Asynchronous Advantage Actor-Critic Reinforcement Learning Method For Stock Selection And Portfolio Management
PROCEEDINGS OF THE 2018 2ND INTERNATIONAL CONFERENCE ON BIG DATA RESEARCH (ICBDR 2018)(2018)
摘要
Computation finance has been a classical field that uses computer techniques to handle financial challenges. The most popular domains include financial forecast and portfolio management. They often involve large datasets with complex relations. Due to the special properties of computation finance problems, machine learning techniques, especially deep learning techniques, are widely used as the quantitative analysis tool. In this paper, we try to apply the state-of-art Asynchronous Advantage Actor-Critic algorithm to solve the portfolio management problem and design a standalone deep reinforcement learning model. In the simulated market environment with practical portfolio constrain settings, asset value managed by the proposed machine learning model largely outperforms S&P500 stock index in the test period.
更多查看译文
关键词
Computational finance, portfolio management, machine learning, deep reinforcement learning, asynchronous advantage actor-critic
AI 理解论文
溯源树
样例
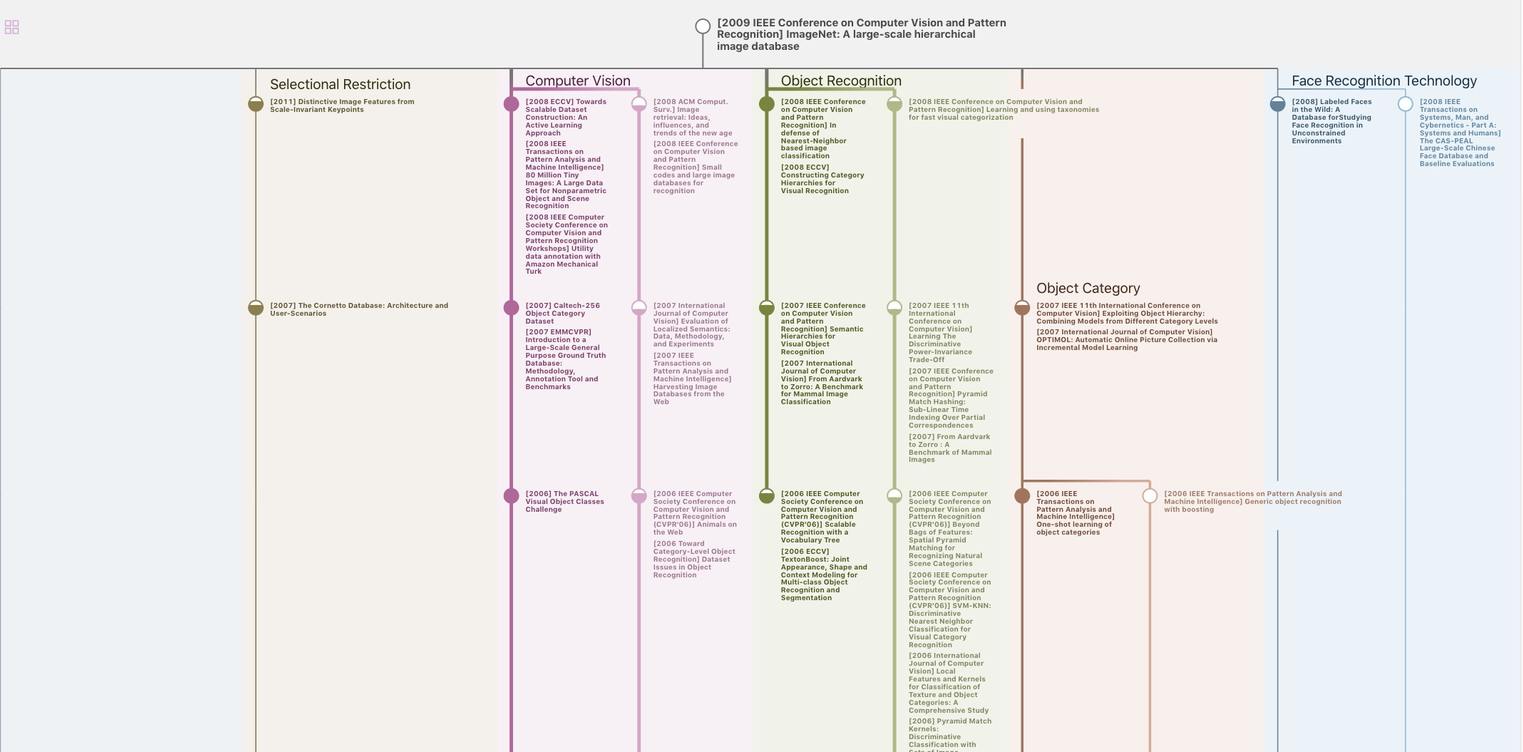
生成溯源树,研究论文发展脉络
Chat Paper
正在生成论文摘要