On Computation of Koopman Operator from Sparse Data
arXiv: Dynamical Systems(2019)
摘要
In this paper we propose a novel approach to compute the Koopman operator from sparse time series data. In recent years there has been considerable interests in operator theoretic methods for data-driven analysis of dynamical systems. Existing techniques for the approximation of the Koopman operator require sufficiently large data sets, but in many applications, the data set may not be large enough to approximate the operators to acceptable limits. In this paper, using ideas from robust optimization, we propose an algorithm to compute the Koopman operator from sparse data. We enrich the sparse data set with artificial data points, generated by adding bounded artificial noise and and formulate the noisy robust learning problem as a robust optimization problem and show that the optimal solution is the Koopman operator with smallest error. We illustrate the efficiency of our proposed approach in three different dynamical systems, namely, a linear system, a nonlinear system and a dynamical system governed by a partial differential equation.
更多查看译文
关键词
sparse time series data,operator theoretic methods,data-driven analysis,dynamical system,artificial data points,Koopman operator computation,bounded artificial noise,robust optimization problem,optimal solution,robust learning problem,linear system,nonlinear system,partial differential equation
AI 理解论文
溯源树
样例
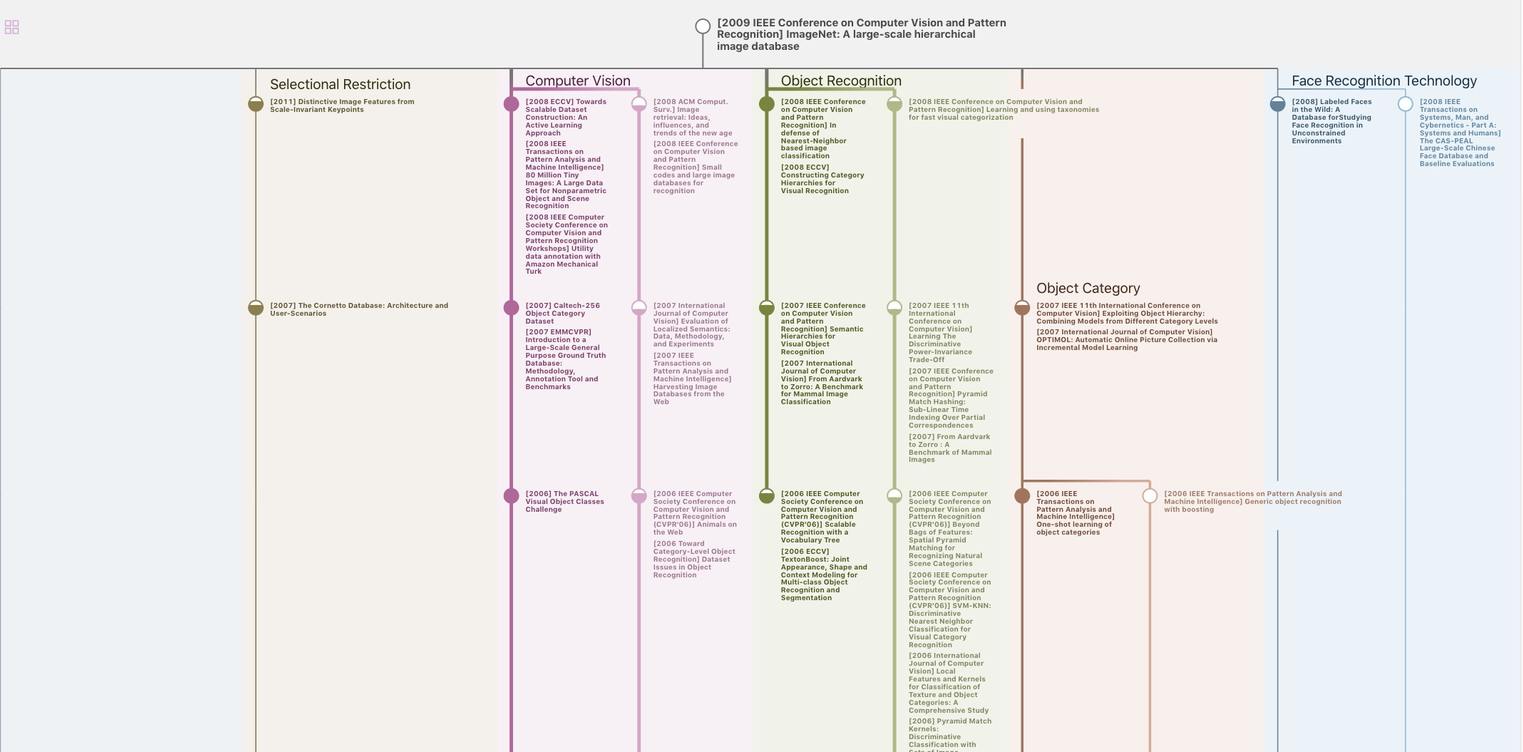
生成溯源树,研究论文发展脉络
Chat Paper
正在生成论文摘要