A Line of MEA Signal Analysis Methods for Human Stem Cell-Derived and Other Dynamic Neuronal Cultures
Frontiers in Cellular Neuroscience(2018)
摘要
Event Abstract Back to Event A Line of MEA Signal Analysis Methods for Human Stem Cell-Derived and Other Dynamic Neuronal Cultures Jarno M. A. Tanskanen1*, Fikret Emre Kapucu2, 3 and Jari A. K. Hyttinen1 1 Tampere University of Technology, BioMediTech Institute and Faculty of Biomedical Sciences and Engineering, Finland 2 Aarhus University, Danish Research Institute of Translational Neuroscience - DANDRITE, Denmark 3 Aarhus University, Department of Biomedicine, Denmark Abstract Here, we review the line of MEA signal analysis methods we have developed. Our target has been the analysis needs of MEA signals measured from developing human pluripotent stem cell (hPSC)–derived neuronal cells and networks, which exhibit highly varying spiking statistics. Such MEA signals do not lend themselves well to analysis by traditional means. A hypothesis in our work has also been that MEA measurements carry more information than assessable by the commonly used spike and burst analysis methods. The MEA signal analysis areas advanced are: 1) Neuronal action potential spike detection [1,2]; 2) Adaptive burst detection [3]; 3) Burst quantification [4]; 4) Joint analysis of bursts and sorted spikes [5,6]; and 5) Network connectivity/synchronization analysis [7-11], including the developed correlated spectral entropy method (CorSE) [8,11]. A more comprehensive introduction and discussion of these methods has been presented by Kapucu [12]. Spike Detection Spike detection is the basis of all analysis based on spikes. Spike detection [13,14] is often performed by thresholding at a usually fixed or adaptive threshold (TH) based on a standard deviation of the signal. Here, an objective MEA signal analysis-based thresholding method was developed. In the smoothed derivative of a spike count histogram, shoulder-like features may be observable approximately above and below background noise amplitudes, and our method [1] sets the THs accordingly automatically. Matlab code for the methods is freely available [2]. Adaptive Burst Detection Bursts are generally considered to indicate network activity. Bursts are detected [15-17] typically by the fulfillment of at least two conditions: a fixed minimum number of spikes and a fixed maximum interspike interval (ISI) in a burst. ISI histograms have been employed to assess firing characteristics [18]. In some MEA signals, intra- and interburst ISIs are well separated (Fig. 1A). However, for hESC-derived neuronal cultures, many different cases have been observed (Fig. 1C). The spiking statistics of developing hPSC-derived neurons and networks vary greatly, and burst detection needs to be based on signal dynamics. Here, such a method was developed [3]. Our burst detection method [3] is based on the cumulative moving average (CMA) of ISI histogram (Fig. 1D), and does not depend on intraburst and other ISIs begin separable in the histogram. In our method, the maximum ISI allowed in a burst in a particular MEA signal, i.e., the ISI TH defining a burst, is found at the maximum of the CMA curve multiplied by alpha, whose value depends on the kurtosis of the ISI histogram (see [3] for a possible mapping between alpha and kurtosis) (Fig. 1D). A recommendable review of eight adaptive methods has been presented by Cotterill et al. [19]. Adaptive methods are crucial for detecting bursts recorded from dynamic cultures. Our CMA method has been successfully used in burst detection from MEA signals measured from hPSC-derived neuronal networks [20-23]. Burst Quantification Burst detection and quantification is possible also using e.g., entropy measures. In [4], we calculated sample entropy (SmE) and spectral entropy (SE) in running windows forming SmE and SE signals for MEA signals from rat cortical cell cultures. Comparing the entropy signals with raw signals and detected bursts indicated that these entropy measures have potential for burst detection and quantification. SmE appeared to be more sensitive to burst duration and number of spikes in bursts, whereas SmE was found potential also for quantifying/classifying presumably different kinds of bursts. In later unpublished experiments with hPSC-derived neurons, SE was able to indicate also possible bursts with spikes buried in background noise, i.e., undetectable by visual assessment. Joint Analysis of Bursts and Sorted Spikes To extract more information from the internal dynamics of network bursts, a method for the joint analysis of bursts and spike waveforms was developed [5]. In the joint analysis, spike sorting is used to generate spike types, and bursts are detected, using any methods suited for the signals at hand. Thereafter, each burst spike is associated with a spike type. Thus, spike type compositions of bursts are obtained. The method can be applied in the analysis of MEA recordings from any experimental paradigms in which changes in burst generating cells and/or networks can be expected. Changes may be observable comparing the spike type compositions of bursts before and after alterations, possibly even when no changes in burst durations, numbers of spikes in bursts, or in other traditional burst metrics could be observed. Matlab code for the methods is freely available [6]. CorSE for Functional Network Connectivity Assessment Entropy in its different forms can be used to assess neuronal networks based on MEA signals [7-11]. Particularly, we have demonstrated that spatial network relations can be revealed by time-variant SE signals, and devised CorSE [8]. In CorSE, an SE signal is formed for each MEA signal, and all pair-wise SE signal correlations (CorSE values) are calculated. A CorSE value quantifies how similarly the uniformities of the frequency spectra of the two signals change in time. Functional connectivity of the local networks in the vicinities of two MEA electrodes is deemed if the CorSE value is sufficiently high. A comparison of network connectivity detected by CorSE and a few other methods is shown in Fig. 2B-E, and an example of tracking network formation in Fig. 2F. CorSE could be particularly beneficial in tracking the formation of maturing neuronal networks since it does not depend on any predetermined spike/burst detection parameters or alike, or even of the spike timings: To observe functional connectivity, it is sufficient that the complexities of the frequency characteristics of the two MEA signals change similarly. Work using CorSE in the analysis of maturing hPSC-derived neuronal networks is ongoing. Matlab code for CorSE is freely available [11]. Conclusions and Discussion We have developed a line of MEA signal analysis methods motivated by the analysis needs of dynamic hPSC-derived neuronal networks. However, they can be applied in many other areas as well, from basic neuroscience to toxicology and drug studies. We hope that the methods will be found useful in gaining more information on the development and functioning of living neuronal networks. Figure 1 Figure 2 Acknowledgements This work has been funded by the Human Spare Parts Project funded by Business Finland (formerly the Finnish Funding Agency for Technology and Innovation (TEKES)), 3DNeuroN project in the European Union’s Seventh Framework Programme, Future and Emerging Technologies (grant agreement number 296590). The work of JMAT has been funded by Jane and Aatos Erkko Foundation (grant ‘Biological Neuronal Communications and Computing with ICT’). References [1] Tanskanen JMA, Kapucu FE, Vornanen I et al (2016) Automatic objective thresholding to detect neuronal action potentials. In: 2016 24th European Signal Processing Conference (EUSIPCO), Budapest, Hungary, August-September 2016, pp. 662-666. https://doi.org/10.1109/eusipco.2016.7760331 [2] Tanskanen J (2017) Automatic objective neuronal spike detection. Available via MATLAB Central File Exchange, The MathWorks, Inc. https://se.mathworks.com/matlabcentral/fileexchange/55227. Accessed 18 March 2018. [3] Kapucu FE, Tanskanen JMA, Mikkonen JE et al (2012) Burst analysis tool for developing neuronal networks exhibiting highly varying action potential dynamics. Front Comput Neurosci 6:38. https://doi.org/10.3389/fncom.2012.00038 [4] Kapucu FE, Mikkonen JE, Tanskanen JMA et al (2015) Quantification and automatized adaptive detection of in vivo and in vitro neuronal bursts based on signal complexity. In: Proc. 2015 37th Annual International Conference of the Engineering in Medicine and Biology Society (EMBC), Milan, Italy, pp. 4729-4732. https://doi.org/10.1109/EMBC.2015.7319450 [5] Kapucu FE, Mäkinen ME-L, Tanskanen JMA et al (2016) Joint analysis of extracellular spike waveforms and neuronal network bursts. J Neurosci Methods 259:143-155. https://doi.org/10.1016/j.jneumeth.2015.11.022 [6] Kapucu (2015) Joint analysis of extracellular spike waveforms and neuronal network bursts. [Software] Available via MATLAB Central File Exchange, The MathWorks, Inc. https://se.mathworks.com/matlabcentral/fileexchange/54277. Accessed 18 March 2018. [7] Kapucu FE, Mikkonen JE, Tanskanen JMA et al (2016) Analyzing the feasibility of time correlated spectral entropy for the assessment of neuronal synchrony. In: 2016 IEEE 38th Annual International Conference of the Engineering in Medicine and Biology Society (EMBC), Orlando, FL, pp. 1595-1598. https://doi.org/10.1109/EMBC.2016.7591017 [8] Kapucu FE, Välkki I, Mikkonen JE et al (2016) Spectral entropy based neuronal network synchronization analysis based on microelectrode array measurements. Front Comput Neurosci 10:112. https://doi.org/10.3389/fncom.2016.00112 [9] Kapucu FE, Tanskanen JMA, Christophe F et al (2017) Evaluation of the effective and functional connectivity estimators for microelectrode array recordings during in vitro neuronal network maturation. In: Eskola H, Väisänen O, Viik J et al (eds) European Medical and Biological Engineering Conference, Nordic-Baltic Conference on Biomedical Engineering and Medical Physics (EMBEC & NBC 2017), Tampere, Finland, June 2017. IFMBE Proceedings, vol. 65, Springer, Singapore, pp. 1105-1108. https://doi.org/10.1007/978-981-10-5122-7_276 [10] Kapucu FE, Välkki I, Christophe F et al (2017) On electrophysiological signal complexity during biological neuronal network development and maturation. In: 2017 39th Annual International Conference of the IEEE Engineering in Medicine and Biology Society (EMBC), Seogwipo, South Korea, pp. 3333-3338. https://doi.org/10.1109/EMBC.2017.8037570 [11] Kapucu (2016) Spectral entropy based neuronal network synchronization analysis : CorSE. [Software] Available via MATLAB Central File Exchange, The MathWorks, Inc. https://se.mathworks.com/matlabcentral/fileexchange/59626. Accessed 18 March 2018. [12] Kapucu FE (2016) Methods to enhance information extraction from microelectrode array measurements of neuronal networks. Dissertation, Tampere University of Technology, vol. 1438. http://urn.fi/URN:ISBN:978-952-15-3862-9 [13] Lewicki MS (1998) A review of methods for spike sorting: The detection and classification of neural action potentials. Network: Comput Neural Syst 9(4): R53-R78. https://doi.org/10.1088/0954-898x/9/4/001 [14] Wilson SB, Emerson R (2002) Spike detection: A review and comparison of algorithms. Clin Neurophysiol 113(12):1873-1881. https://doi.org/10.1016/S1388-2457(02)00297-3 [15] Chiappalone M, Novellino A, Vajda I et al (2005) Burst detection algorithms for the analysis of spatio-temporal patterns in cortical networks of neurons. Neurocomputing 65-66:653-662. https://doi.org/10.1016/j.neucom.2004.10.094 [16] Wagenaar DA, Pine J, Potter SM (2006) An extremely rich repertoire of bursting patterns during the development of cortical cultures. BMC Neurosci 7:11. https://doi.org/10.1186/1471-2202-7-11 [17] Mazzoni A, Broccard FD, Garcia-Perez E et al (2007) On the dynamics of the spontaneous activity in neuronal networks. PLoS One 2(5):e439. https://doi.org/10.1371/journal.pone.0000439 [18] Christodoulou C, Bugmann G (2001) Coefficient of variation vs. mean interspike interval curves: What do they tell us about the brain? Neurocomputing 38-40:1141-1149. https://doi.org/10.1016/S0925-2312(01)00480-5 [19] Cotterill E, Charlesworth P, Thomas CW et al (2016) A comparison of computational methods for detecting bursts in neuronal spike trains and their application to human stem cell-derived neuronal networks. J Neurophysiol 116(2):306-321. https://doi.org/10.1152/jn.00093.2016 [20] Hyysalo A, Ristola M, Mäkinen ME-L et al (2017) Laminin alpha5 substrates promote survival, network formation and functional development of human pluripotent stem cell-derived neurons in vitro. Stem Cell Res 24:118-127. https://doi.org/10.1016/j.scr.2017.09.002 [21] Toivanen M, Pelkonen A, Mäkinen M et al (2017) Optimised PDMS tunnel devices on MEAs increase the probability of detecting electrical activity from human stem cell-derived neuronal networks. Front Neurosci 11:606. https://doi.org/10.3389/fnins.2017.00606 [22] Paavilainen T, Pelkonen A, Mäkinen ME-L et al (2018) Effect of prolonged differentiation on functional maturation of human pluripotent stem cell-derived neuronal cultures. Stem Cell Res 27:151-161. https://doi.org/10.1016/j.scr.2018.01.018 [23] Mäkinen ME-L, Ylä-Outinen L, Narkilahti S (2018) GABA and gap junctions in the development of synchronized activity in human pluripotent stem cell-derived neural networks. Front Cell Neurosci 12:56. https://doi.org/10.3389/fncel.2018.00056 Keywords: microelectrode array, MEA, Human pluripotent stem cell, HPSC, Electrophysiology, Spike Detection, thresholding, Burst detection, interspike interval, cumulative moving average, neuronal network, synchronization, functional connectivity, Spectral entropy, Corse Conference: MEA Meeting 2018 | 11th International Meeting on Substrate Integrated Microelectrode Arrays, Reutlingen, Germany, 4 Jul - 6 Jul, 2018. Presentation Type: Poster Presentation Topic: Microelectrode Array Technology Citation: Tanskanen J, Kapucu F and Hyttinen J (2019). A Line of MEA Signal Analysis Methods for Human Stem Cell-Derived and Other Dynamic Neuronal Cultures. Conference Abstract: MEA Meeting 2018 | 11th International Meeting on Substrate Integrated Microelectrode Arrays. doi: 10.3389/conf.fncel.2018.38.00020 Copyright: The abstracts in this collection have not been subject to any Frontiers peer review or checks, and are not endorsed by Frontiers. They are made available through the Frontiers publishing platform as a service to conference organizers and presenters. The copyright in the individual abstracts is owned by the author of each abstract or his/her employer unless otherwise stated. Each abstract, as well as the collection of abstracts, are published under a Creative Commons CC-BY 4.0 (attribution) licence (https://creativecommons.org/licenses/by/4.0/) and may thus be reproduced, translated, adapted and be the subject of derivative works provided the authors and Frontiers are attributed. For Frontiers’ terms and conditions please see https://www.frontiersin.org/legal/terms-and-conditions. Received: 18 Mar 2018; Published Online: 17 Jan 2019. * Correspondence: Dr. Jarno M. A. Tanskanen, Tampere University of Technology, BioMediTech Institute and Faculty of Biomedical Sciences and Engineering, Tampere, FI-33520, Finland, jarno.m.tanskanen@tut.fi Login Required This action requires you to be registered with Frontiers and logged in. To register or login click here. Abstract Info Abstract The Authors in Frontiers Jarno M. A. Tanskanen Fikret Emre Kapucu Jari A. K. Hyttinen Google Jarno M. A. Tanskanen Fikret Emre Kapucu Jari A. K. Hyttinen Google Scholar Jarno M. A. Tanskanen Fikret Emre Kapucu Jari A. K. Hyttinen PubMed Jarno M. A. Tanskanen Fikret Emre Kapucu Jari A. K. Hyttinen Related Article in Frontiers Google Scholar PubMed Abstract Close Back to top Javascript is disabled. Please enable Javascript in your browser settings in order to see all the content on this page.
更多查看译文
关键词
other dynamic neuronal cultures,mea signal analysis methods,stem,cell-derived
AI 理解论文
溯源树
样例
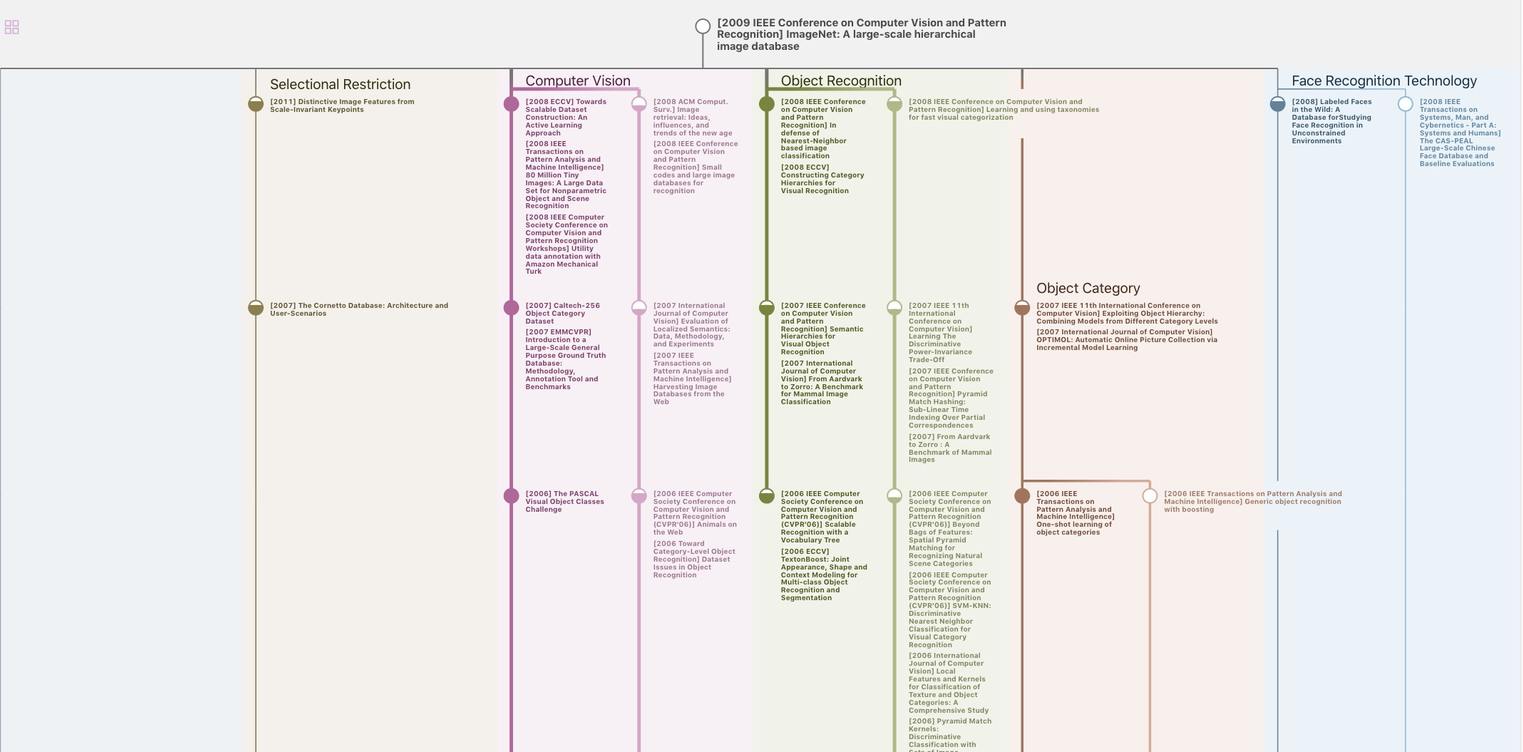
生成溯源树,研究论文发展脉络
Chat Paper
正在生成论文摘要