A novel many-objective evolutionary algorithm based on transfer matrix with Kriging model
Information Sciences(2020)
摘要
Intuitively, the main novelties of the proposed Tk-MaOEA can be presented as follows.•In the global space optimization level, a new transfer learning approach is developed to reduce a large number of objectives while the original property of the problem is kept well. The proposed approach uses a specific transfer matrix to compress the search space, which is simple yet effective to handle with the curse of dimensionality in MaOPs.•In the objective optimization level, the Kriging model is devised for each objective to further reduce its computational cost. This Bayesian based surrogate model is to measure not only the objective value itself but also stochastic error of the approximation, which is essentially conductive to improve the accuracy of the optimization.•The multi-scale normalization approach is employed so as to avoid the distortion caused by conventional normalization in the high-dimensional objective space. This is a significant operation for the MaOEA to keep unchanged spatial distribution when the original population are normalized.•The FCS approach is incorporated instead of the traditional crowding distance method in the environmental selection. This approach is more effective to select a set of representative non-dominated solutions in a single run.
更多查看译文
关键词
Evolutionary algorithm,Many-objective optimization,Transfer matrix,Kring model
AI 理解论文
溯源树
样例
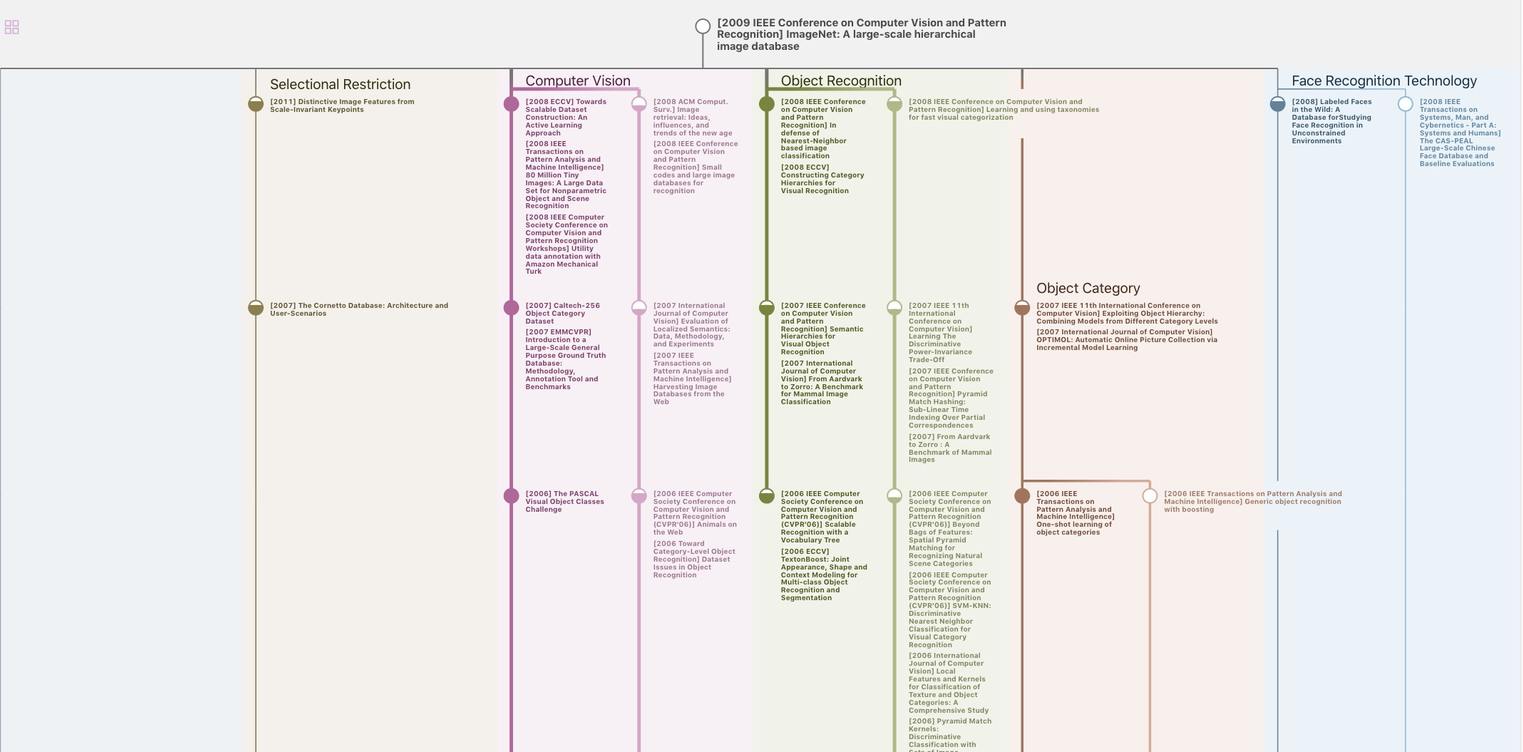
生成溯源树,研究论文发展脉络
Chat Paper
正在生成论文摘要