Convolutional neural network-based inspection of metal additive manufacturing parts
RAPID PROTOTYPING JOURNAL(2019)
摘要
Purpose Part quality inspection is playing a critical role in the metal additive manufacturing (AM) industry. It produces a part quality analysis report which can be adopted to further improve the overall part quality. However, the part quality inspection process puts heavy reliance on the engineer's background and experience. This manual process suffers from both low efficiency and potential errors and, therefore, cannot meet the requirement of real-time detection. The purpose of this paper is to look into a deep neural network, Convolutional Neural Network (CNN), towards a robust method for online monitoring of AM parts. Design/methodology/approach The proposed online monitoring method relies on a deep CNN that takes a real metal AM part's images as inputs and the part quality categories as network outputs. The authors validate the efficacy of the proposed methodology by recognizing the "beautiful-weld" category from material CoCrMo top surface images. The images of "beautiful-weld" parts that show even hatch lines and appropriate overlaps indicate a good quality of an AM part. Findings The classification accuracy of the developed method using limited information of a small local block of an image is 82 per cent. The classification accuracy using the full image and the ensemble of model outputs is 100 per cent. Originality/value A real-world data set of high resolution images of ASTM F75 I CoCrMo-based three-dimensional printed parts (Top surface images with magnification 63x) annotated with categories labels. Development of a CNN-based classification model for the supervised learning task of recognizing a "beautiful-weld" AM parts. The classification accuracy using the full image and the ensemble of model outputs is 100 per cent.
更多查看译文
关键词
Engineering,Tests
AI 理解论文
溯源树
样例
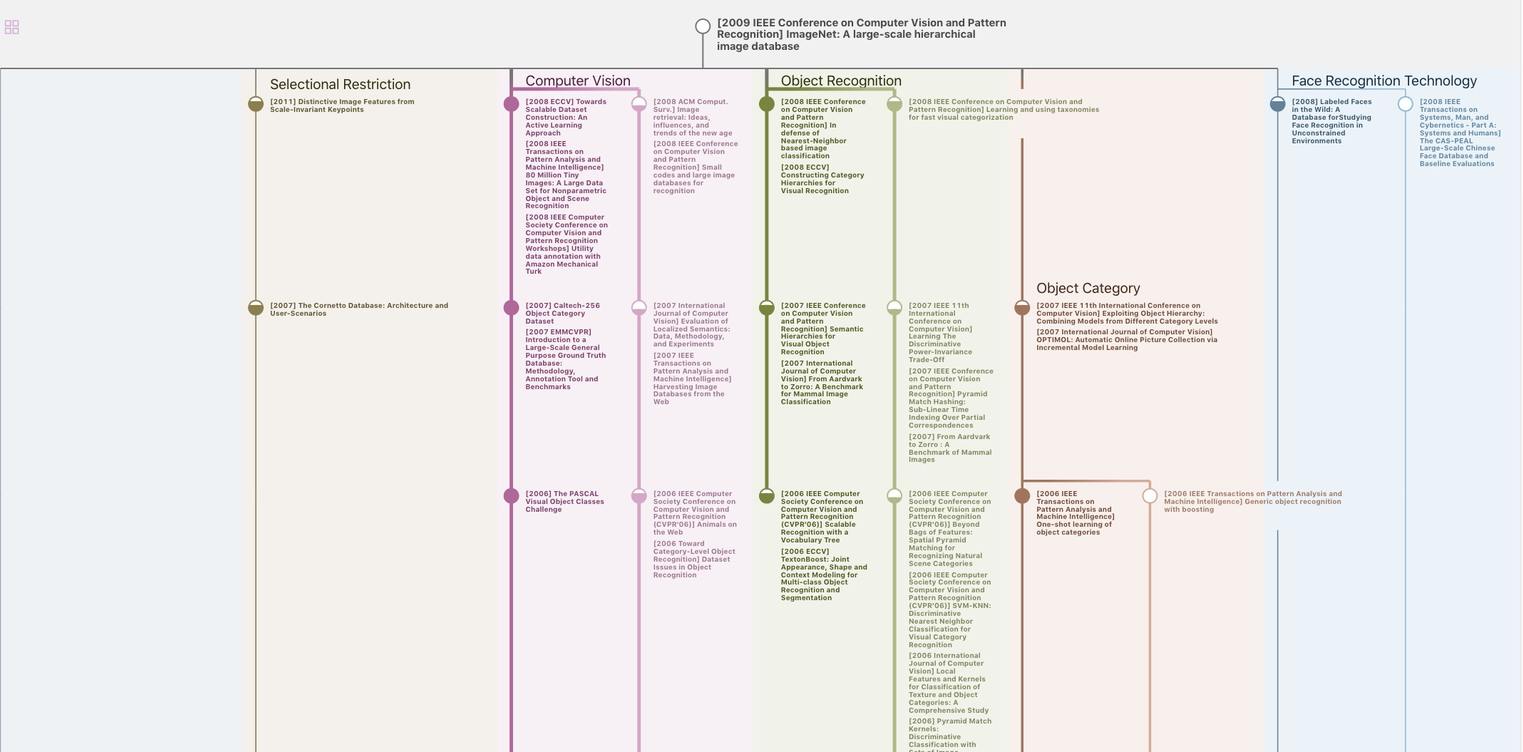
生成溯源树,研究论文发展脉络
Chat Paper
正在生成论文摘要