A decomposition-based ant colony optimization algorithm for the multi-objective community detection
Journal of Ambient Intelligence and Humanized Computing(2019)
摘要
Community structure is an important feature of complex network and detecting community can help us understand the function of networks very well. Community detection can be considered as a multi-objective optimization problem and the heuristic operators have shown promising results in dealing with this problem. In this paper, a multi-objective community detection algorithm named MOCD-ACO is proposed by combining the heuristic operator of ant colony optimization (ACO) and the multi-objective evolutionary algorithmbased on decomposition (MOEA/D). MOCD-ACO can simultaneously decompose two objective functions, i.e., Negative Ratio Association and Ratio Cut, into a number of single-objective optimization problems. Each ant is responsible for searching for a solution to a sub-problem. All ants are divided into some groups, each group sharing a pheromone matrix. The ants use pseudo-random probability selection models to construct solutions. An ant updates its current solution if it has found a better one in terms of its own objective. To make the algorithm not easy to fall into the local optimal solution, the weighted simulated annealing local search operator is integrated into the framework to expand the search range. In the experiments, synthetic network datasets and real network datasets are used to evaluate the performance of MOCD-ACO. Compared with five state-of-the-art methods, our algorithm proves to be effective in terms of normalized mutual information and modularity.
更多查看译文
关键词
Ant colony optimization, Decompose, Multi-objective optimization problems, Community detection, Complex network
AI 理解论文
溯源树
样例
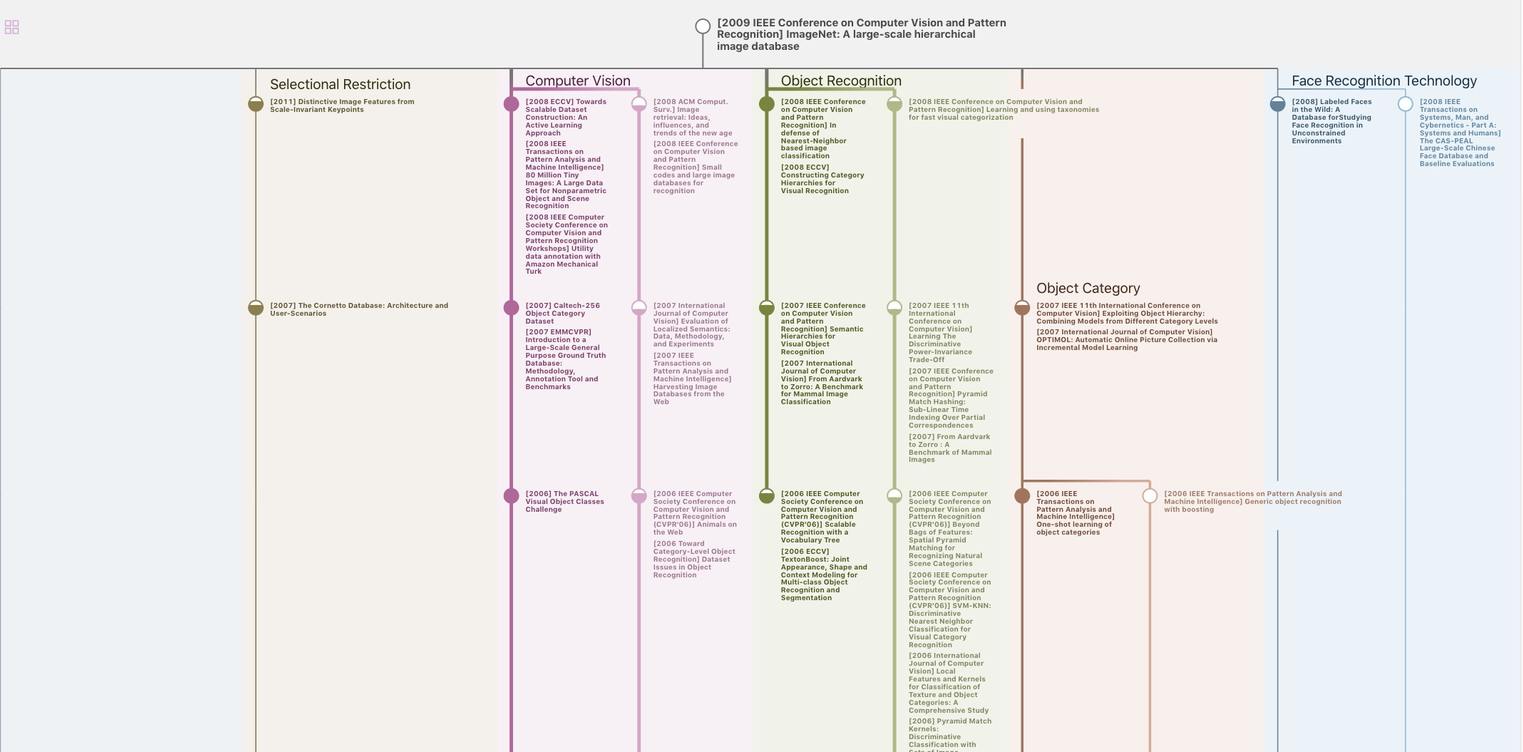
生成溯源树,研究论文发展脉络
Chat Paper
正在生成论文摘要