The multidimensional nature of aphasia: Using factors beyond language to predict treatment outcomes
Frontiers in Human Neuroscience(2017)
摘要
Event Abstract Back to Event The multidimensional nature of aphasia: Using factors beyond language to predict treatment outcomes Natalie Gilmore1*, Jeffrey P. Johnson1, Erin L. Meier1, Carrie A. Des Roches1 and Swathi Kiran1 1 Boston University, Speech, Language and Hearing Sciences, United States Background/Aims Despite the traditional view of aphasia as a language disorder, research has shown that general cognition (e.g., van de Sandt-Koenderman et al., 2008) and specific cognitive skills (e.g., Dignam et al., 2017) can predict response to aphasia rehabilitation. To investigate the relationship between cognition and treatment outcomes, two retrospective analyses were conducted to: 1) determine whether baseline cognitive/linguistic abilities and/or demographic variables predicted language treatment response; and 2) ascertain which specific cognitive measures predicted anomia treatment response. Methods/Results The first analysis included 64 persons with aphasia (PWA) (41 male; age range=26-86 years, M=60.1, SD=12.6; months post onset (MPO) range=5-67 months, M=49.3, SD=45.3) who received therapy targeting naming or sentence comprehension deficits in one of four studies. Individual proportion of potential maximal gain scores (PMG; Lambon Ralph et. al, 2010) were calculated for all participants (mean PMG=.55, SD=.35, range= -.18-1.00). Baseline cognitive/linguistic status was measured via the Western Aphasia Battery-Revised (WAB-R; Kertesz, 2007), Cognitive-Linguistic Quick Test (CLQT; Helm-Estabrooks, 2001), Pyramids and Palm Trees Test (PAPT; Howard & Patterson, 1992) and Boston Naming Test (BNT; Goodglass et al., 2001). A principal components analysis indicated that scores on the aforementioned assessments distinctly loaded onto either a language component (L.Comp) or a cognitive component (C.Comp). Individual L.Comp and C.Comp scores were computed and entered into a backward elimination regression model with age and MPO to predict treatment response as indicated by PMG. The best-fit model was significant and explained 41.08% of the variance in PMG (F(2,61)=22.96, p
更多查看译文
关键词
aphasia
AI 理解论文
溯源树
样例
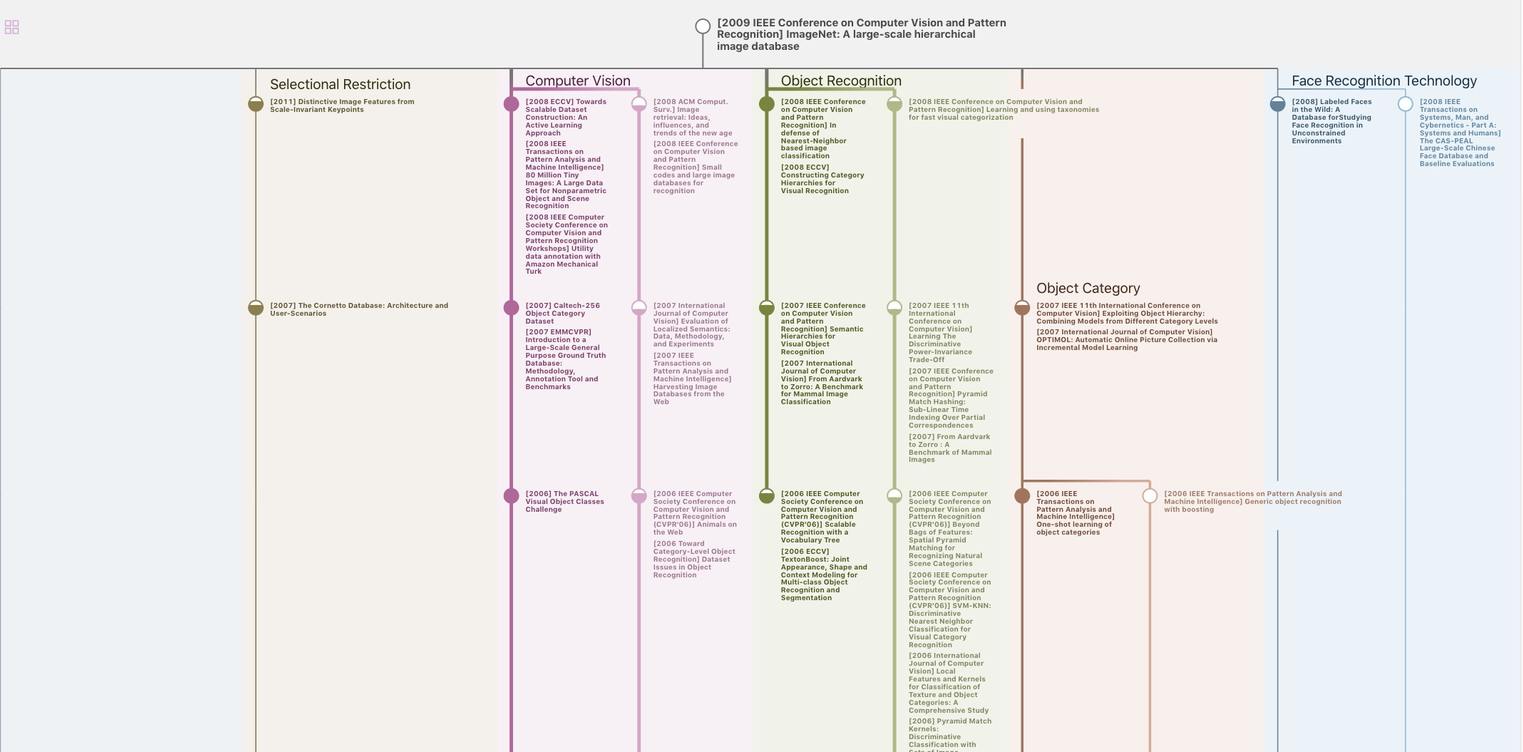
生成溯源树,研究论文发展脉络
Chat Paper
正在生成论文摘要