Sparse residual tree and forest.
arXiv: Numerical Analysis(2019)
Abstract
Sparse residual tree (SRT) is an adaptive exploration method for multivariate scattered data approximation. It leads to sparse and stable approximations in areas where the data is sufficient or redundant, and points out the possible local regions where data refinement is needed. Sparse residual forest (SRF) is a combination of SRT predictors to further improve the approximation accuracy and stability according to the error characteristics of SRTs. The hierarchical parallel SRT algorithm is based on both tree decomposition and adaptive radial basis function (RBF) explorations, whereby for each child a sparse and proper RBF refinement is added to the approximation by minimizing the norm of the residual inherited from its parent. The convergence results are established for both SRTs and SRFs. The worst case time complexity of SRTs is $mathcal{O}(Nlog_2N)$ for the initial work and $mathcal{O}(log_2N)$ for each prediction, meanwhile, the worst case storage requirement is $mathcal{O}(Nlog_2N)$, where the $N$ data points can be arbitrary distributed. Numerical experiments are performed for several illustrative examples.
MoreTranslated text
AI Read Science
Must-Reading Tree
Example
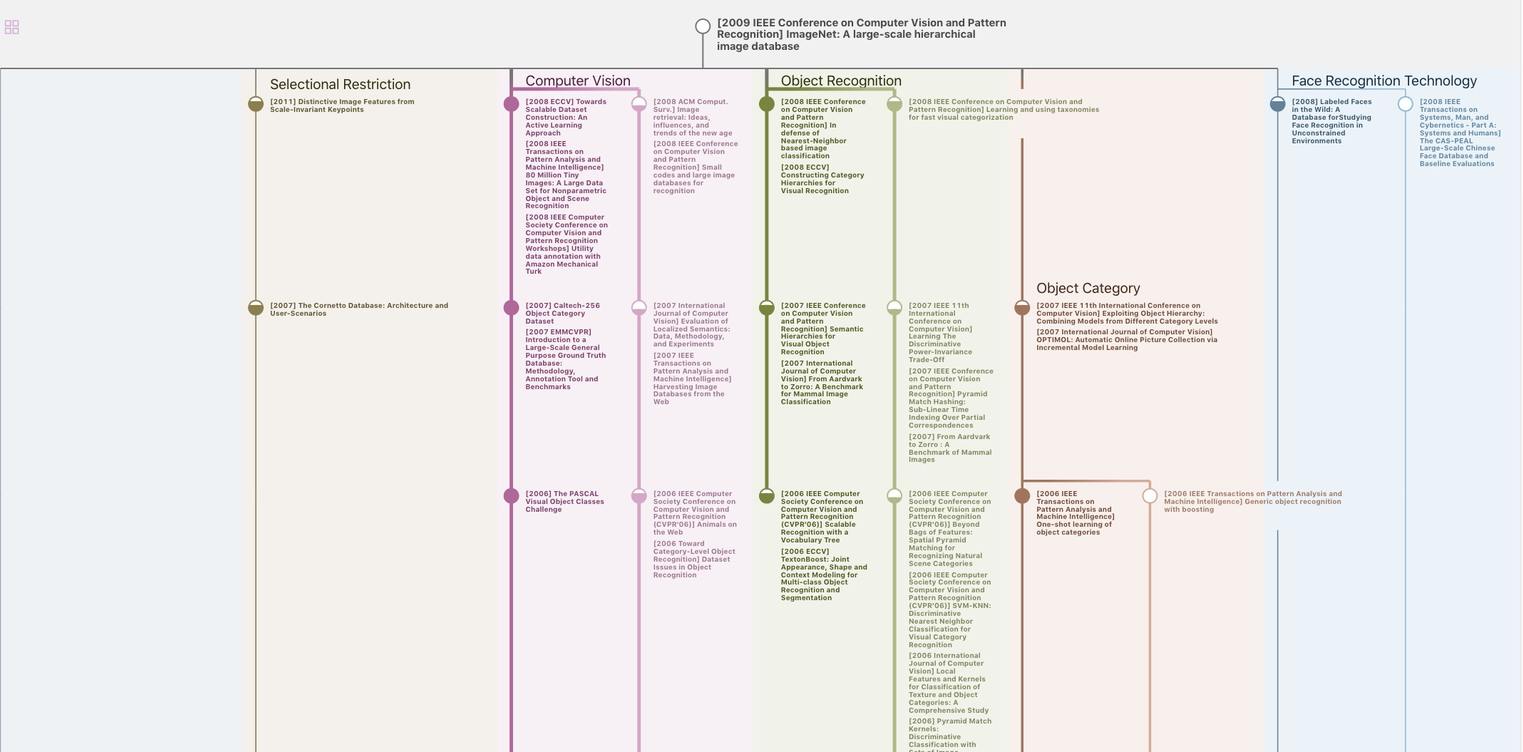
Generate MRT to find the research sequence of this paper
Chat Paper
Summary is being generated by the instructions you defined