Change-point detection in a linear model by adaptive fused quantile method.
arXiv: Statistics Theory(2019)
摘要
A novel approach to quantile estimation in multivariate linear regression models with change-points is proposed: the change-point detection and the model estimation are both performed automatically, by adopting either the quantile fused penalty or the adaptive version of the quantile fused penalty. These two methods combine the idea of the check function used for the quantile estimation and the $L_1$ penalization principle known from the signal processing and, unlike some standard approaches, the presented methods go beyond typical assumptions usually required for the model errors, such as sub-Gaussian or Normal distribution. They can effectively handle heavy-tailed random error distributions, and, in general, they offer a more complex view on the data as one can obtain any conditional quantile of the target distribution, not just the conditional mean. The consistency of detection is proved and proper convergence rates for the parameter estimates are derived. The empirical performance is investigated via an extensive comparative simulation study and practical utilization is demonstrated using a real data example.
更多查看译文
关键词
adaptive fused penalty, change-point detection, conditional quantiles, convergence rate, estimation
AI 理解论文
溯源树
样例
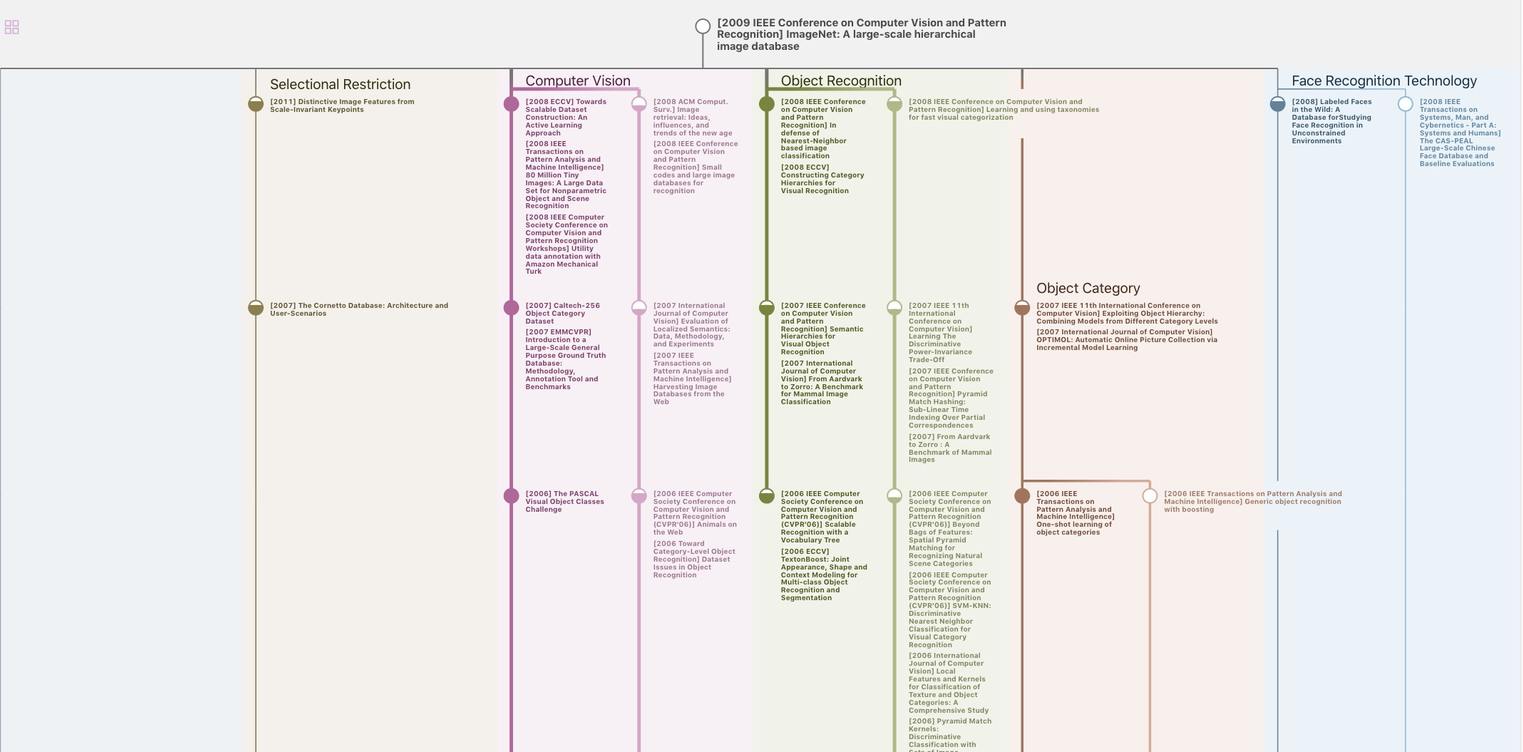
生成溯源树,研究论文发展脉络
Chat Paper
正在生成论文摘要