Pumpout: A Meta Approach to Robust Deep Learning with Noisy Labels.
arXiv: Learning(2019)
摘要
Recent studies reveal that deep neural networks gradually memorize individual data while fitting distributions of data. Hence, when facing noisy labels, all existing methods inevitably suffer from generalization degeneration and have to be early stopped. In this paper, we propose Pumpout as a meta approach to learning with noisy labels and an alternative to early stopping. Pumpout comes from sample selection and goes beyond: in every mini-batch, it uses gradient decent on good data, while it uses scaled gradient ascent on bad data rather than drops those data, where the goodness and badness are w.r.t. a base learning method. It is advantageous over early stopping, since it can continue to fit distributions of data and it has the ability of actively forgetting individual data that is memorized by mistakes. We demonstrate via experiments that Pumpout robustifies two representative base learning methods, and the performance boost is often significant.
更多查看译文
AI 理解论文
溯源树
样例
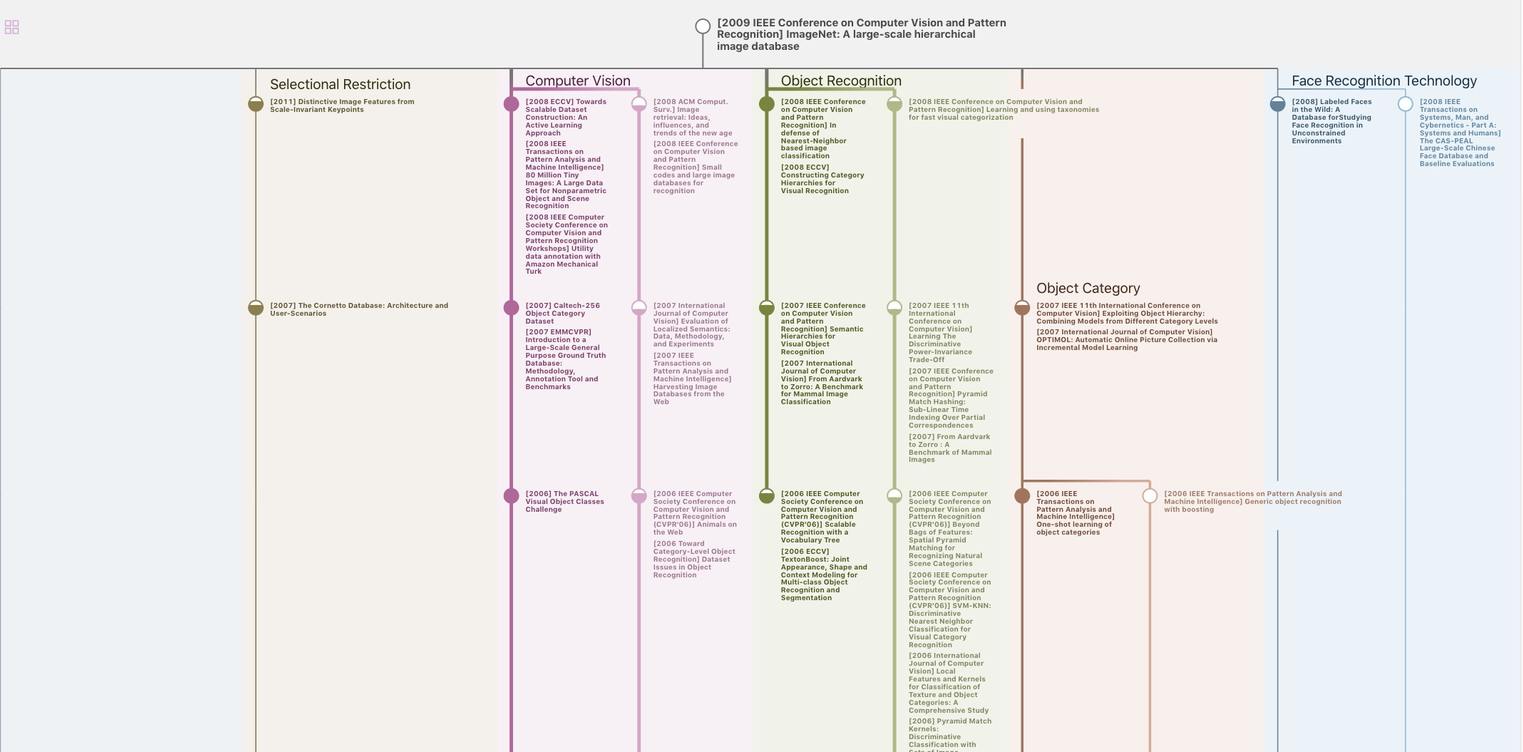
生成溯源树,研究论文发展脉络
Chat Paper
正在生成论文摘要