A Novel Bearing Fault Diagnosis Method Based On 2d Image Representation And Transfer Learning-Convolutional Neural Network
MEASUREMENT SCIENCE AND TECHNOLOGY(2019)
摘要
Traditional methods used for intelligent condition monitoring and diagnosis significantly depend on manual feature extraction and selection. To address this issue, a transfer learning-convolutional neural network (TLCNN) based on AlexNet is proposed for bearing fault diagnosis. Firstly, a 2D image representation method converts vibration signals to 2D timefrequency images. Secondly, the proposed TLCNN model extracts the features of the 2D time-frequency images and achieves the classification conditions of the bearing, which is faster to train and more accurate. Thirdly, t-distributed stochastic neighbor embedding (t-SNE) is applied to visualize the feature learning process to demonstrate the feature learning ability of the proposed model. The experimental results verify that the proposed fault diagnosis model has higher accuracy and has much better robustness against noise than other deep learning and traditional methods.
更多查看译文
关键词
fault diagnosis, transfer learning, convolutional neural network, 2D image representation, bearing
AI 理解论文
溯源树
样例
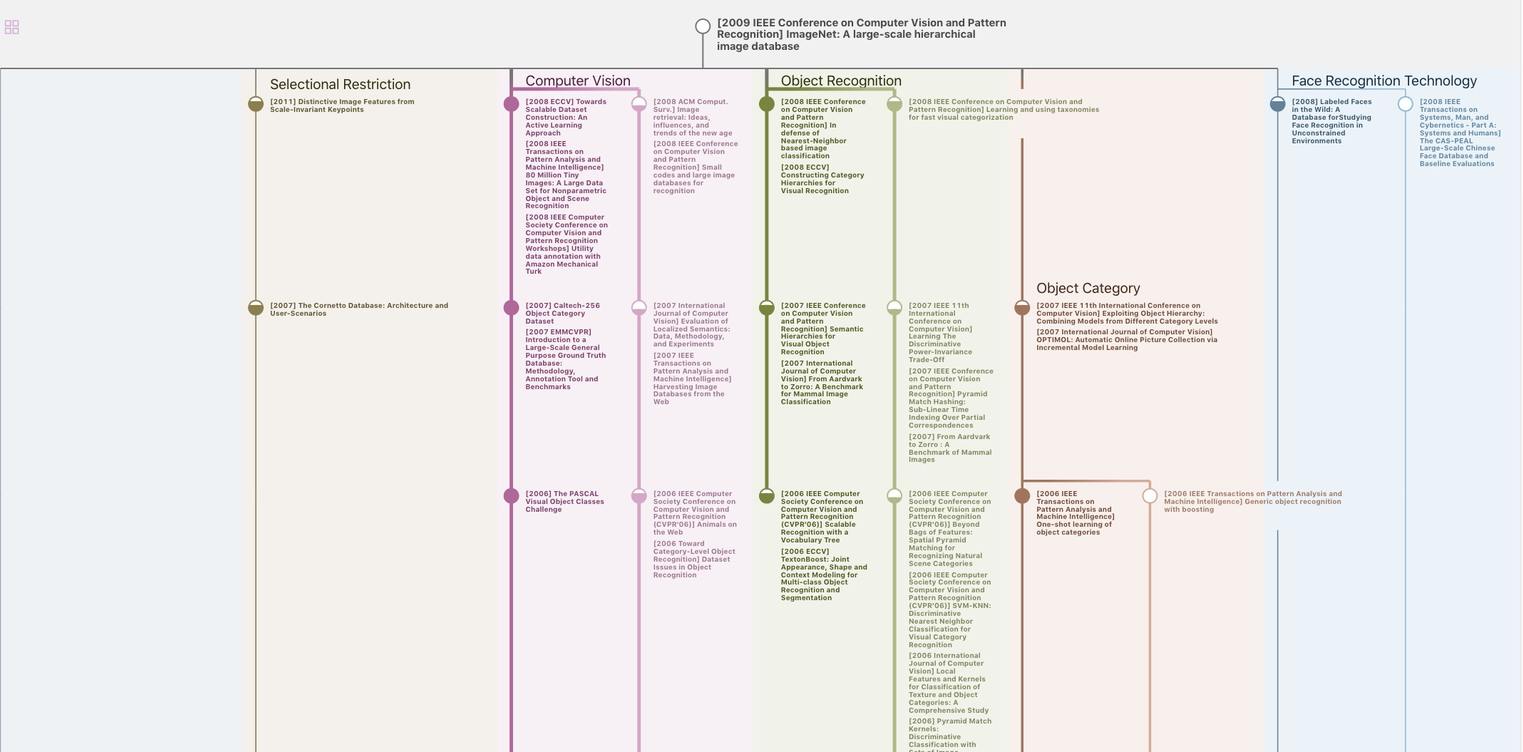
生成溯源树,研究论文发展脉络
Chat Paper
正在生成论文摘要