Field Dynamics Inference For Local And Causal Interactions
ANNALEN DER PHYSIK(2021)
摘要
Inference of fields defined in space and time from observational data is a core discipline in many scientific areas. This work approaches the problem in a Bayesian framework. The proposed method is based on statistically homogeneous random fields defined in space and time and demonstrates how to reconstruct the field together with its prior correlation structure from data. The prior model of the correlation structure is described in a non-parametric fashion and solely builds on fundamental physical assumptions such as space-time homogeneity, locality, and causality. These assumptions are sufficient to successfully infer the field and its prior correlation structure from noisy and incomplete data of a single realization of the process as demonstrated via multiple numerical examples.
更多查看译文
关键词
Bayesian methods, data analysis, information theory, stochastic processes
AI 理解论文
溯源树
样例
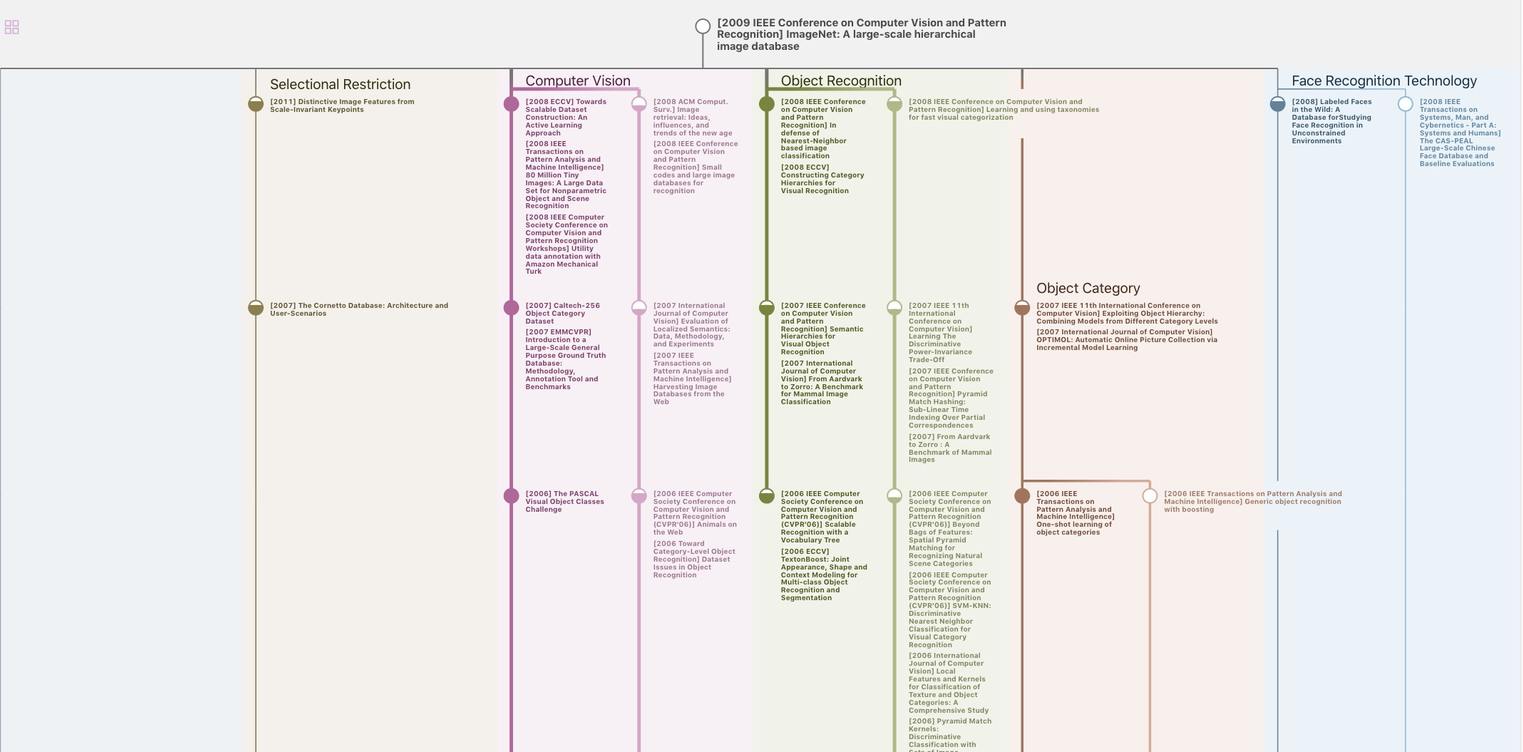
生成溯源树,研究论文发展脉络
Chat Paper
正在生成论文摘要