Data driven parallel prediction of building energy consumption using generative adversarial nets
Energy and Buildings(2019)
摘要
Building energy consumption prediction is becoming increasingly vital for energy management, equipment efficiency improvement, cooperation between building energy and power grid, and so on. But it is still a hard work to obtain accurate prediction results because of the complexity of the building energy behavior and the frequent undulations in the energy demand. In the building energy consumption prediction, the existing historical data are usually used to construct the traditional machine learning models and the deep learning models. However, compared with the data sets in the research domains of image recognition, speech processing and other fields, the data sets in the time series prediction of building energy consumption do not have a large quantity. Although the gray model can reduce the reliability on sufficient data, the model is difficult to develop, and it still needs detailed building information that may be lost in existing buildings. To overcome such issues, based on the parallel learning theory, we propose the parallel prediction scheme for the building energy consumption using Generative Adversarial Nets (GAN). The parallel prediction firstly makes use of a small number of the original data series to generate the parallel data via GAN, and then forms the mixed data set which includes the original data and the artificial data, and finally utilizes the mixed data to train the prediction models. To verify the proposed parallel prediction method, two experiments which adopts different kinds of data sets from two real-world buildings are conducted. In each experiment, the availability of the parallel data and the rationality of the parallel prediction model are evaluated, and detailed comparisons are made. Experimental results show that the parallel data have similar distributions to the original data, and the prediction models trained by the mixed data perform better than those trained only using the original data. Comparison results demonstrated that the proposed method performs best compared with the existing methods such as the information diffusion technology (IDT), the heuristic Mega-trend-diffusion (HMTD) method and the bootstrap method. The proposed parallel prediction scheme can also be extended to other time series forecasting problems, such as the electricity load forecasting, and the traffic flow prediction.
更多查看译文
关键词
building energy consumption,deep learning,parallel prediction,Generative Adversarial Nets
AI 理解论文
溯源树
样例
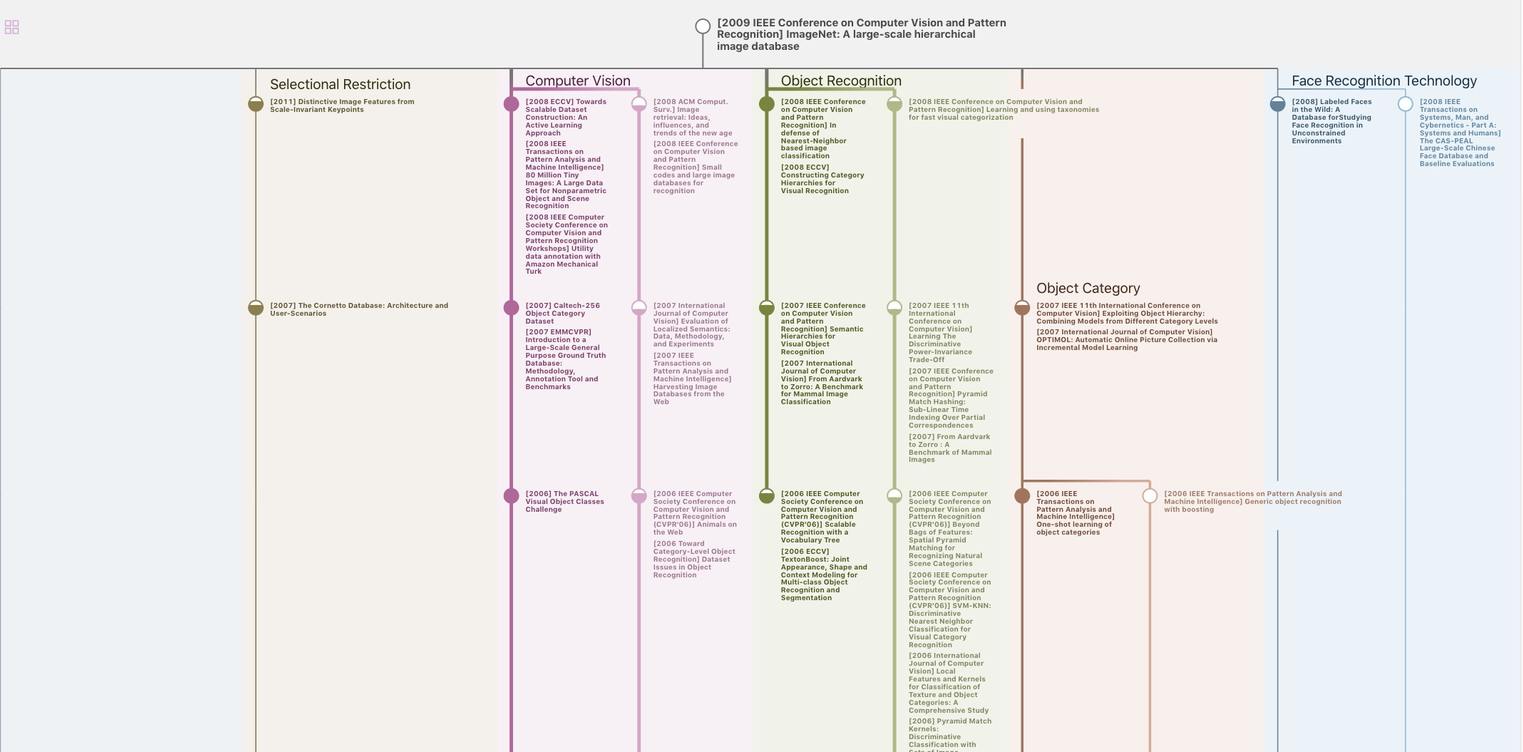
生成溯源树,研究论文发展脉络
Chat Paper
正在生成论文摘要