Location of Pulmonary Mast Cells Predicts Asthma Outcomes
Journal of Allergy and Clinical Immunology(2019)SCI 1区
Univ Pittsburgh | Dept Environm & Occupat Hlth
Abstract
Pulmonary mast cells (MC) have been associated with asthma pathogenesis. We have previously shown differences in MC phenotype by location in the lung (submucosa and epithelium) [Balzar, AJRCCM, 2011]. Little is known regarding the relative importance of luminal MCs to asthma. We hypothesized that distal/luminal MCs would better predict more severe clinical outcomes than proximal/epithelial MCs. In 102 University of Pittsburgh asthmatics enrolled in NHLBI trials, bronchial epithelial brushings, bronchoalveolar (BAL) cells and fluid were obtained at bronchoscopy. Expression of the MC protease, tryptase mRNA was determined by qRT-PCR. Using the median tryptase mRNA values for epithelial brushings and BAL cells, subjects were classified as proximal and distal MC "Hi" or "Lo." Regression analysis was performed to assess the effect of MC "Hi" on the outcomes of history of recent exacerbation, baseline FEV1% predicted and FEV1/FVC. Distal MCHi asthma significantly predicted exacerbation [OR=3.6, p=0.004], while proximal MCHi asthma did not [OR=1.7, p=0.2]. Distal MCHi asthma negatively associated with baseline FEV1% predicted [Beta= -12.5, p=0.006, R2=0.07] and baseline FEV1/FVC [Beta= -0.06, p=0.019, R2=0.05]. This relationship was not seen with proximal MCHi asthma [p for FEV1% predicted=0.6, p for FEV1/FVC=0.8]. In matched samples from asthmatic subjects, the distal/BAL cell MC signature better predicted asthma exacerbation, lower FEV1%predicted and decreased FEV1/FVC than the proximal/epithelial MC signature. In asthma, this suggests an important difference in MC function based on the location in the lung. Use of distal MC biomarkers should better identify more clinically severe asthma.
MoreTranslated text
PDF
View via Publisher
AI Read Science
AI Summary
AI Summary is the key point extracted automatically understanding the full text of the paper, including the background, methods, results, conclusions, icons and other key content, so that you can get the outline of the paper at a glance.
Example
Background
Key content
Introduction
Methods
Results
Related work
Fund
Key content
- Pretraining has recently greatly promoted the development of natural language processing (NLP)
- We show that M6 outperforms the baselines in multimodal downstream tasks, and the large M6 with 10 parameters can reach a better performance
- We propose a method called M6 that is able to process information of multiple modalities and perform both single-modal and cross-modal understanding and generation
- The model is scaled to large model with 10 billion parameters with sophisticated deployment, and the 10 -parameter M6-large is the largest pretrained model in Chinese
- Experimental results show that our proposed M6 outperforms the baseline in a number of downstream tasks concerning both single modality and multiple modalities We will continue the pretraining of extremely large models by increasing data to explore the limit of its performance
Try using models to generate summary,it takes about 60s
Must-Reading Tree
Example
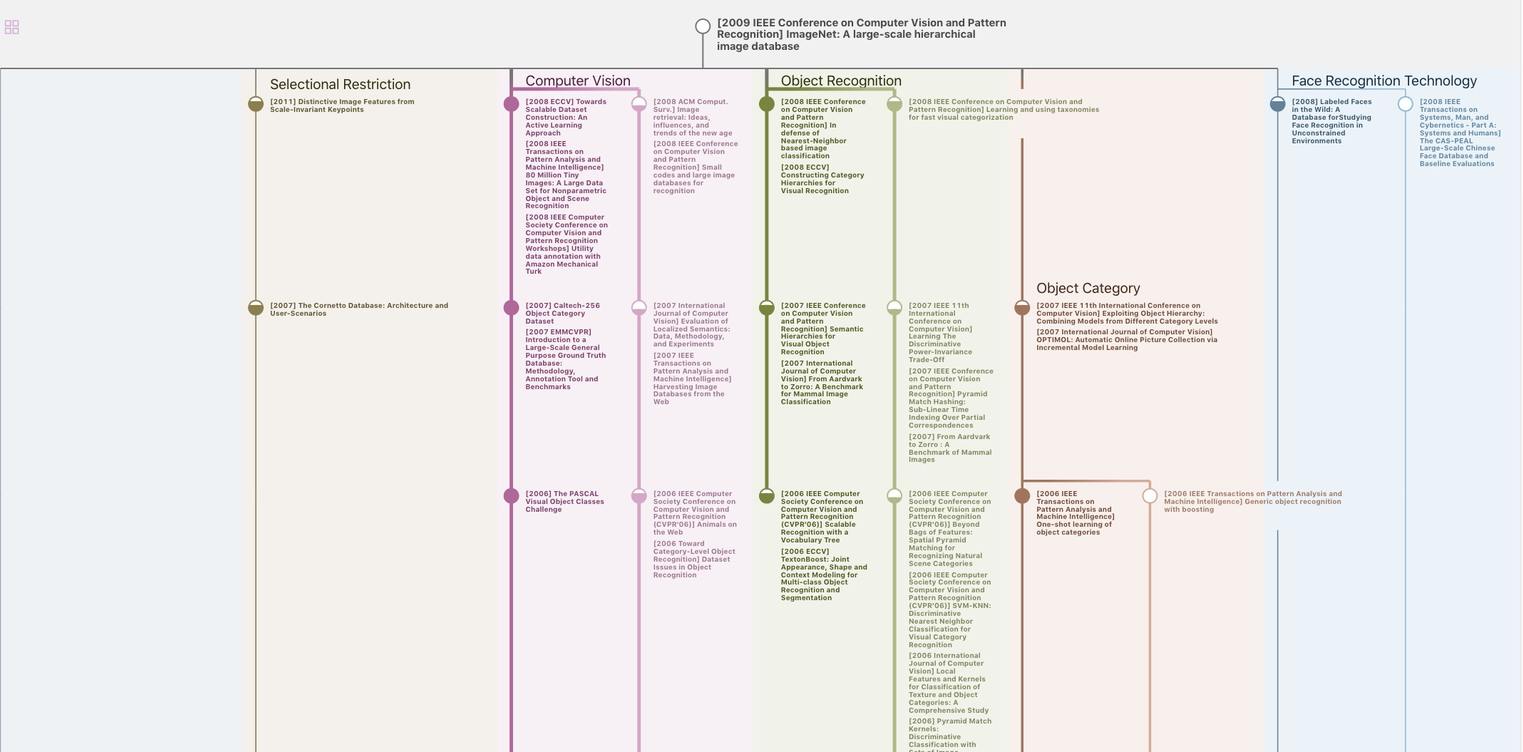
Generate MRT to find the research sequence of this paper
Data Disclaimer
The page data are from open Internet sources, cooperative publishers and automatic analysis results through AI technology. We do not make any commitments and guarantees for the validity, accuracy, correctness, reliability, completeness and timeliness of the page data. If you have any questions, please contact us by email: report@aminer.cn
Chat Paper
去 AI 文献库 对话