Comparison of Gvhd Biomarker Algorithms for Predicting Lethal Gvhd and Non-Relapse Mortality
Biology of Blood and Marrow Transplantation(2019)
摘要
Acute GVHD biomarkers predict long-term outcomes after GVHD diagnosis. Our group has validated the MAGIC algorithm which uses the concentrations of 2 GVHD biomarkers, ST2+REG3a, to predict lethal GVHD (defined as death from GVHD without relapse) [Hartwell 2017]. Other published biomarker combinations that predict GVHD outcomes include ST2+ REG3a+TNFR1 [Levine 2015], ST2+TIM3 [Abu Zaid 2017], ST2+TNFR1 [McDonald 2015], TIM3+TNFR1+IL6 [McDonald 2015], as well as AREG alone [Holtan 2018]. It is not clear which biomarker combination best predicts lethal GVHD because these algorithms were developed using different patient cohorts with different endpoints. To answer this question, we compared the predictive accuracy of different biomarker combinations as well as these six specific combinations in the same patient cohort. We studied 522 patients with serum samples at GVHD diagnosis who were transplanted at 19 Mount Sinai Acute GVHD International Consortium (MAGIC) centers between January 1, 2004 and April 30, 2017. Patients were divided into training (n = 253) and validation (n = 269) sets; validation patients were transplanted after November 1, 2015 and had not previously been used to generate an algorithm. Biomarkers were measured by ELISA and log-transformed values were used to predict 1-year lethal GVHD by competing risk regression. Four of the 6 biomarkers (ST2, REG3a, TNFR1, and TIM3) independently predicted lethal GVHD in the training set. We then developed algorithms that predicted lethal GVHD using all possible combinations of these 4 biomarkers as well as the published combination of TIM3+TNFR1+IL6 and AREG alone. The best algorithms of 1-4 biomarkers for predicting lethal GVHD were REG3a alone, ST2+REG3a, ST2+REG3a+TNFR1, and ST2+REG3a+TNFR1+TIM3. While ST2+REG3a was the most accurate combination based on the lowest Akaike Information Criterion (AIC), the other published biomarker combinations produced similar AICs. Therefore, we used the independent validation set to compare all six published algorithms. We generated area under the receiver operating characteristic curves (AUC) for each algorithm (FIG 1). We next determined the threshold that maximized sensitivity and specificity for each algorithm and calculated the cumulative incidence of lethal GVHD in the resulting high and low risk strata (FIG 2). Highly similar results were obtained when the cumulative incidence of non-relapse mortality was used as the endpoint. In a validation set of previously unanalyzed patients, several biomarker combinations reproducibly stratified patients with GVHD for risk of death. The best 4 algorithms, all of which included ST2, produced comparable outcomes. Adding TNFR1 to ST2+ REG3a did not improve accuracy. The ST2+REG3a algorithm best identifies patients at onset of GVHD for high risk of lethal GVHD and NRM, and thus remains a standard for biomarker-based risk prediction.
更多查看译文
AI 理解论文
溯源树
样例
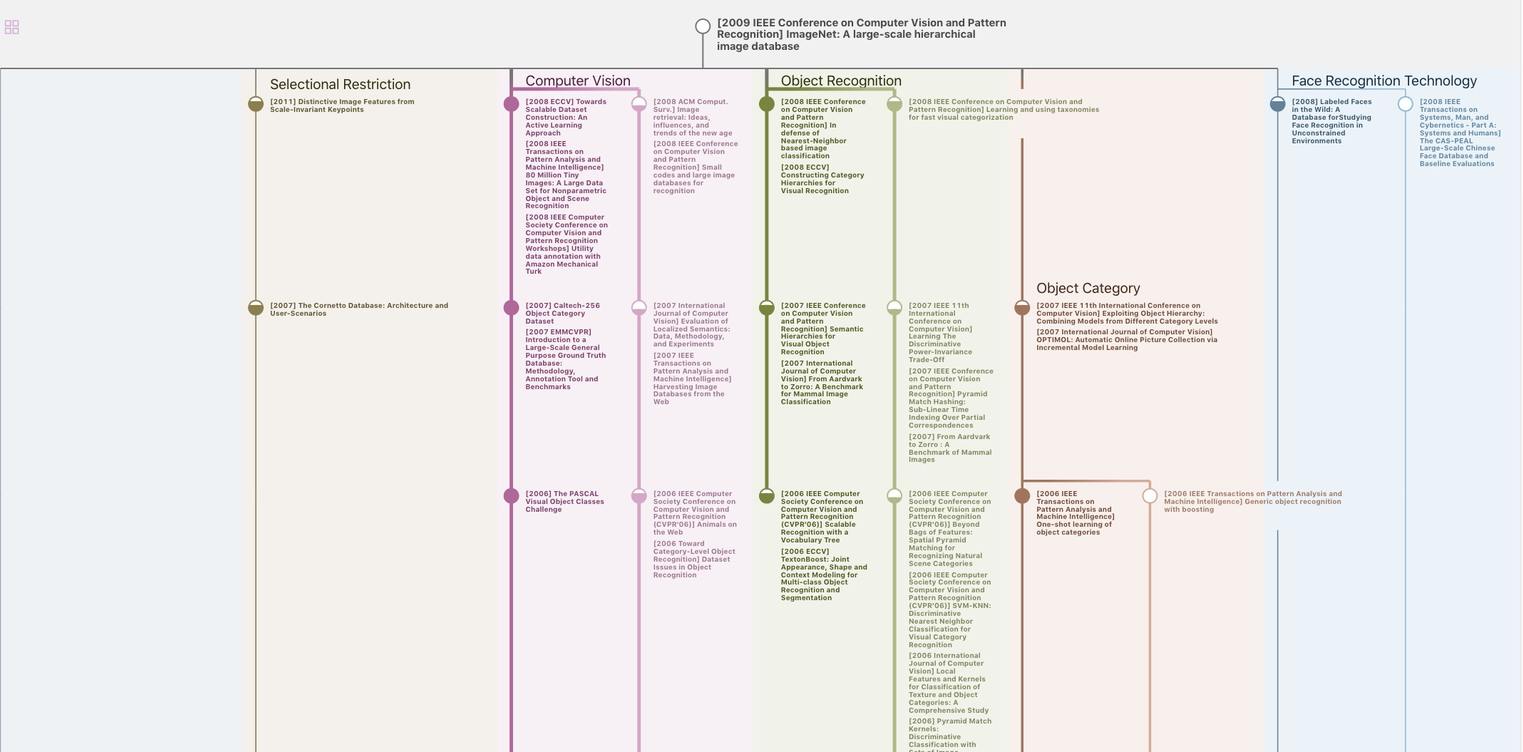
生成溯源树,研究论文发展脉络
Chat Paper
正在生成论文摘要