Self-Attention based model for de-novo antibiotic resistant gene classification with enhanced reliability for out of distribution data detection
bioRxiv(2019)
摘要
Antibiotic resistance monitoring is of paramount importance in the face of this ongoing global epidemic. Using traditional alignment based methods to detect antibiotic resistant genes results in huge number of false negatives. In this paper, we introduce a deep learning model based on a self-attention architecture that can classify antibiotic resistant genes into correct classes with high precision and recall by just using protein sequences as input. Additionally, deep learning models trained with traditional optimization algorithms (e.g. Adam, SGD) provide poor posterior estimates when tested against Out-of-Distribution (OoD) antibiotic resistant/non-resistant genes. We train our model with an optimization method called Preconditioned Stochastic Gradient Langevin Dynamics (pSGLD) which provides reliable uncertainty estimates when tested against OoD data.
更多查看译文
关键词
gene,classification,self-attention,forde-novoantibiotic
AI 理解论文
溯源树
样例
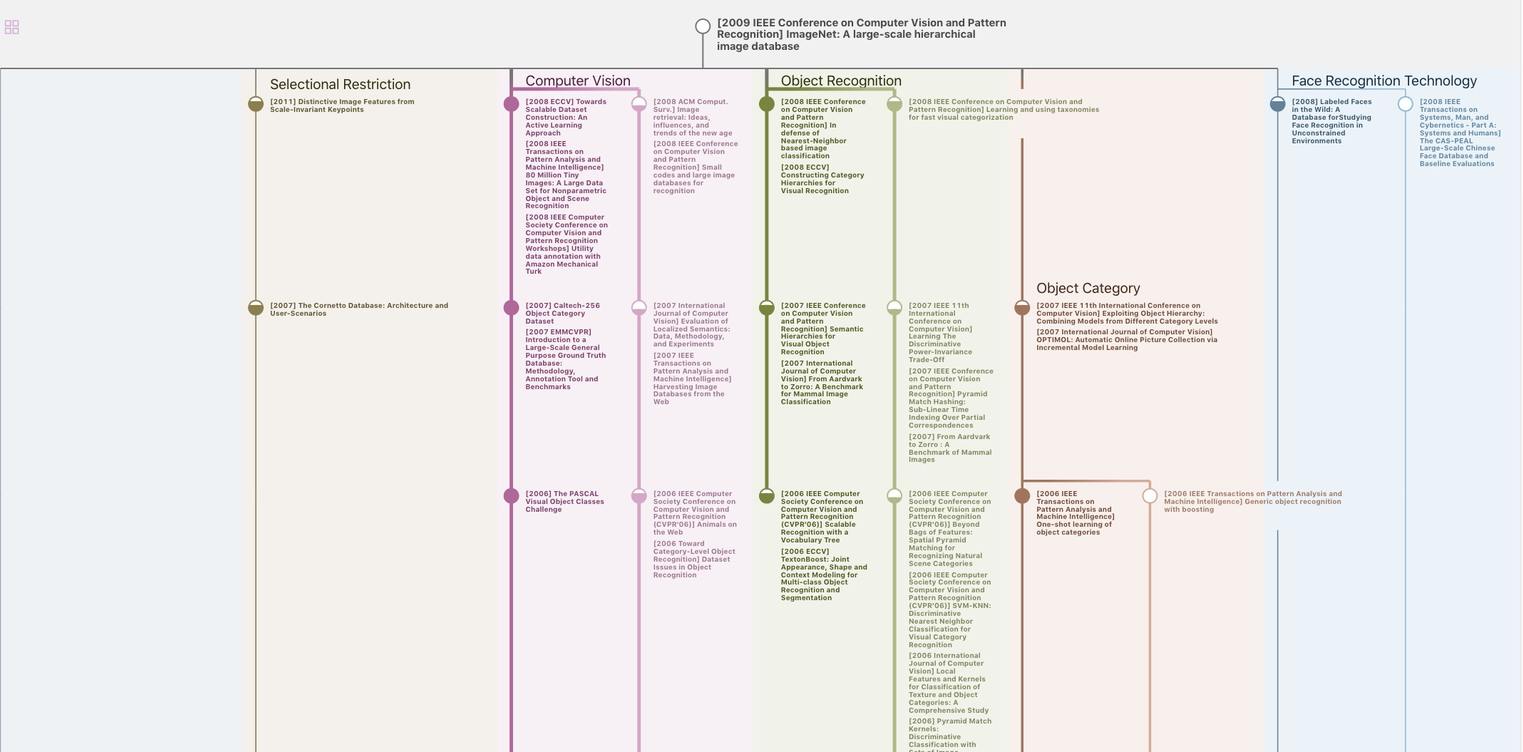
生成溯源树,研究论文发展脉络
Chat Paper
正在生成论文摘要