Predicting Seismic-Based Risk of Lost Circulation Using Machine Learning
Journal of petroleum science & engineering(2019)
摘要
Lost circulation during well drilling and completion wastes productive time, and even kills the well in severe cases. Timely identifying lost circulation events and taking countermeasures has been the focus of related study. However, a real prediction of lost circulation risk before drilling would be an active response to the challenge. In this paper, a technical solution is proposed to evaluate geological lost-circulation risk in the field using 3D seismic data attributes and machine learning technique. First, four seismic attributes (variance, attenuation, sweetness, RMS amplitude) that are the most correlated with lost circulation incidents are recommended. Then a prediction model is built by conducing supervised learning that involves a majority voting algorithm. The performance of the model is illustrated by six unseen drilled wells and shows the ability and potential to forecast lost circulation probability both along well trajectory and in the region far away from the drilled wells. The prediction resolution in the lateral and vertical direction is about 25 m and 6 m (2 ms), respectively, which are distinct advantages over the traditional description of geological structures using seismic data. It shows that the lost circulation risk can be hardly recognized by interpreting one specific seismic attribute, which is a common practice. Finally, the challenges in predicting lost circulation risk using seismic data are summarized. Overall, the study suggests that machine learning would be a practical solution to predict various construction risks that are related to seismic-based geological issues. Knowing in advance the risks, people could avoid or at least minimize the losses by optimizing well deployment in the field and taking preventive measures.
更多查看译文
关键词
Machine learning,Lost circulation,Seismic attribute,Mud loss,Seismic interpretation
AI 理解论文
溯源树
样例
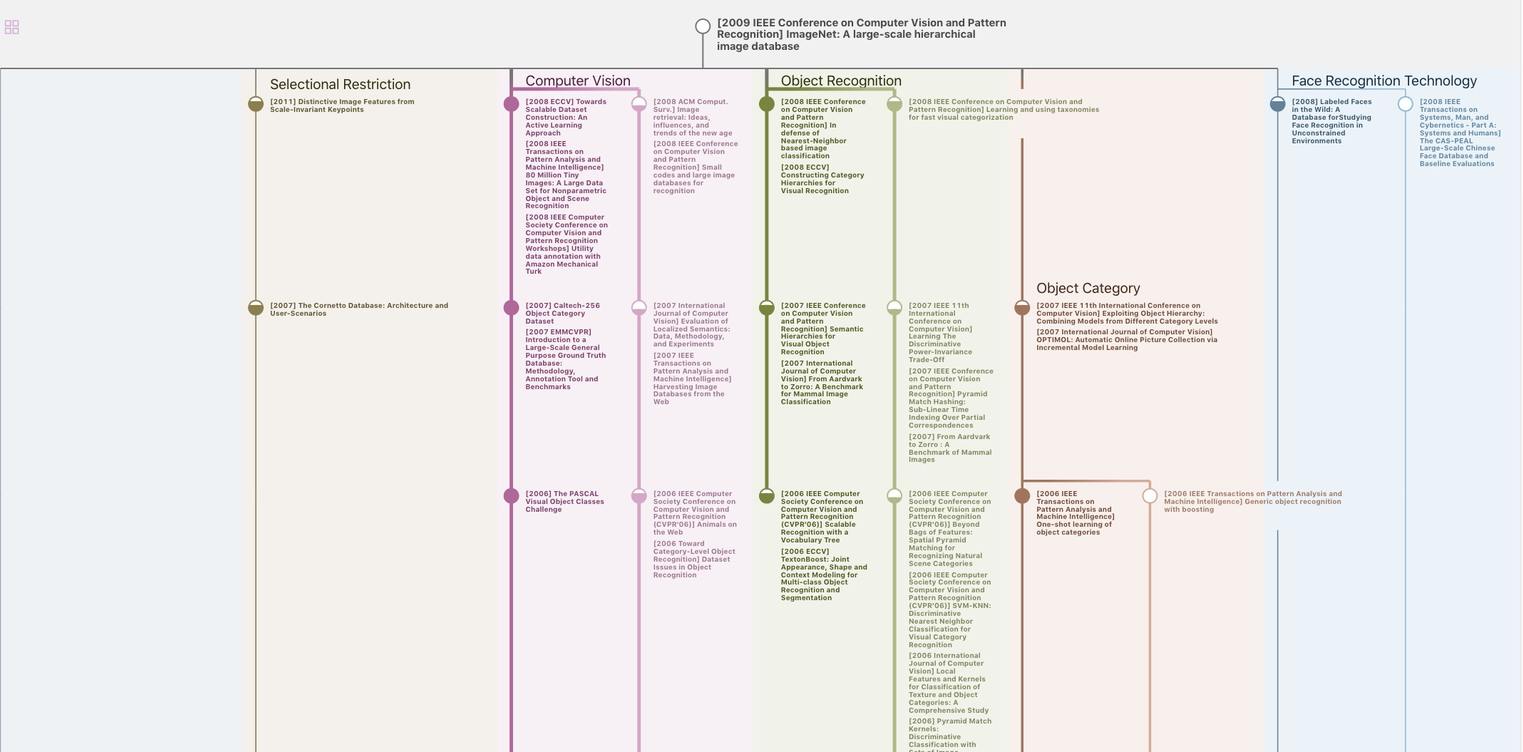
生成溯源树,研究论文发展脉络
Chat Paper
正在生成论文摘要