A collaborative filtering recommendation algorithm based on information theory and bi-clustering
Neural Computing and Applications(2019)
Abstract
Collaborative filtering is the most popular and efficient recommendation algorithm to character the potential preference of the new users, by exploring the patterns of historical consuming records/ratings of the investigated users. There are two types of primary collaborative filtering algorithms: the user-based recommendation system, which recommends items to new users by ranking the similarity of the shared items between the history users and the new users, and the item-based collaborative filtering recommend items to new users by considering the rank of the similarity among all the history items of the training data. Although the collaborative filtering has been successfully applied to many commercial fields, several original drawbacks of collaborative filtering, especially the sparsity of the rating data raises a serious challenge to the accuracy and the universality of those algorithms. In particular, the most rating terms for each specific user are missing in many applications, and the performance of collaborative filtering will be degraded along with the increment of the number of items in training dataset. In this paper, we proposed a novel collaborative filtering method (CBE-CF) to extract the local dense rating modules to cope with the data sparsity and the computational efficiency of the traditional recommendation algorithms, by introducing the information entropy and bi-clustering into collaborative filtering. Here, both the rows and columns of the user-item-rating matrix are clustered together to identify the dense rating modules of the historical records (training) data, and then an information entropy metric is used to quantify the similarity between the new user and each dense modules, and the final prediction is optimized by the aggregative recommendations of the global generalization of item-based methods and the local similarity of the nearest modules. Experimental analysis presents the characters of the proposed CBE-CF, and the precision and the computational cost, etc., are better than state of the art on the benchmark dataset.
MoreTranslated text
Key words
Collaborative filtering,Information entropy,Bi-clustering,Recommendation system
AI Read Science
Must-Reading Tree
Example
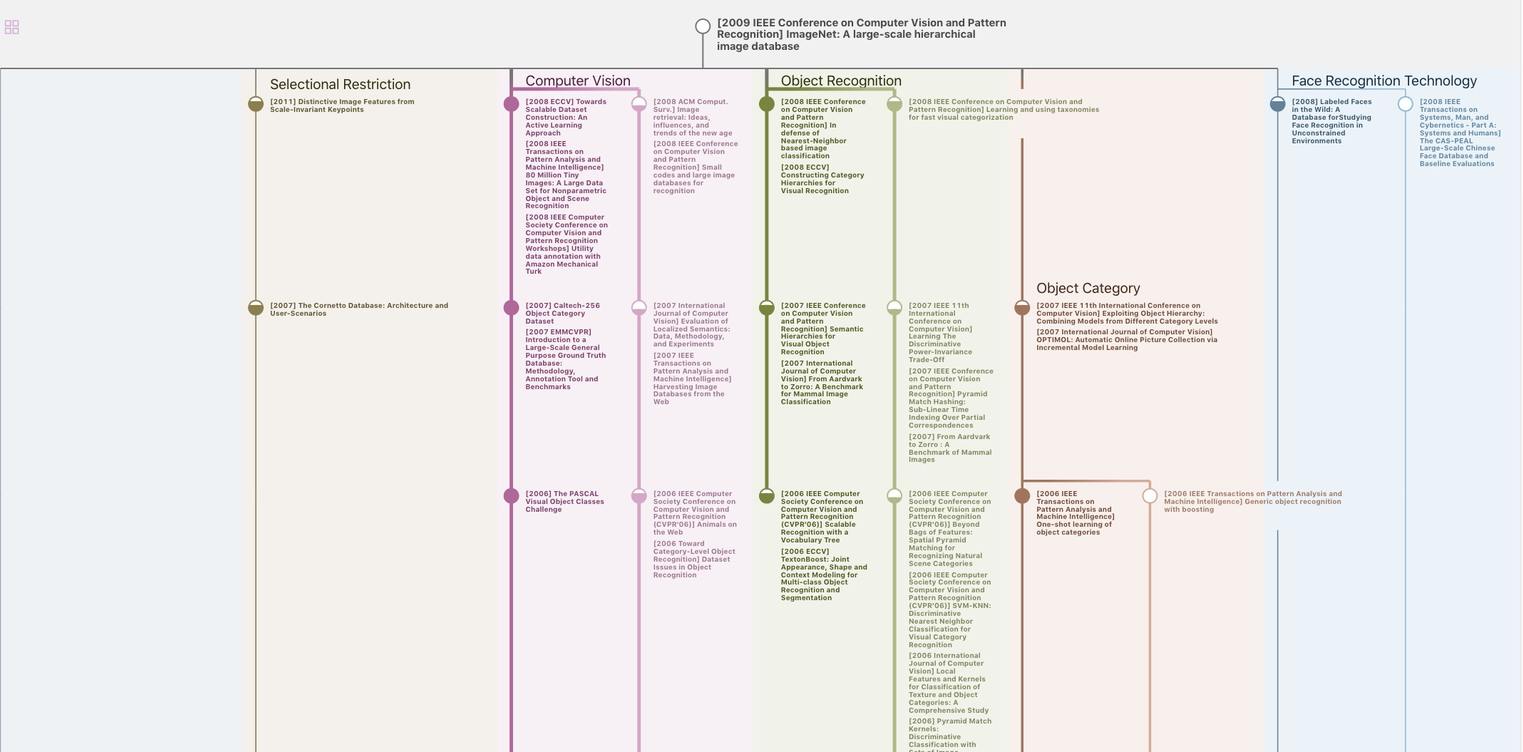
Generate MRT to find the research sequence of this paper
Chat Paper
Summary is being generated by the instructions you defined