Supervised representation learning for multi-label classification
Machine Learning(2019)
摘要
Representation learning is one of the most important aspects of multi-label learning because of the intricate nature of multi-label data. Current research on representation learning either fails to consider label knowledge or is affected by the lack of labeled data. Moreover, most of them learn the representations and incorporate the label information in a two-step manner. In this paper, due to the success of representation learning by deep learning we propose a novel framework based on neural networks named SERL to learn global feature representation by jointly considering all labels in an effective supervised manner. At its core, a two-encoding-layer autoencoder, which can utilize labeled and unlabeled data, is adopted to learn feature representation in the supervision of softmax regression. Specifically, the softmax regression incorporates label knowledge to improve the performance of both representation learning and multi-label learning by being jointly optimized with the autoencoder. Moreover, the autoencoder is expanded into two encoding layers to share knowledge with the softmax regression by sharing the second encoding weight matrix. We conduct extensive experiments on five real-world datasets to demonstrate the superiority of SERL over other state-of-the-art multi-label learning approaches.
更多查看译文
关键词
Representation learning, Multi-label learning, Two-encoding-layer autoencoder
AI 理解论文
溯源树
样例
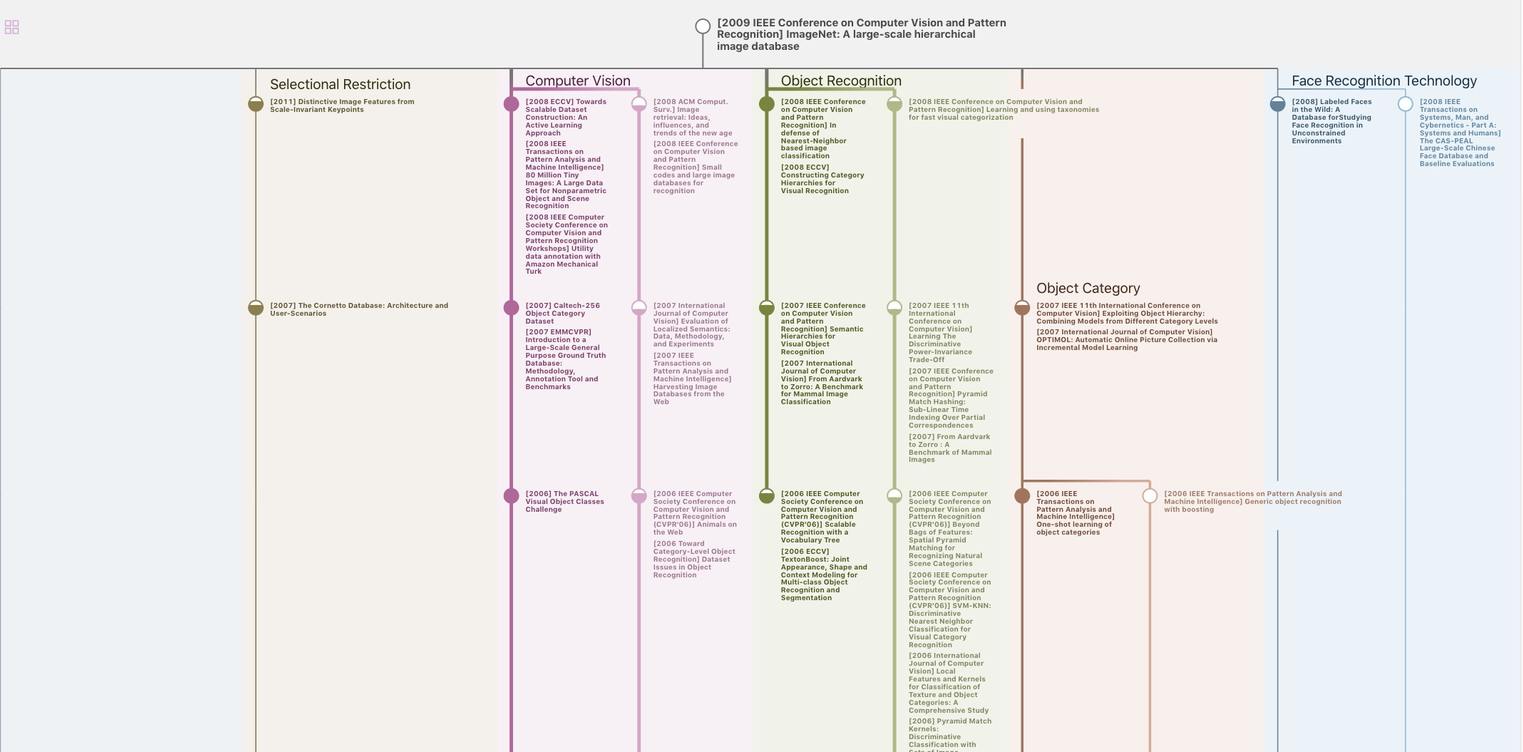
生成溯源树,研究论文发展脉络
Chat Paper
正在生成论文摘要