A Simulation Smoother For Long Memory Time Series With Correlated And Heteroskedastic Additive Noise
COMMUNICATIONS IN STATISTICS-SIMULATION AND COMPUTATION(2021)
摘要
The purpose of this paper is to extend the work of So (1999) by accommodating heteroskedasticity in long memory processes and correlation in disturbances. We propose an alternative representation of long memory processes from which we develop filtering equations, prediction densities and a simulation smoother for latent state variables as in the classical Kalman filter. We illustrate the simulation smoother introduced in this paper by estimating a class of long memory models with heteroskedastic correlated additive noises.
更多查看译文
关键词
Filtering, Kalman filter, Long Memory, State Space Models, Simulation Smoother, Stochastic Volatility
AI 理解论文
溯源树
样例
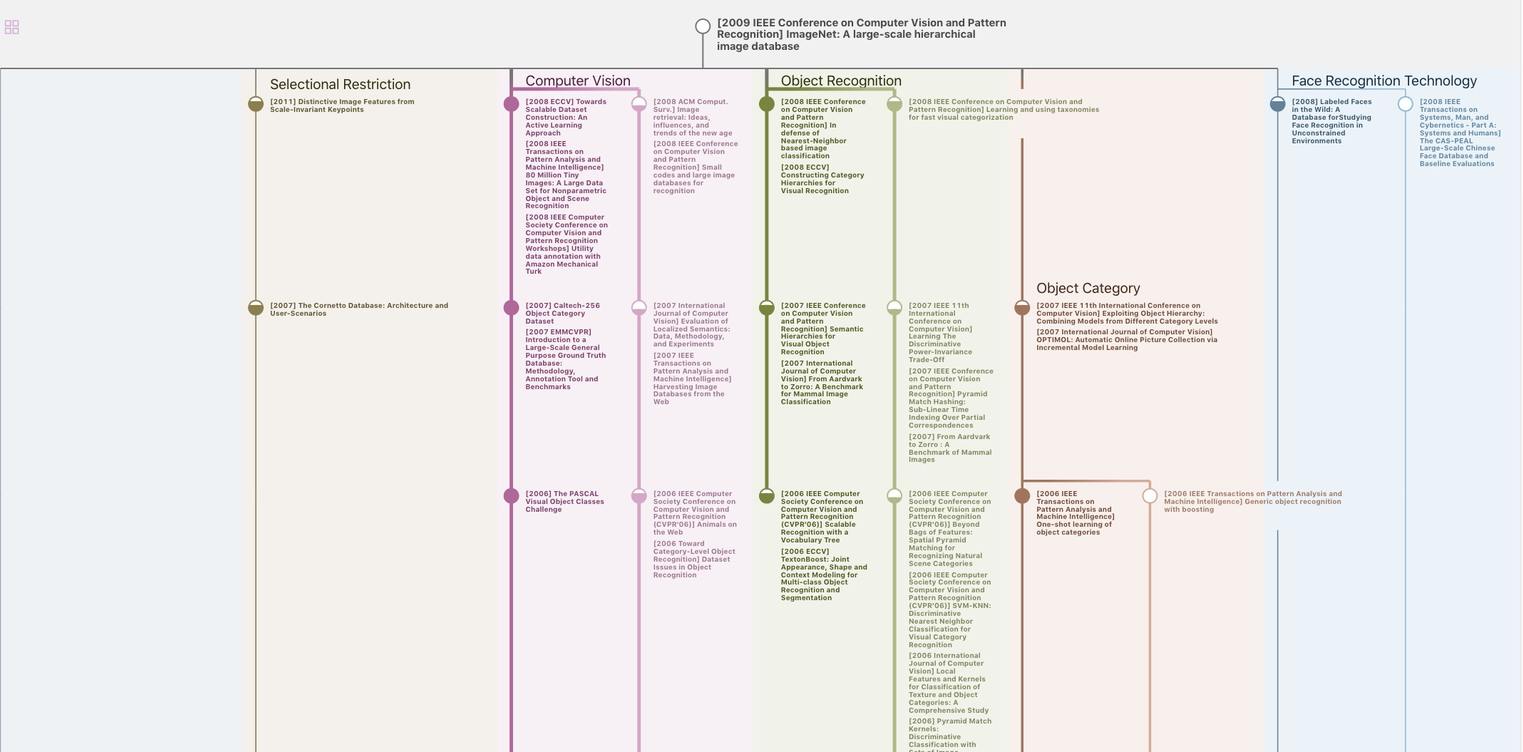
生成溯源树,研究论文发展脉络
Chat Paper
正在生成论文摘要