An Exact Penalty Method For Semidefinite-Box-Constrained Low-Rank Matrix Optimization Problems
IMA JOURNAL OF NUMERICAL ANALYSIS(2020)
摘要
This paper considers a matrix optimization problem where the objective function is continuously differentiable and the constraints involve a semidefinite-box constraint and a rank constraint. We first replace the rank constraint by adding a non-Lipschitz penalty function in the objective and prove that this penalty problem is exact with respect to the original problem. Next, for the penalty problem we present a nonmonotone proximal gradient (NPG) algorithm whose subproblem can be solved by Newton's method with globally quadratic convergence. We also prove the convergence of the NPG algorithm to a first-order stationary point of the penalty problem. Furthermore, based on the NPG algorithm, we propose an adaptive penalty method (APM) for solving the original problem. Finally, the efficiency of an APM is shown via numerical experiments for the sensor network localization problem and the nearest low-rank correlation matrix problem.
更多查看译文
关键词
rank constrained optimization, non-Lipschitz penalty, nonmonotone proximal gradient, penalty method
AI 理解论文
溯源树
样例
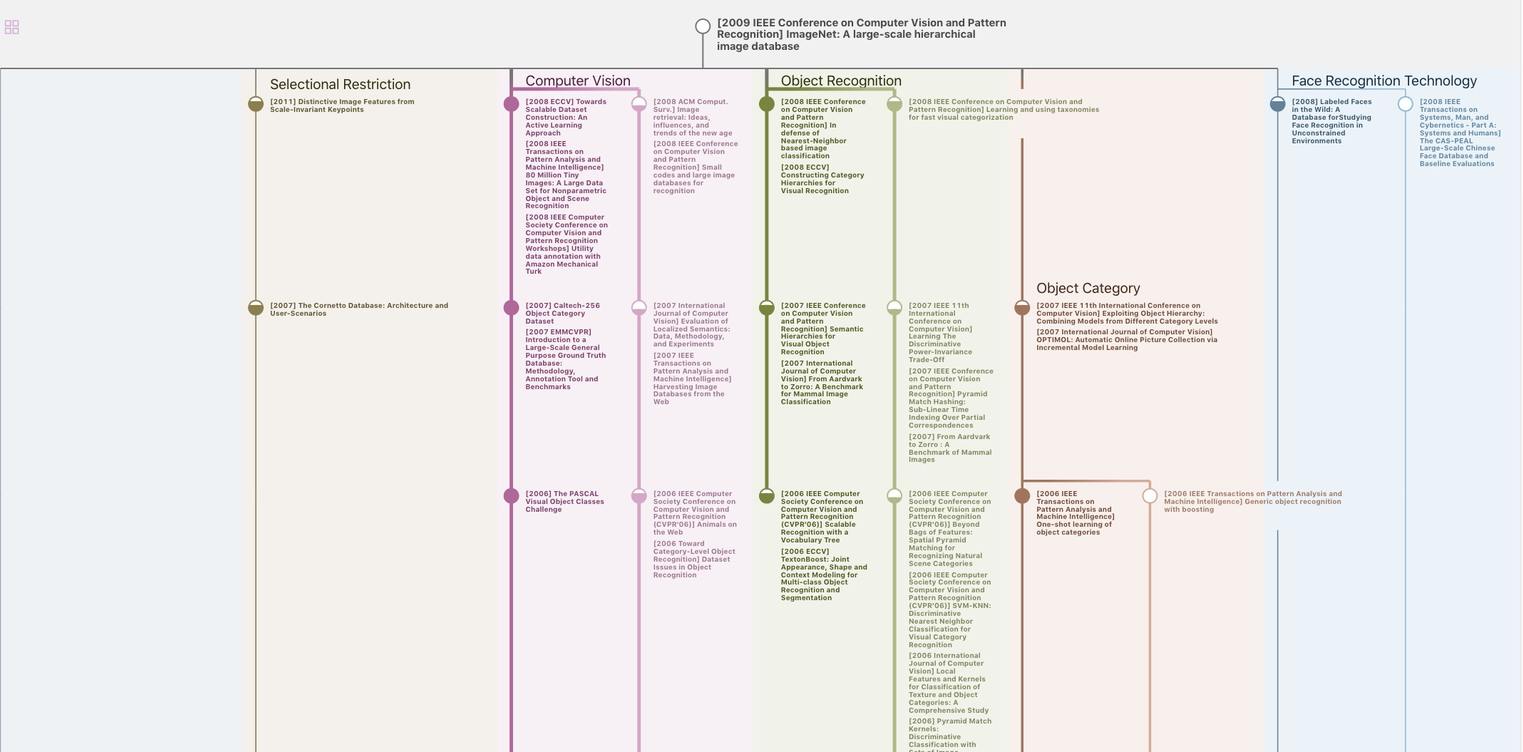
生成溯源树,研究论文发展脉络
Chat Paper
正在生成论文摘要