Evaluation Of Non-Intrusive Load Monitoring Algorithms For Appliance-Level Anomaly Detection
2019 IEEE INTERNATIONAL CONFERENCE ON ACOUSTICS, SPEECH AND SIGNAL PROCESSING (ICASSP)(2019)
摘要
Appliance fault in buildings resulting in abnormal energy consumption is known as an anomaly. Traditionally, anomaly detection is performed either at aggregate, i.e., meter-level, or at appliance level. Meter-level anomaly detection does not identify the anomaly-causing appliance, while appliance-level detection requires submetering each appliance in the building. Non-Intrusive Load Monitoring (NILM) has been proposed as an alternative to submetering to detect when appliances are running as well as estimate the appliance energy consumption. So far, applications have revolved around meaningful energy feedback. In this paper, we assess whether NILM can indeed be used for anomaly detection, as an alternative to submetering. We propose a supervised anomaly detection approach, AEM, and evaluate the effectiveness of NILM for anomaly detection. The proposed approach first learns an appliance's normal operation and then monitors its energy consumption for anomaly detection. We resort to real data, aggregate and submetered data from the two-year long REFIT dataset. We explain why anomaly detection performs worse with NILM data as compared to submetered data, highlighting the need for new, anomaly-aware NILM approaches.
更多查看译文
关键词
NILM, energy disaggregation, anomaly detection, smart metering
AI 理解论文
溯源树
样例
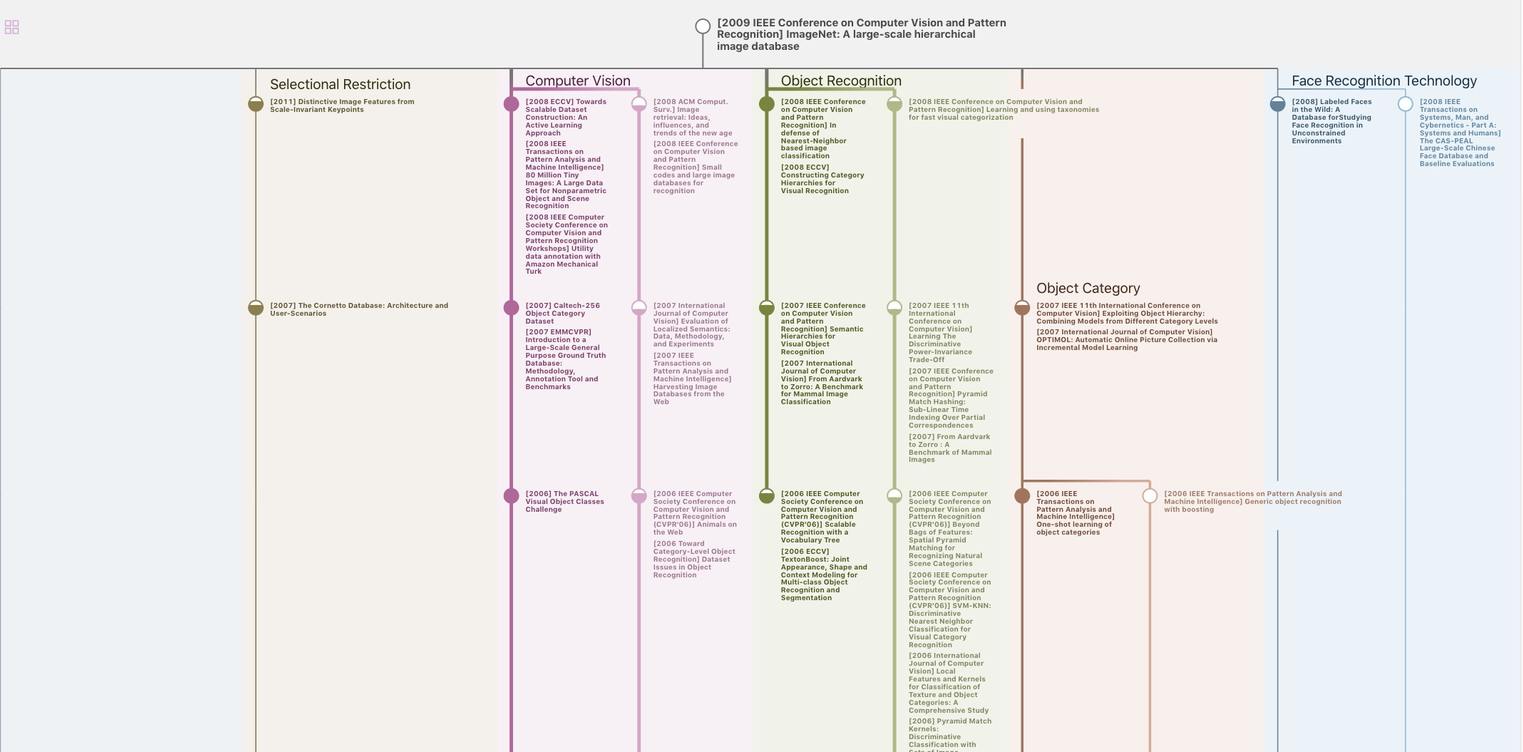
生成溯源树,研究论文发展脉络
Chat Paper
正在生成论文摘要