Consistent Online Optimization: Convex And Submodular
22ND INTERNATIONAL CONFERENCE ON ARTIFICIAL INTELLIGENCE AND STATISTICS, VOL 89(2019)
摘要
Modern online learning algorithms achieve low (sublinear) regret in a variety of diverse settings. These algorithms, however, update their solution at every time step. While these updates are computationally efficient, the very requirement of frequent updates makes the algorithms untenable in some practical applications. In this work, we develop online learning algorithms that update a sublinear number of times. We give a meta-algorithm based on non-homogeneous Poisson Processes that gives a smooth tradeoff between regret and frequency of updates. Empirically, we show that in many cases, we can significantly reduce updates at a minimal increase in regret.
更多查看译文
AI 理解论文
溯源树
样例
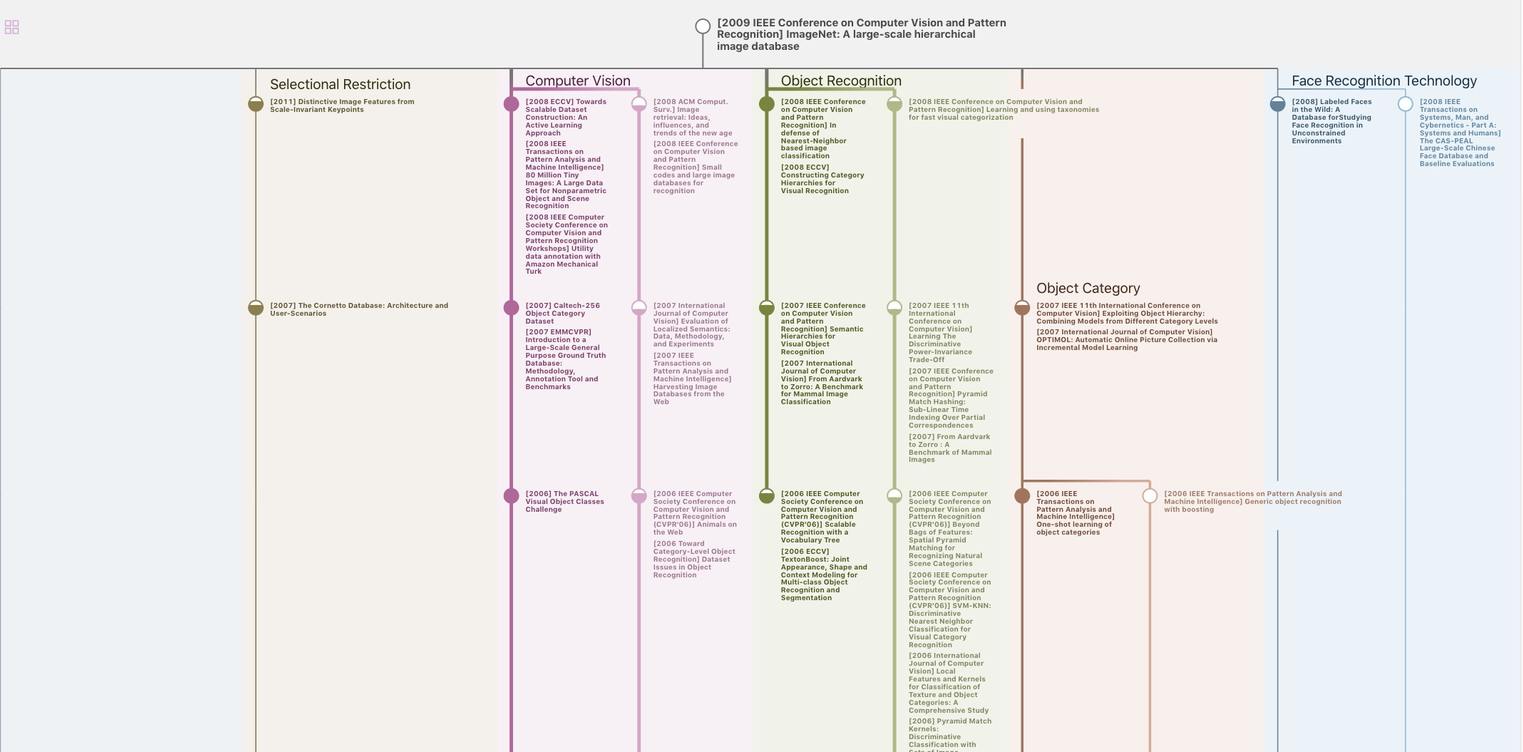
生成溯源树,研究论文发展脉络
Chat Paper
正在生成论文摘要