Unsupervised data to content transformation with histogram-matching cycle-consistent generative adversarial networks
bioRxiv(2019)
摘要
The segmentation of images is a common task in a broad range of research fields. To tackle increasingly complex images, artificial intelligence-based approaches have emerged to overcome the shortcomings of traditional feature detection methods. Owing to the fact that most artificial intelligence research is made publicly accessible and programming the required algorithms is now possible in many popular languages, the use of such approaches is becoming widespread. However, these methods often require data labelled by the researcher to provide a training target for the algorithms to converge to the desired result. This labelling is a limiting factor in many cases and can become prohibitively time consuming. Inspired by the ability of cycle-consistent generative adversarial networks to perform style transfer, we outline a method whereby a computer-generated set of images is used to segment the true images. We benchmark our unsupervised approach against a state-of-the-art supervised cell-counting network on the VGG Cells dataset and show that it is not only competitive but also able to precisely locate individual cells. We demonstrate the power of this method by segmenting bright-field images of cell cultures, images from a live/dead assay of C . elegans , and X-ray computed tomography of metallic nanowire meshes.
更多查看译文
关键词
Computational biology and bioinformatics,Image processing,Imaging techniques,Machine learning,Engineering,general
AI 理解论文
溯源树
样例
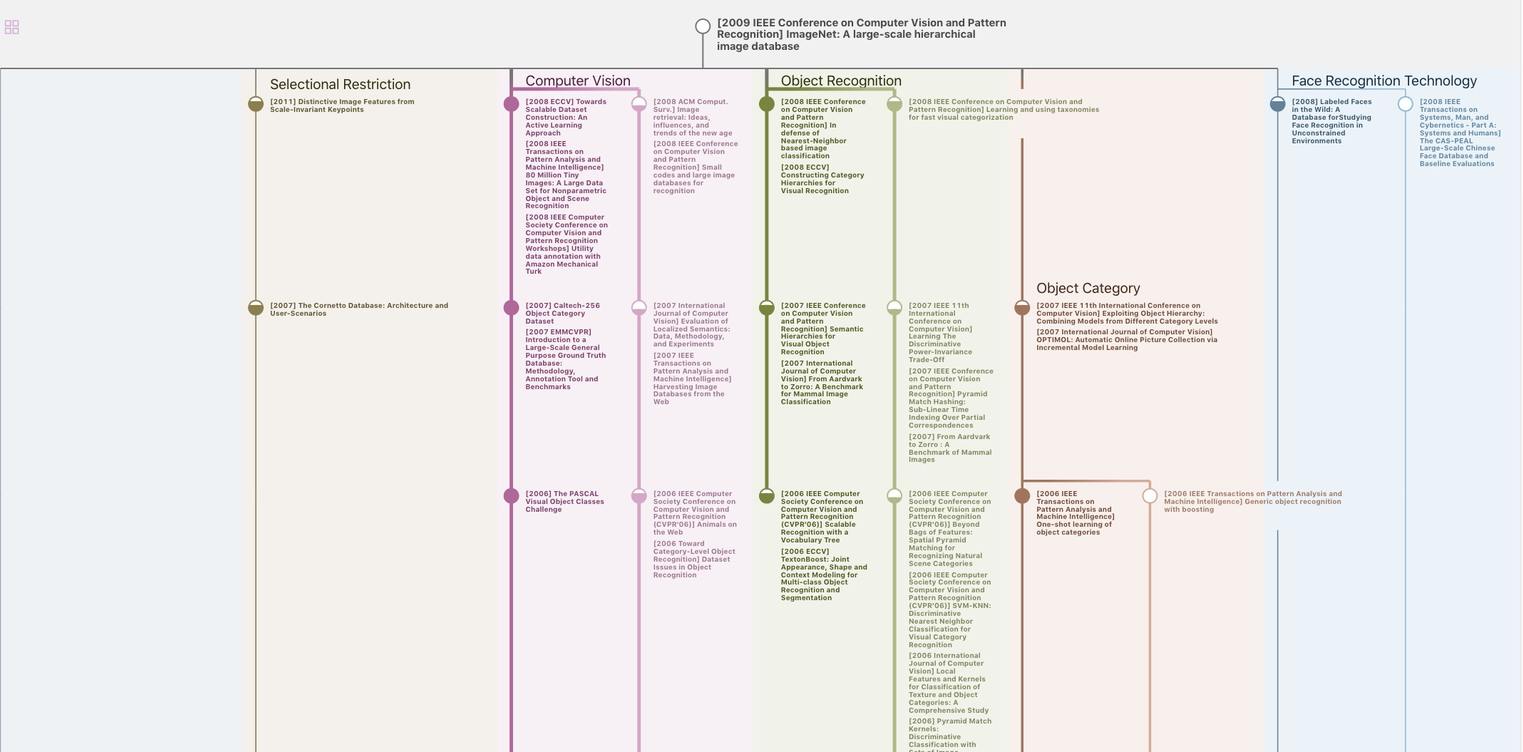
生成溯源树,研究论文发展脉络
Chat Paper
正在生成论文摘要