Deep Learning Models Augment Analyst Decisions for Event Discrimination
GEOPHYSICAL RESEARCH LETTERS(2019)
摘要
Long-term seismic monitoring networks are well positioned to leverage advances in machine learning because of the abundance of labeled training data that curated event catalogs provide. We explore the use of convolutional and recurrent neural networks to accomplish discrimination of explosive and tectonic sources for local distances. Using a 5-year event catalog generated by the University of Utah Seismograph Stations, we train models to produce automated event labels using 90-s event spectrograms from three-component and single-channel sensors. Both network architectures are able to replicate analyst labels above 98%. Most commonly, model error is the result of label error (70% of cases). Accounting for mislabeled events (similar to 1% of the catalog) model accuracy for both models increases to above 99%. Classification accuracy remains above 98% for shallow tectonic events, indicating that spectral characteristics controlled by event depth do not play a dominant role in event discrimination.
更多查看译文
关键词
Utah,event classification,event discrimination,deep learning,convolutional neural network,recurrent neural network
AI 理解论文
溯源树
样例
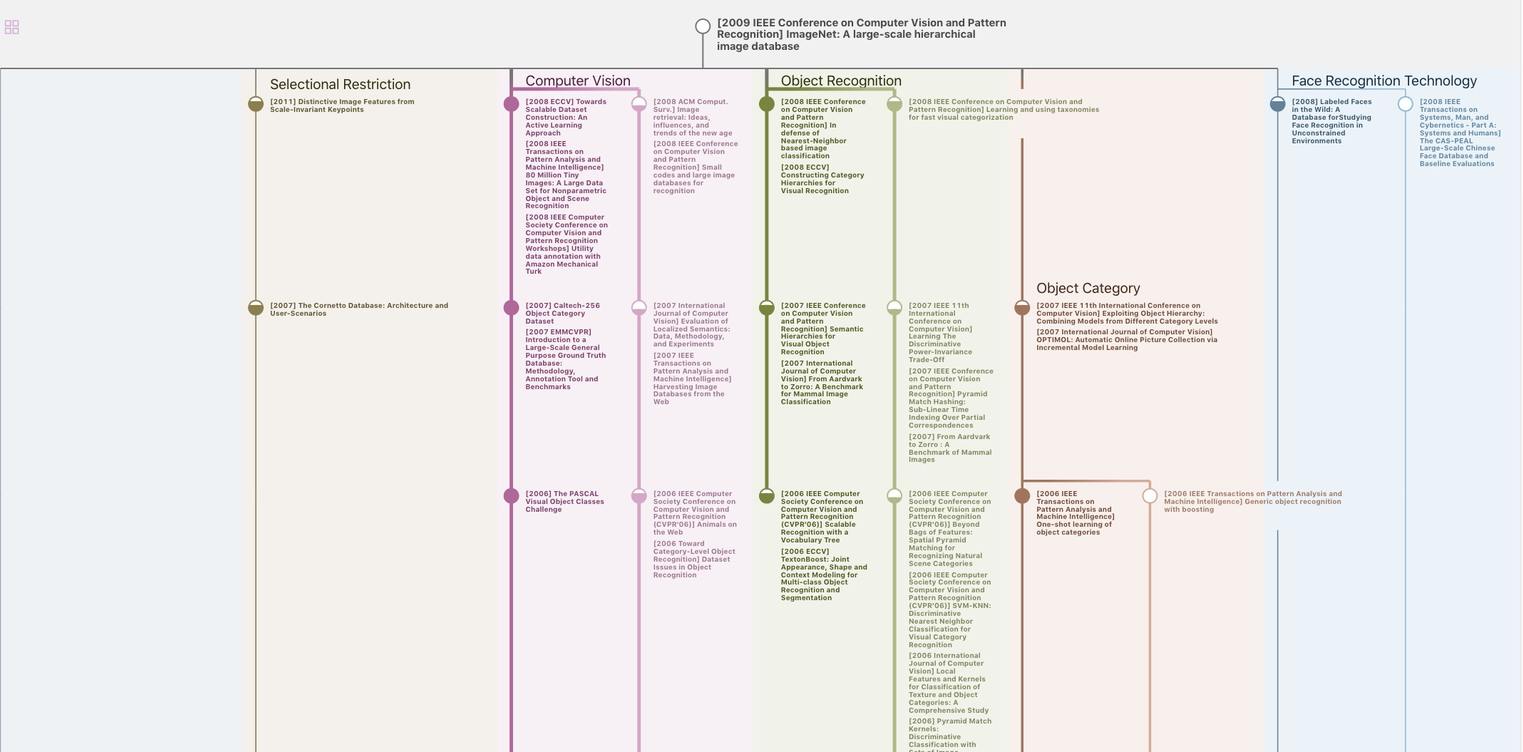
生成溯源树,研究论文发展脉络
Chat Paper
正在生成论文摘要