Stochastic Proximal Gradient Methods for Non-smooth Non-Convex Regularized Problems.
arXiv: Optimization and Control(2019)
摘要
In this paper, we propose and analyze stochastic proximal gradient methods for minimizing a non-convex objective that consists of a smooth non-convex loss and a non-smooth non-convex regularizer. Surprisingly, these methods are as simple as those proposed for handling convex regularizers, and enjoy the same complexities as those for solving convex regularized non-convex problems in terms of finding an approximate stationary point. Our results improve upon the-state-of-art results for solving non-smooth non-convex regularized problems in (Xu et al., 2018a; Metel and Takeda, 2019).
更多查看译文
AI 理解论文
溯源树
样例
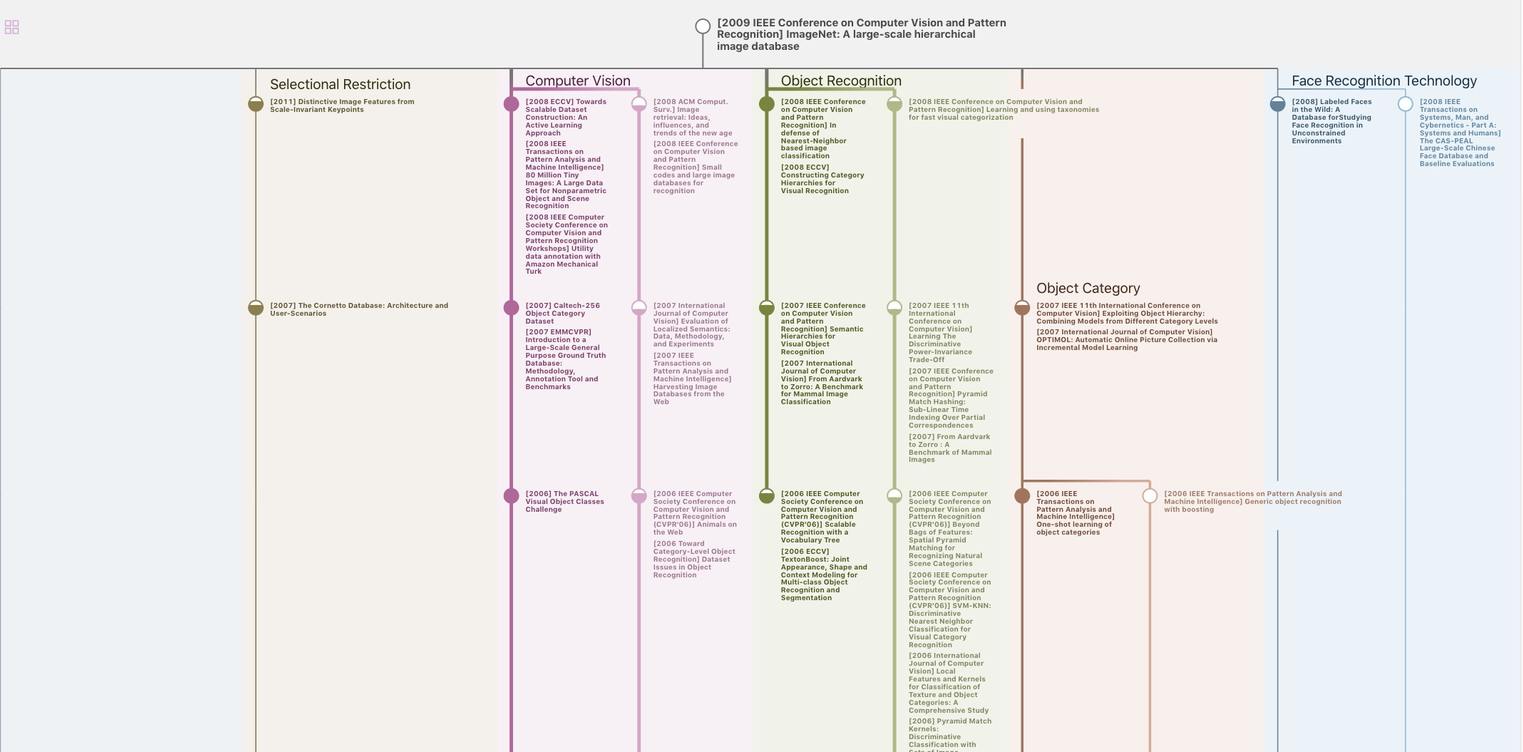
生成溯源树,研究论文发展脉络
Chat Paper
正在生成论文摘要