Depth-Aware Motion Deblurring Using Loopy Belief Propagation
IEEE Transactions on Circuits and Systems for Video Technology(2020)
摘要
Most motion-blurred images captured in real world have spatially-varying point-spread functions. And some are caused by different positions and depth values, which cannot be handled by most state-of-the-art deblurring methods based on deconvolution. To overcome this problem, we propose a depthaware motion blur model that treats a blurred image as an integration of a sequence of clear images. To restore the clear latent image, we extend the Richardson-Lucy method to incorporate our blur model with a given depth image. Empty holes in the depth image, caused by occlusion or device limitations, are fixed by PatchMatch-based depth filling. We regard the depth image as a Markov random field and select candidate labels by using belief propagation to set and smooth depth values for empty areas. Deblurring and depth filling are performed iteratively to refine the results. Our method can also be applied to real-world images with the assistance of motion estimation. The deblurring process is shown to be convergent; moreover, the number of iterations and the level of noise amplification are acceptable. Experimental results show that our method can not only handle depth-variant motion blur but also refine depth images.
更多查看译文
关键词
Transmission line matrix methods,Cameras,Kernel,Image restoration,Three-dimensional displays,Belief propagation,Convolution
AI 理解论文
溯源树
样例
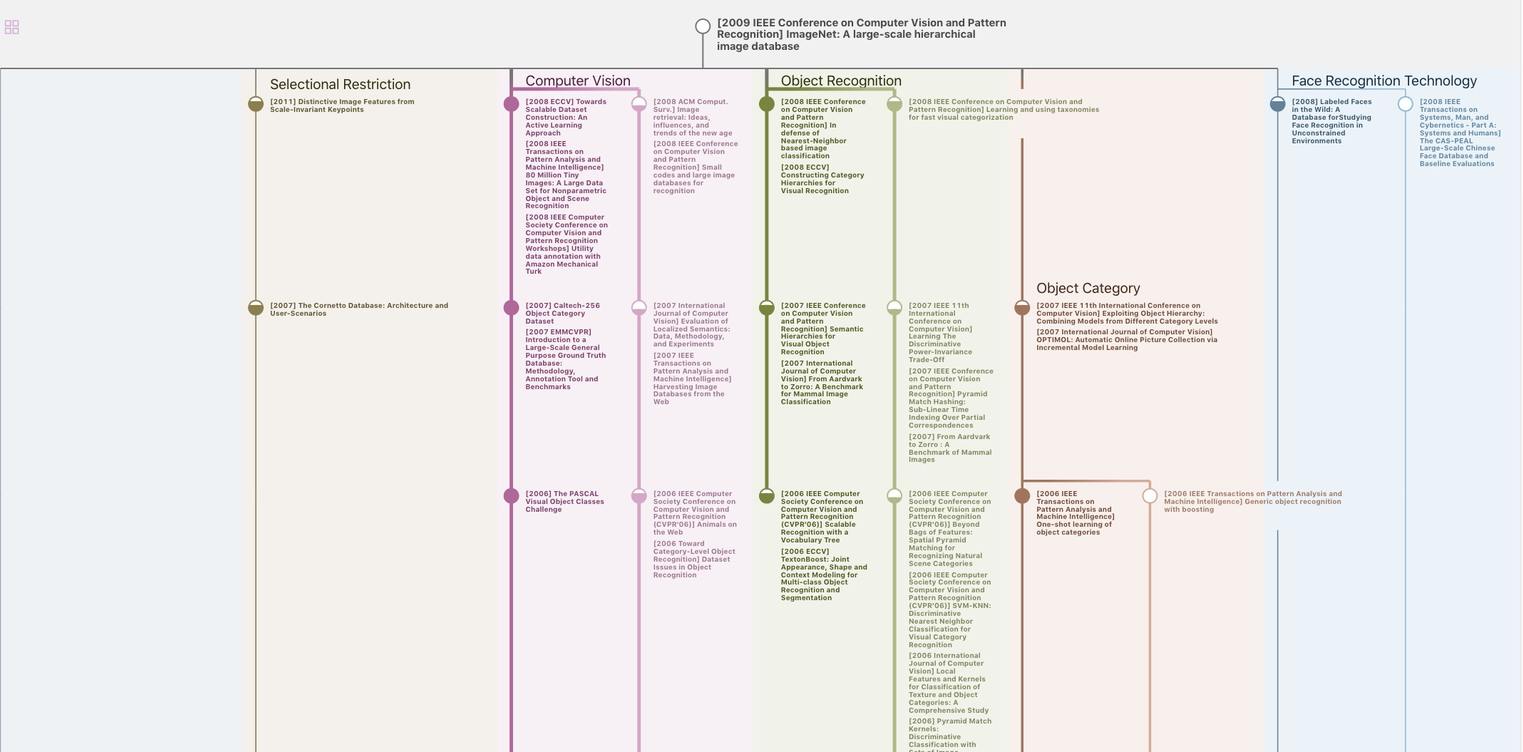
生成溯源树,研究论文发展脉络
Chat Paper
正在生成论文摘要