Synthetic Promoter Design in Escherichia coli based on Generative Adversarial Network
bioRxiv(2019)
摘要
Synthetic promoters are commonly applied elements in circuit design for fine-tuning the protein expression levels. Pro-moter engineering was mostly focused on the random mutation or combination of regulation elements such as transcrip-tion factor binding sites. However, the size of promoter sequence space is still overwhelming and better navigation meth-od is required. On the other hand, the generative adversarial network (GAN) is known for its great ability to reduce the searching space by learning to generate new data on the similar manifold of original data. Here, we applied WGAN-GP model into de novo promoter sequence design to generate entirely new promoter sequences. In total, 83 of model-generated promoter sequences were tested in promoter activity screening by regulating the expression of sfGFP gene in Escherichia coli. As a result, 26 out of 83 newly designed promoters were found functional and successfully expressed with varying activities, with similarity score to natural promoters all less than 0.7. Moreover, 3 of them showed higher promoter strength than the wild type promoters and their highly expression mutants. The much higher successful rate and promoter activity with much lower similarity score in our model-designed novel promoters confirmed the effective-ness of promoter sequence learning. Our work provides insights into an area of navigation of novel functional promoter sequence space automatically, as well as speeding up evolution process of naturally existing promoters, indicating the potential ability for deep generative models to be applied into genetic element designing in the future.
更多查看译文
关键词
synthetic promoters,generative adversarial network,transcriptional fine-tuning,computational design
AI 理解论文
溯源树
样例
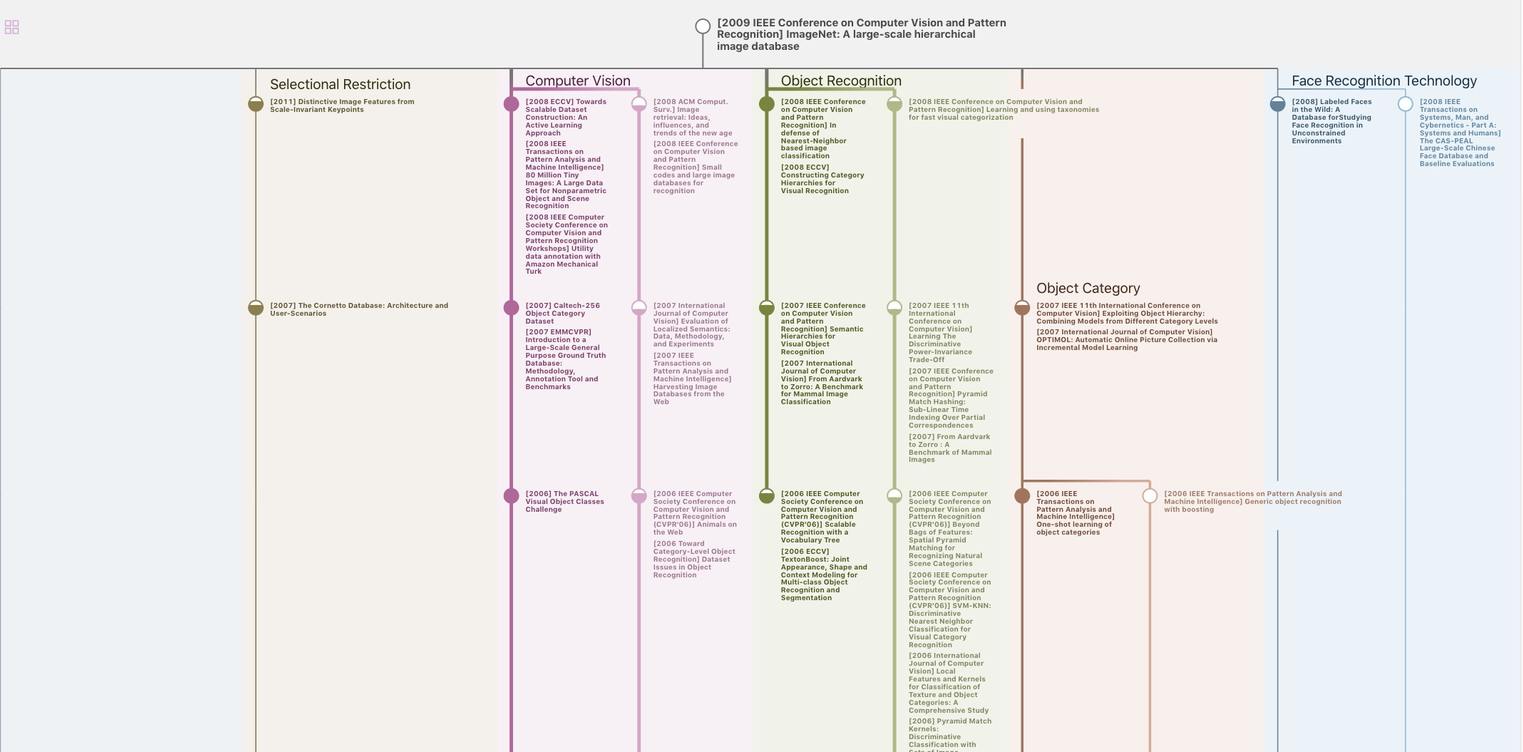
生成溯源树,研究论文发展脉络
Chat Paper
正在生成论文摘要