DeepHoops: Evaluating Micro-Actions in Basketball Using Deep Feature Representations of Spatio-Temporal Data
KDD(2019)
摘要
How much is an on-ball screen worth? How much is a backdoor cut away from the ball worth? Basketball is one of a number of sports which, within the past decade, have seen an explosion in quantitative metrics and methods for evaluating players and teams. However, it is still challenging to evaluate individual off-ball events in terms of how they contribute to the success of a possession. In this study, we develop an end-to-end deep learning architecture DeepHoops to process a unique dataset composed of spatio-temporal tracking data from NBA games in order to generate a running stream of predictions on the expected points to be scored as a possession progresses. We frame the problem as a multi-class sequence classification problem in which our model estimates probabilities of terminal actions taken by players (e.g. take field goal, turnover, foul etc.) at each moment of a possession based on a sequence of ball and player court locations preceding the said moment. Each of these terminal actions is associated with an expected point value, which is used to estimate the expected points to be scored. One of the challenges associated with this problem is the high imbalance in the action classes. To solve this problem, we parameterize a downsampling scheme for the training phase. We demonstrate that DeepHoops is well-calibrated, estimating accurately the probabilities of each terminal action and we further showcase the modelu0027s capability to evaluate individual actions (potentially off-ball) within a possession that are not captured by boxscore statistics.
更多查看译文
关键词
deep learning, lstm, sports analytics
AI 理解论文
溯源树
样例
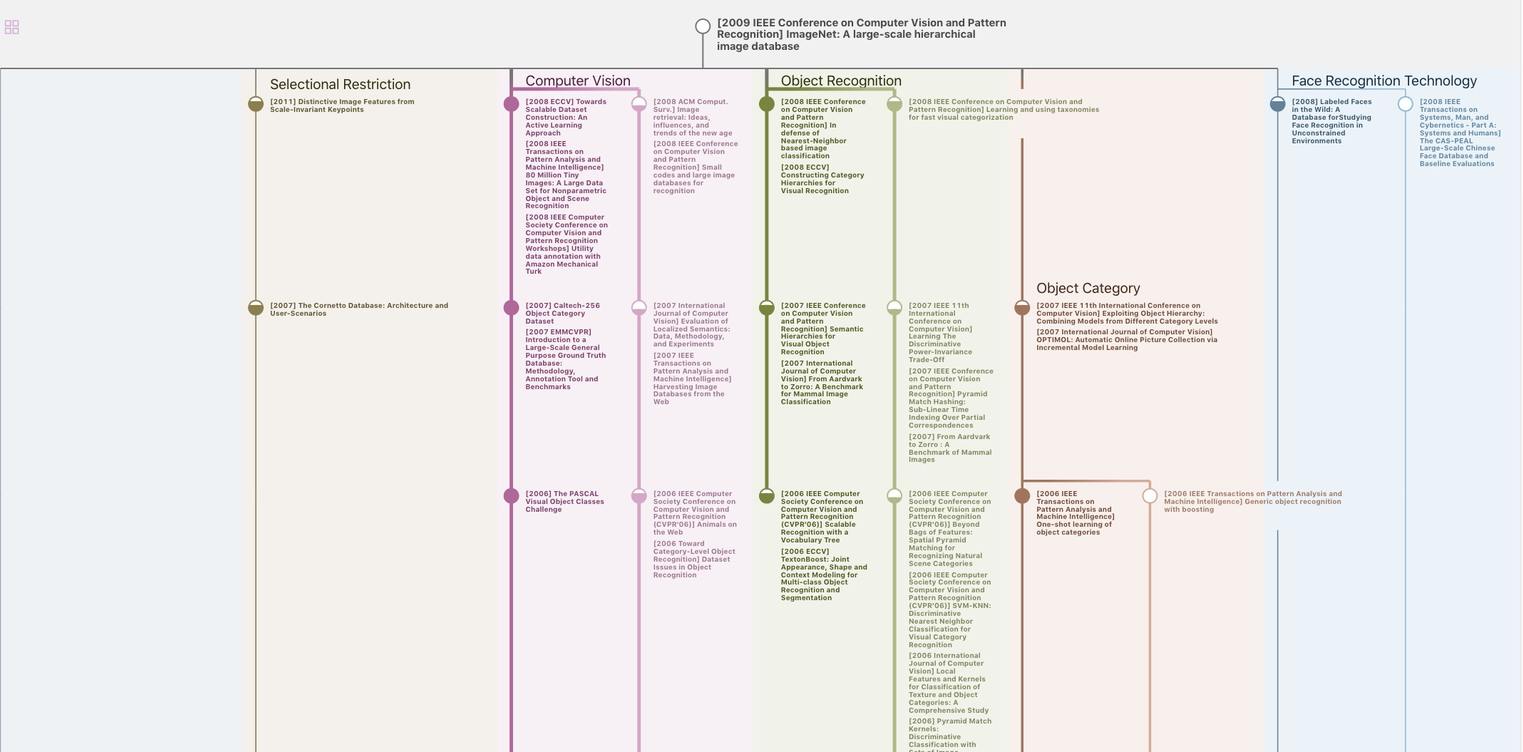
生成溯源树,研究论文发展脉络
Chat Paper
正在生成论文摘要