Time-homogeneous top-K ranking using tensor decompositions
OPTIMIZATION METHODS & SOFTWARE(2020)
摘要
Given N items, all having positive latent strengths, top-K ranking problem aims to identify the K items ( K <= N ) receiving the highest ranks based on partially revealed comparisons among the items. This problem has been widely studied in the case for which comparisons are performed independently. However, identifying the top-K rankings becomes a more intricate task when comparisons per se are performed along some temporal dimension. In this paper, we investigate potential impacts of temporality on sequences of top-K items and propose a time-homogeneous ranking scheme. Our framework relies mainly on tensor decompositions, rank centrality, and an innovative continuous extension of the Bradley-Terry-Luce (BTL) model. The proposed continuous BTL model extends the win/loss nature of the logistic model to a continuous setting, further reflecting preference degrees that may exist among the compared items. Our computations, which pertain to the analysis of S&P500 data from January 2008 to December 2017, confirm that the proposed top-K ranking scheme is an effective approach to optimize cardinality-constrained portfolios which involve large volumes of noisy and incomplete data.
更多查看译文
关键词
Rank aggregation,top-K ranking,Bradley–,Terry–,Luce (BTL) model,rank centrality,non-stationary spatio-temporal data analysis,tensor decomposition,cardinality-constrained portfolio optimization,S&,P500
AI 理解论文
溯源树
样例
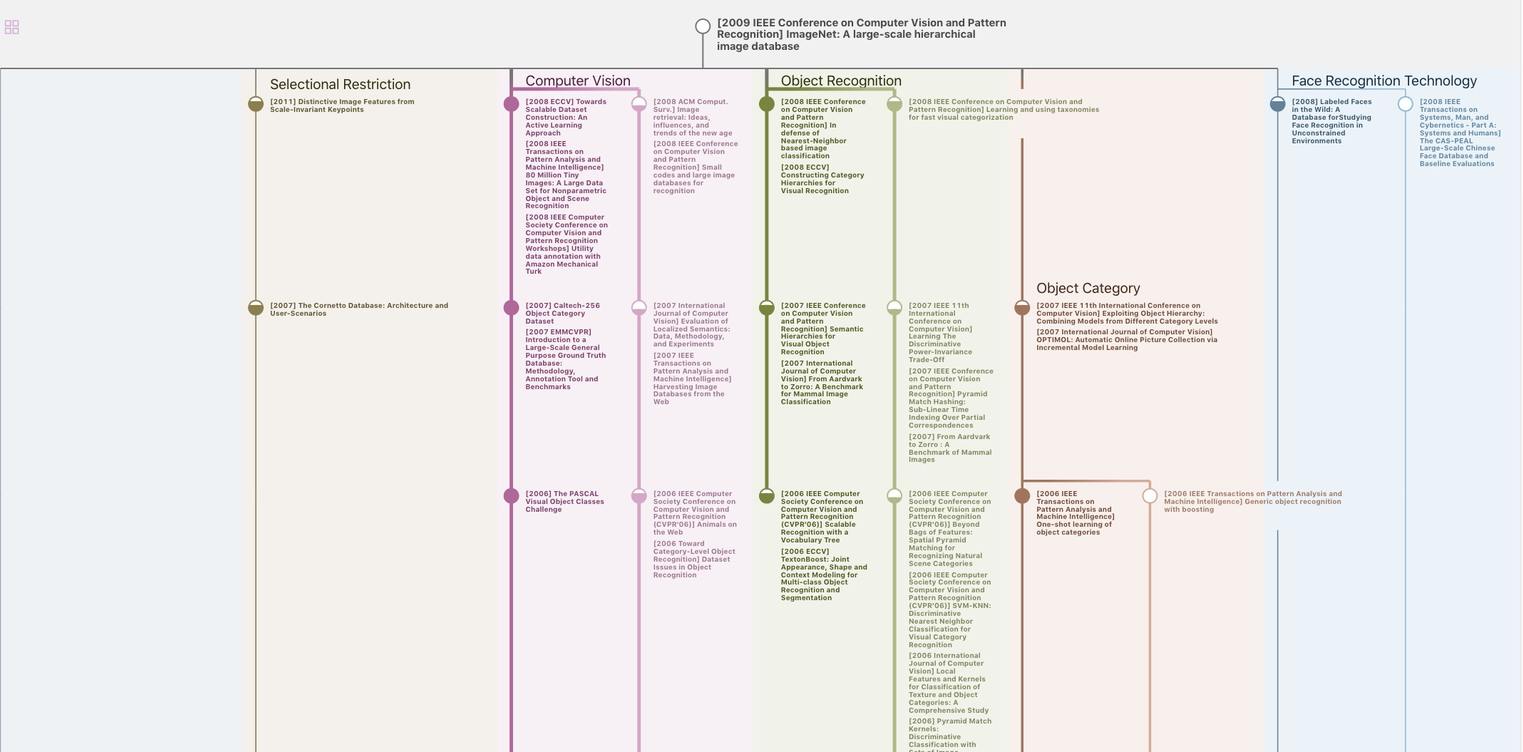
生成溯源树,研究论文发展脉络
Chat Paper
正在生成论文摘要