Estimating Travel Time Distributions by Bayesian Network Inference
IEEE Transactions on Intelligent Transportation Systems(2020)
摘要
Travel time estimation is an important aspect of intelligent transportation systems (ITS). In urban environments, travel times can exhibit much variability due to various stochastic factors. For this reason, we focus on estimating travel time distributions, in contrast to the more commonly studied estimation of mean expected travel times. We present algorithms to infer travel time distributions from Floating Car Data; specifically, from sparse GPS measurements. The framework combines Gaussian copulas and network inference to estimate marginal and joint distributions of travel times. We perform an extensive set of numerical experiments on one month of GPS trajectories. We benchmark the proposed models in terms of Kullback-Leibler (KL) divergence and Hellinger distance for the 50 most common trajectories. Combining Gaussian Copulas and Bayesian Inference of Sparse Networks method achieves 4.9% reduction in KL divergence and 2% reduction in Hellinger distance compared to baseline methods.
更多查看译文
关键词
Global Positioning System,Estimation,Bayes methods,Public transportation,Trajectory,Data models,Computational modeling
AI 理解论文
溯源树
样例
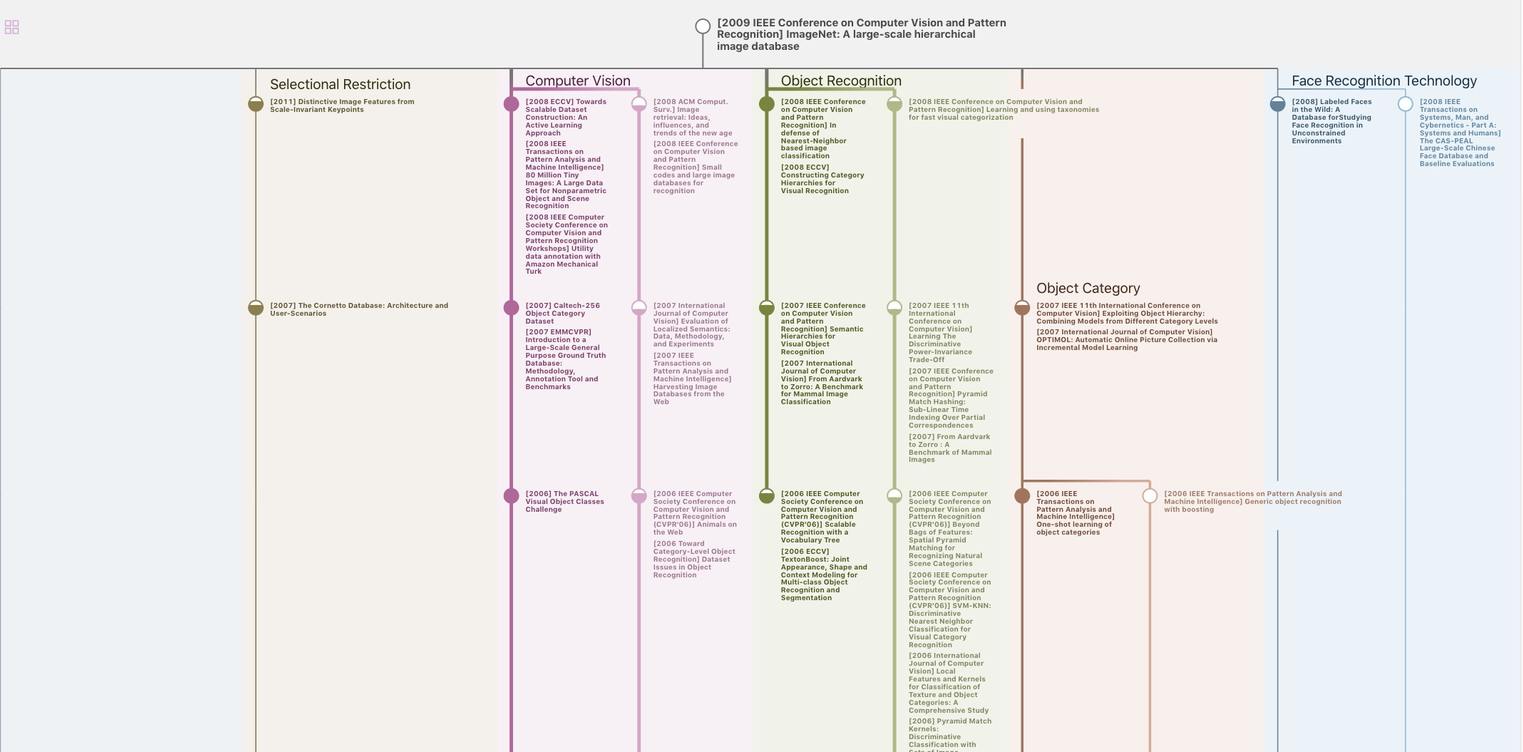
生成溯源树,研究论文发展脉络
Chat Paper
正在生成论文摘要