Can You Trust This Prediction? Auditing Pointwise Reliability After Learning
22ND INTERNATIONAL CONFERENCE ON ARTIFICIAL INTELLIGENCE AND STATISTICS, VOL 89(2019)
摘要
To use machine learning in high stakes applications (e.g. medicine), we need tools for building confidence in the system and evaluating whether it is reliable. Methods to improve model reliability often require new learning algorithms (e.g. using Bayesian inference to obtain uncertainty estimates). An alternative is to audit a model after it is trained. In this paper, we describe resampling uncertainty estimation (RUE), an algorithm to audit the pointwise reliability of predictions. Intuitively, RUE estimates the amount that a prediction would change if the model had been fit on different training data. The algorithm uses the gradient and Hessian of the model's loss function to create an ensemble of predictions. Experimentally, we show that RUE more effectively detects inaccurate predictions than existing tools for auditing reliability subsequent to training. We also show that RUE can create predictive distributions that are competitive with state-of-the-art methods like Monte Carlo dropout, probabilistic back-propagation, and deep ensembles, but does not depend on specific algorithms at train-time like these methods do.
更多查看译文
关键词
auditing pointwise reliability,prediction,trust,learning
AI 理解论文
溯源树
样例
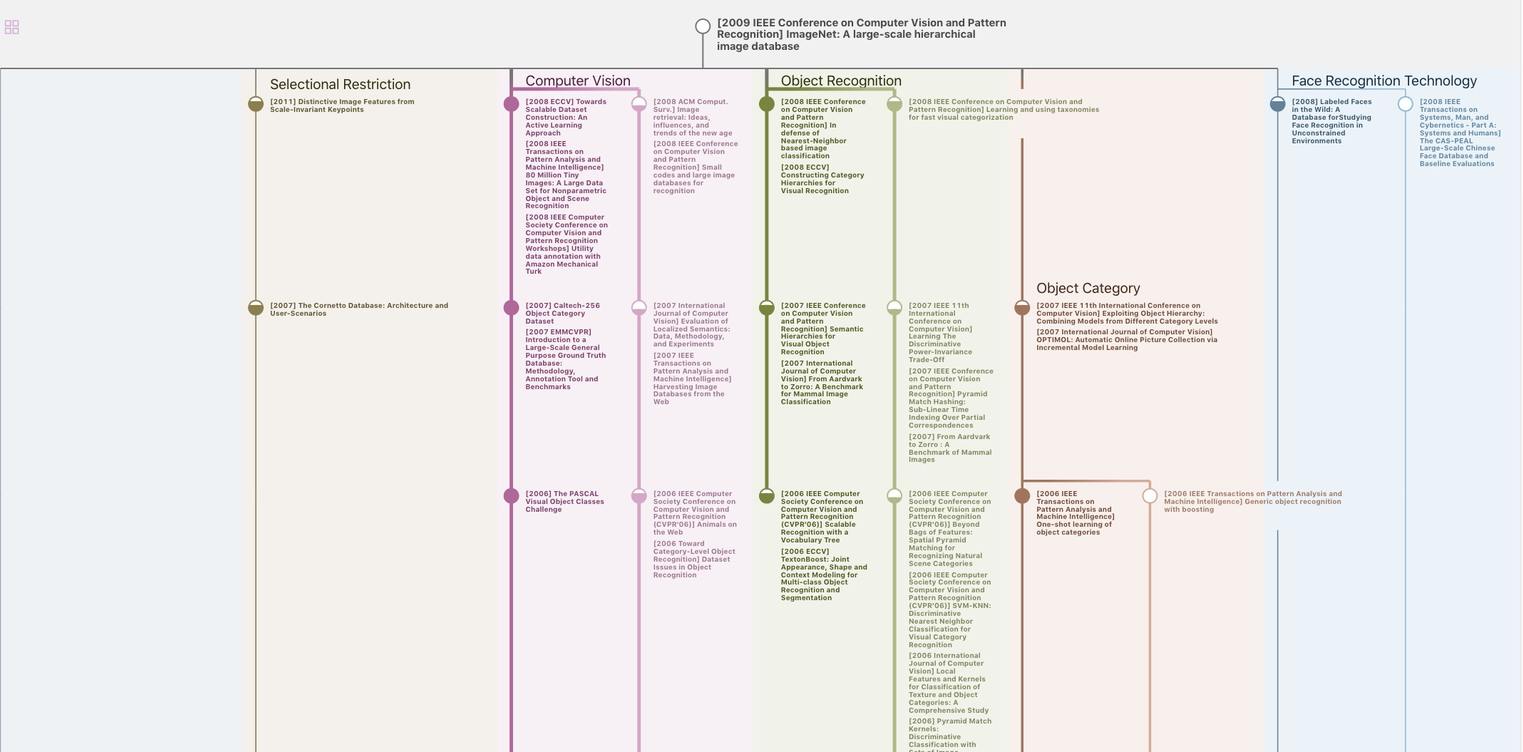
生成溯源树,研究论文发展脉络
Chat Paper
正在生成论文摘要