Hardware-Based Model of Node Clustering Using Q-Learning for Wireless Sensor Networks
TENCON IEEE Region 10 Conference Proceedings(2018)
摘要
Recent researches on the development and deployment of large-scale wireless sensor networks focus on the reduction of power consumption to increase network lifetime. This entails the development of more efficient hardware and the integration of the multiple components into a single platform; possibly, a system-on-a-chip sensor node platform. This paper focuses on the application of Q-learning to node clustering as an effective technique in reducing power consumption. A hardware implementation of CLIQUE, a Q-learning-based clustering algorithm that enhances the operation and resiliency of the network and improves the energy expenditure of the sensor nodes, is presented. The power consumption of the hardware is recorded to be around 6.4 mW. By incorporating this value into OMNeT++, it was demonstrated that CLIQUE improves energy expenditure by 6%-19% and extends network lifetime by 5%-22%, when compared to LEACH, a traditional clustering algorithm.
更多查看译文
关键词
sensor nodes,power consumption,CLIQUE,energy expenditure,network lifetime,traditional clustering algorithm,hardware-based model,node clustering,large-scale wireless sensor networks,multiple components,single platform,system-on-a-chip sensor node platform,Q-learning,OMNeT++
AI 理解论文
溯源树
样例
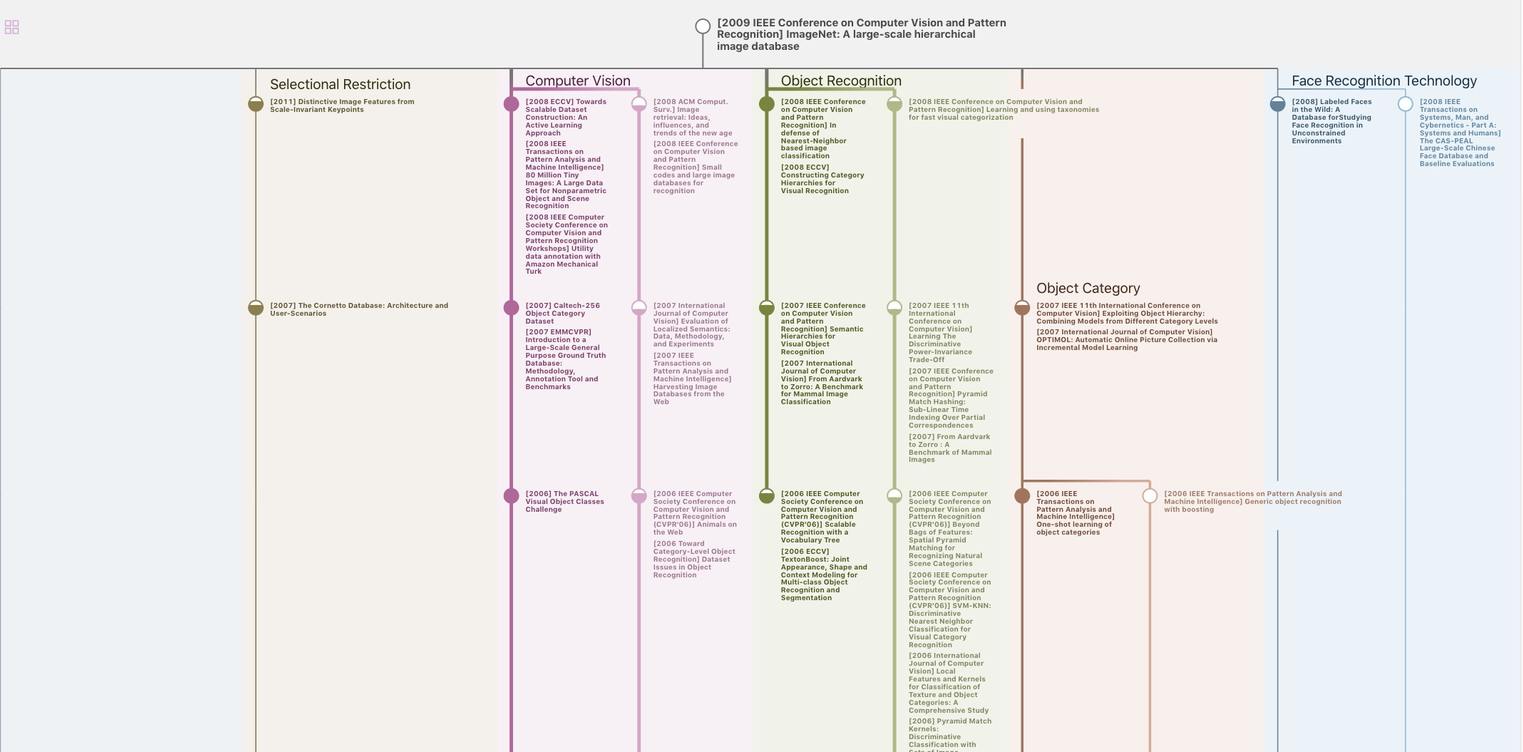
生成溯源树,研究论文发展脉络
Chat Paper
正在生成论文摘要