TDFI: Two-stage Deep Learning Framework for Friendship Inference via Multi-source Information
ieee international conference computer and communications(2019)
摘要
Due to the explosive growth of social network services, friendship inference has been widely adopted by Online Social Service Providers (OSSPs) for friend recommendation. The conventional techniques, however, have limitations in accuracy or scalability to handle such a large yet sparse multi-source data. For example, the OSSPs will be required to manually give the order in which the various information is applied. This unavoidably reduces the applicability of existing friend recommendation systems. To address this issue, we propose a Two-stage Deep learning framework for Friendship Inference (TDFI). This approach can utilize multi-source information simultaneously with low complexity. In particular, we apply an Extended Adjacency Matrix (EAM) to represent the multi-source information. We then adopt an improved Deep AutoEncoder Network (iDAEN) to extract the fused feature vector for each user. The TDFI framework also provides an improved Deep Siamese Network (iDSN) to measure user similarity from iDAEN. Finally, we evaluate the effectiveness and robustness of TDFI on three large-scale real-world datasets. It shows that TDFI can effectively handle the sparse multi-source data while providing better accuracy for friend recommendation.
更多查看译文
关键词
Feature extraction,Complexity theory,Deep learning,Scalability,Computer science,Facebook
AI 理解论文
溯源树
样例
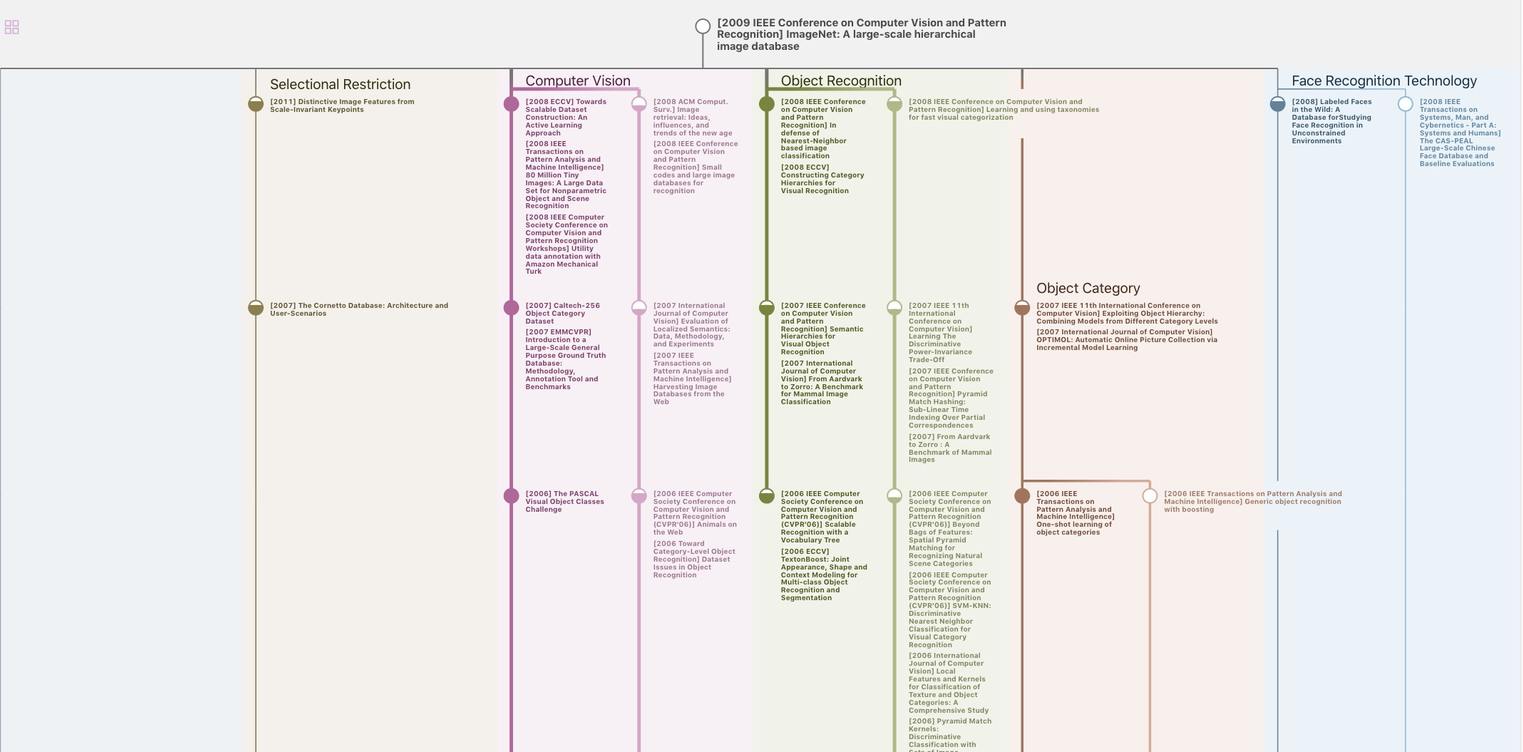
生成溯源树,研究论文发展脉络
Chat Paper
正在生成论文摘要