Learning Invariant Representations With Kernel Warping
22ND INTERNATIONAL CONFERENCE ON ARTIFICIAL INTELLIGENCE AND STATISTICS, VOL 89(2019)
摘要
Invariance is an effective prior that has been extensively used to bias supervised learning with a given representation of data. In order to learn invariant representations, wavelet and scattering based methods "hard code" invariance over the entire sample space, hence restricted to a limited range of transformations. Kernels based on Haar integration also work only on a group of transformations. In this work, we break this limitation by designing a new representation learning algorithm that incorporates invariances beyond transformation. Our approach, which is based on warping the kernel in a data-dependent fashion, is computationally efficient using random features, and leads to a deep kernel through multiple layers. We apply it to convolutional kernel networks and demonstrate its stability.
更多查看译文
AI 理解论文
溯源树
样例
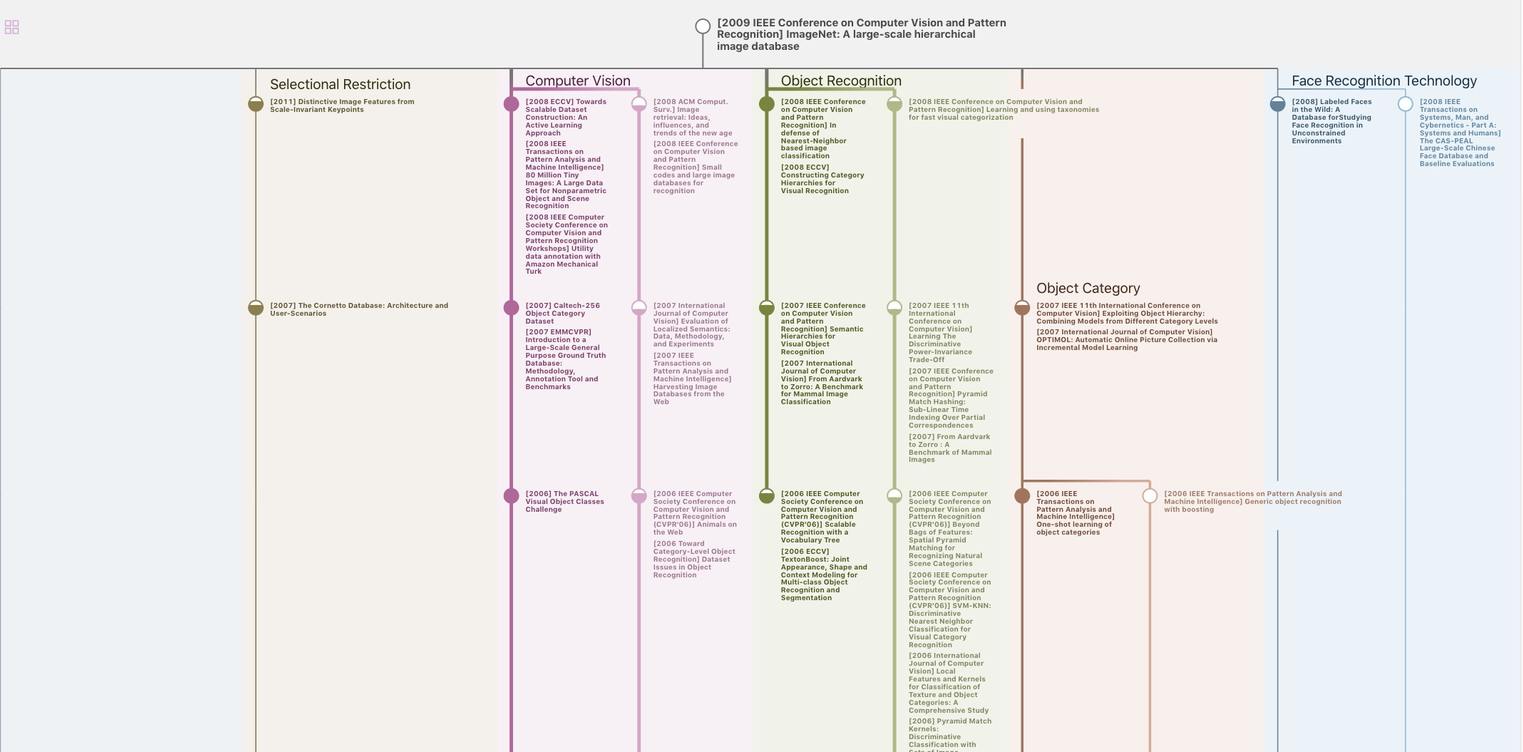
生成溯源树,研究论文发展脉络
Chat Paper
正在生成论文摘要